Analyzing the Interplay of Rainfall, Humidity, and Groundwater in Chennai and Kanchipuram through ARIMA Modeling
2023 International Conference on Data Science, Agents & Artificial Intelligence (ICDSAAI)(2023)
Abstract
To solve pressing environmental issues, a thorough understanding of the transition of the climate system is required. Rising global temperatures are linked to rainfall unpredictability. Groundwater recharge patterns need to be taken into account since rainfall is the main factor causing streamflow, especially flood flow, in India. Rainfall patterns vary seasonally, with the volume of rainfall across different regions and throughout the year exhibiting complex variations. Further research is needed to fully understand these variations, which can lead to different hydrological concerns like floods and dry seasons. Rainfall research during the storm season (July to October) and temperature research throughout the late summer season (February to May) are crucial for economic development, disaster management, and hydrological planning in India. Understanding major temperature and rainfall changes at a limited geographical scale is essential. The different patterns of these variables can be examined using the seasonality index of temperature and rainfall. Kanchipuram region is divided into two divisions: Kanchipuram division and Chennai division. These divisions encompass a total of six taluks: Kanchipuram, Uthiramerur, Sriperumbudur, Kundrathur, and Chengalpet. As of July 2023, there are approximately 189 revenue villages in the Kanchipuram region. This research aims to investigate annual trends in rainfall patterns, Groundwater level, and Humidity using the Autoregressive Integrated Moving Average (ARIMA) model. We have additionally concentrated on region-level geography and waterway re-energize examples to figure out the relationship grid and connection boundaries. Our perceptions show an immediate reliance of stream water stream on precipitation and groundwater re-energize, with 67% and 78% accuracy, respectively. The moistness dataset displays promising expectation potential, with 35% exactness in light of the 5-year timetable dataset. We have likewise confirmed information overt repetitiveness and information irregularity to accomplish a close to 99% information proficient model.Keywords–- Machine learning, Artificial Neural network(ANN) models, water quality Index, comparative analysis, Irrigation purposes.)
MoreTranslated text
Key words
Autoregressive integrated moving average (ARIMA) model,Climate Trends,Power BI Software,Data Analytics
AI Read Science
Must-Reading Tree
Example
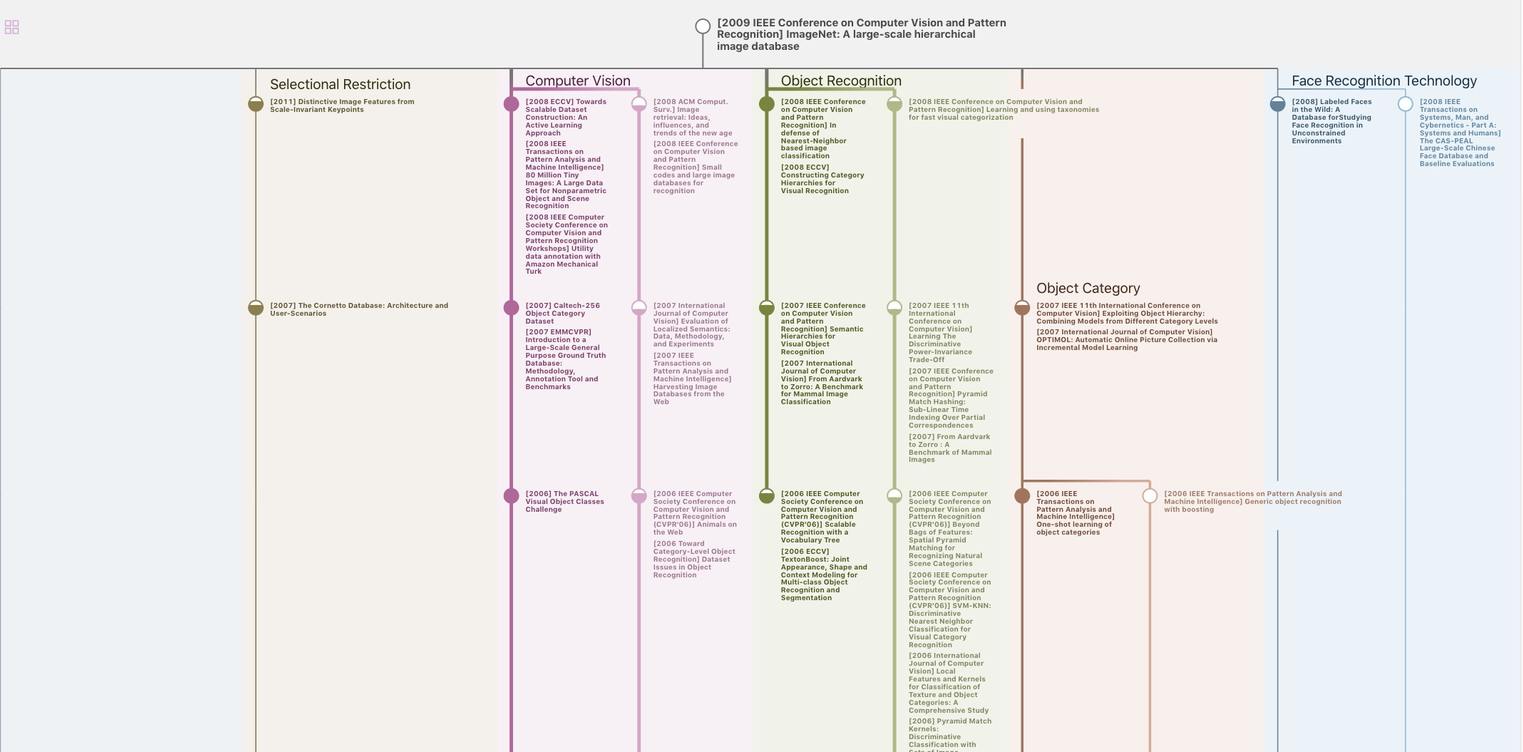
Generate MRT to find the research sequence of this paper
Chat Paper
Summary is being generated by the instructions you defined