Predictive Modeling of Donor Mobilization for Hematopoietic Cell Transplantation
Transplantation and Cellular Therapy(2024)
摘要
Introduction
Efficacy of mobilization of peripheral blood stem cells (PBSC) is critical in providing patients with optimal products. We wanted to determine if pre-mobilization donor characteristics and lab values routinely obtained prior to mobilization could predict CD34+ yield. Our aim was to develop predictive models to understand donor traits associated with mobilization response, thereby facilitating optimal donor selection and apheresis procedure planning.
Methods
We analyzed all initial NMDP-facilitated U.S. domestic apheresis PBSC collections from donors aged ≤ 45 years between January 2005 and September 2019, comprising 23,393 records with detailed clinical and HLA-related features available. We defined super mobilizers (SM) as donors with a day 5 pre-apheresis peripheral blood CD34+ count/μL ≥125.020 (at or above 75th percentile), and poor mobilizers (PM) as those with a day 5 CD34+ count/μL ≤ 36.820 (at or below the 10th percentile), indicating likelihood of mobilization success and failure at day 5 (or 6), respectively. Machine learning models were built using feature subsets selected through the recursive feature elimination technique, predicting SM or PM. Feature importance was assessed through SHapley Additive exPlanations (SHAP). Multiple logistic regression evaluated the effect of a-priori clinically selected donor factors associated with SM or PM. Final models were validated using internal and external datasets. Model performance was evaluated via calibration and discrimination.
Results
Machine learning models achieved accuracies ranging from 72-90% for SM/PM. Figure 1 depicts the feature importance plot, ranking features based on mean absolute SHAP values. Higher SHAP values indicate SM or PM likelihoods in Fig. 1(a) and (b), respectively. A high BMI suggests high likelihood of SM status, while a low BMI is associated with PM. Male donors are more likely to be SM, while female donors tend to be PM. Logistic regression showed many of the same predictive variables were associated with CD34+ yield, (Figure 2) which was supported by internal and external validation with the C-statistic ranging from 0.67-0.74 and the Brier score ranging from 0.16-0.17 on the validation datasets.
Conclusion
Our models offer insights into the likelihood of predicting successful or poor donor mobilization using routinely obtained data. These models may support future approaches to optimize donor selection and aid collection for transplantation.
更多查看译文
AI 理解论文
溯源树
样例
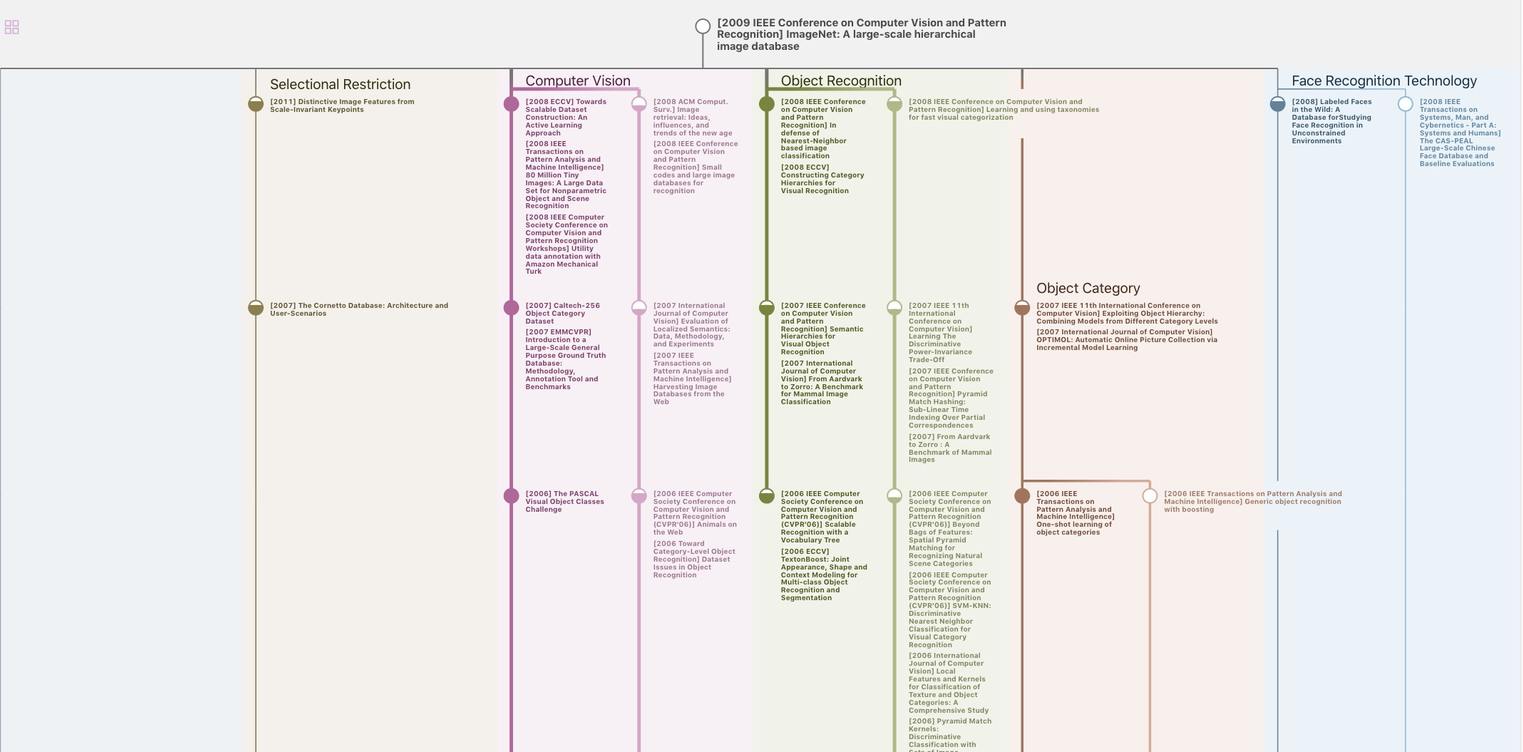
生成溯源树,研究论文发展脉络
Chat Paper
正在生成论文摘要