Transformer-based structural seismic response prediction
STRUCTURES(2024)
摘要
Seismic response prediction is a crucial aspect of evaluating the performance of civil structures. Accurate and efficient response prediction is of significance owing to its application ranging from structural design to structural performance evaluation. Nonlinear time history analysis offers precise and deterministic predictions of seismic response. However, its practical application is limited by the significant computational costs and low modeling efficiency associated with this method. Therefore, a novel Deep Learning (DL) based deterministic structural seismic response prediction method is proposed as an alternative to nonlinear time history analysis. This framework adopts the Encoder-Decoder architecture of Transformer, with the Encoder encoding the seismic wave and the Decoder, coupled with a Long Short-Term Memory (LSTM) neural network, decoding seismic wave encoding features and obtaining preliminary seismic response. Additionally, Moving Average (MA) operation is embedded into the proposed framework, aiming to adjust the preliminary prediction and acquire the final seismic response. Experimental results on four synthetic datasets and one real dataset show that the proposed TLM method has excellent prediction accuracy for both linear and nonlinear systems as well as for linear-elastic and elastoplastic response prediction of structures. Meanwhile, the TLM method is more computationally efficient than traditional numerical methods for solving relatively refined models.
更多查看译文
关键词
Deterministic structural response prediction,Deep learning,Transformer,LSTM,Moving average
AI 理解论文
溯源树
样例
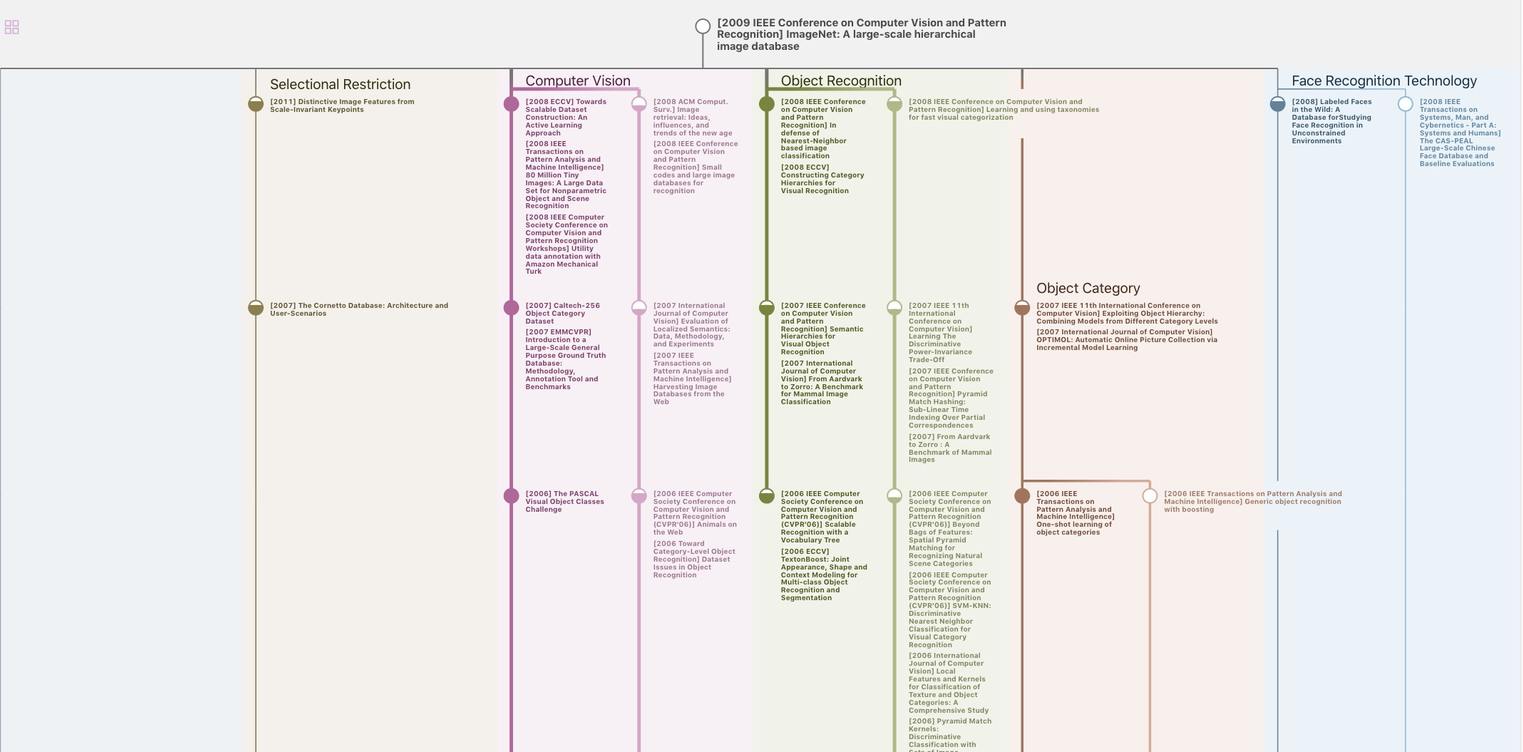
生成溯源树,研究论文发展脉络
Chat Paper
正在生成论文摘要