Instance-level Adversarial Source-free Domain Adaptive Person Re-identification.
ACM Trans. Multim. Comput. Commun. Appl.(2024)
摘要
Domain adaption (DA) for person re-identification (ReID) has attained considerable progress by transferring knowledge from a source domain with labels to a target domain without labels. Nonetheless, most of existing methods require access to source data, which raises privacy concerns. Source-free DA has recently emerged as a response to these privacy challenges, yet its direct application to open-set pedestrian re-identification tasks is hindered by the reliance on a shared category space in existing methods. Current source-free DA approaches for person ReID still encounter several obstacles, particularly the divergence-agnostic problem and the notable domain divergence due to the absent source data. In this paper, we introduce an Instance-level Adversarial Mutual Teaching (IAMT) framework, which utilizes adversarial views to tackle the challenges mentioned above. Technically, we first elaborately develop a variance-based division (VBD) module to segregate the target data into instance-level subsets based on their similarity and dissimilarity to the source using source-trained model, implicitly tackling the divergence-agnostic problem. To mitigate domain divergence, we additionally introduce a dynamic adversarial alignment (DAA) strategy, aiming to enhance the consistence of feature distribution across domains by employing adversarial instances from the target data to confuse the discriminators. Experiments reveal the superiority of the IAMT over state-of-the-art methods for DA person ReID tasks, while preserving the privacy of the source data.
更多查看译文
关键词
Person re-identification,Source-free domain adaptation,Adversarial attacks,Mutual teaching
AI 理解论文
溯源树
样例
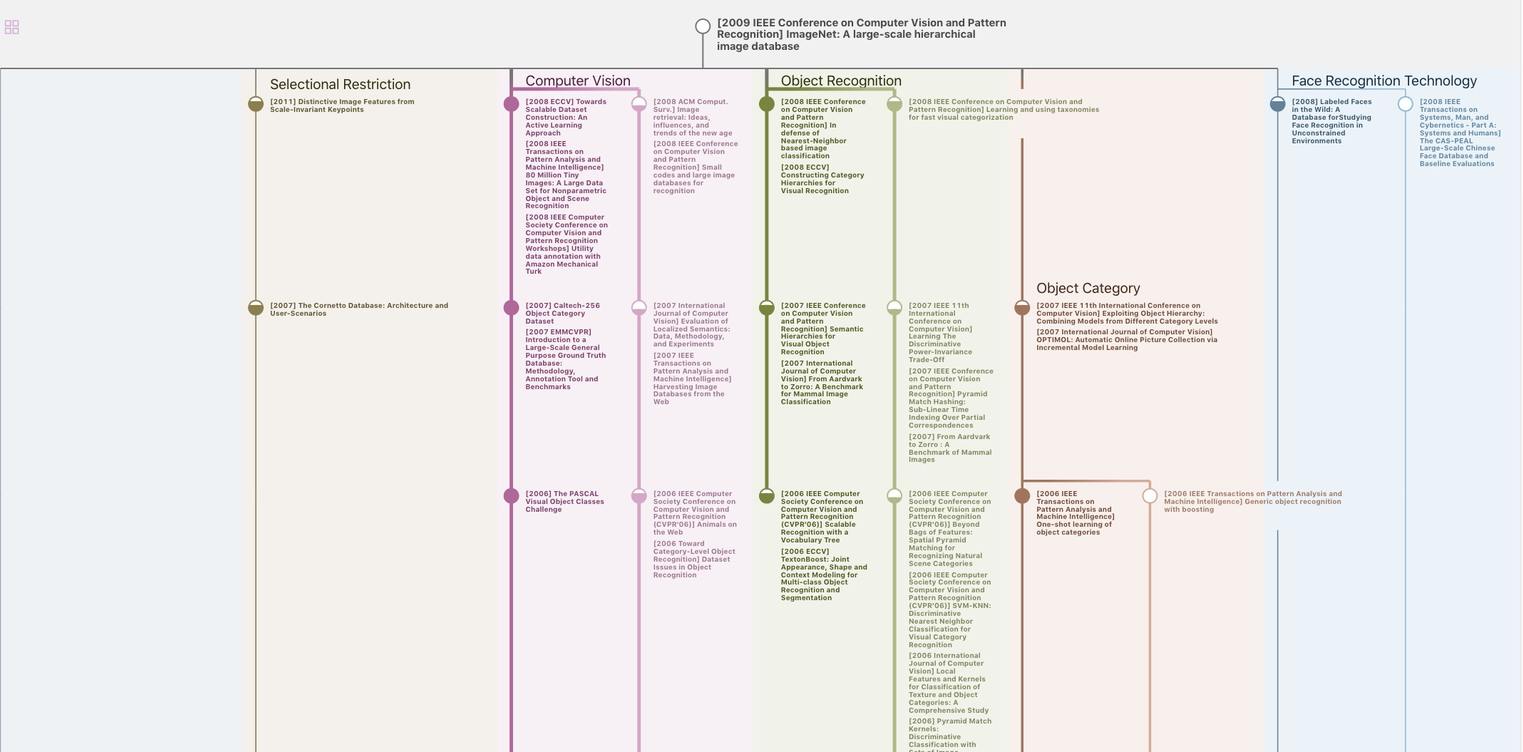
生成溯源树,研究论文发展脉络
Chat Paper
正在生成论文摘要