FedTM: Memory and Communication Efficient Federated Learning with Tsetlin Machine
2023 INTERNATIONAL SYMPOSIUM ON THE TSETLIN MACHINE, ISTM(2023)
摘要
Federated Learning has been an exciting development in machine learning, promising collaborative learning without compromising privacy. However, the resource-intensive nature of Deep Neural Networks (DNN) has made it difficult to implement FL on edge devices. In a bold step towards addressing this challenge, we present FedTM, the first FL framework to utilize Tsetlin Machine, a low-complexity machine learning alternative. We proposed a two-step aggregation scheme for combining local parameters at the server which addressed challenges such as data heterogeneity, varying participating client ratio and bit-based aggregation. Compared to conventional Federated Averaging (FedAvg) with Convolutional Neural Networks (CNN), on average, FedTM provides a substantial reduction in communication costs by 30.5x and 36.6x reduction in storage memory footprint. Our results demonstrate that FedTM outperforms BiFL-BiML (SOTA) in every FL setting while providing 1.37 - 7.6x reduction in communication costs and 2.93 - 7.2x reduction in run-time memory on our evaluated datasets, making it a promising solution for edge devices.
更多查看译文
关键词
Federated Learning,Tsetlin Machine,Communication-Efficient
AI 理解论文
溯源树
样例
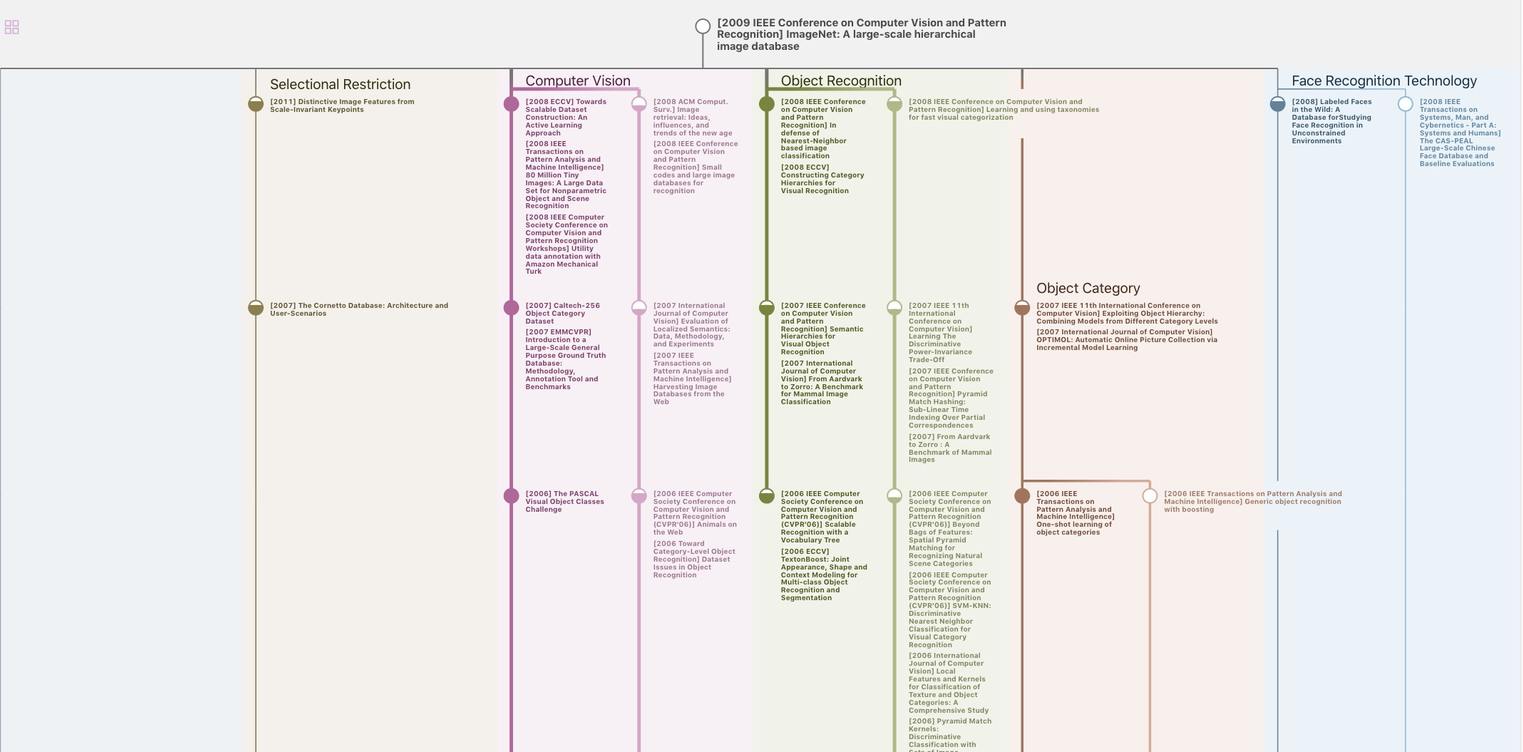
生成溯源树,研究论文发展脉络
Chat Paper
正在生成论文摘要