Scalable efficient reproducible multi-task learning via data splitting
STATISTICS & PROBABILITY LETTERS(2024)
摘要
In contemporary application, multi -task learning's significance has surged. This paper presents a scalable, efficient variable selection method for reproducible multi -task learning through data splitting, offering theoretically guaranteed FDR control and exhibiting asymptotic power of one under mild assumptions.
更多查看译文
关键词
Multi-task learning,False discovery rate,High dimensionality,Power
AI 理解论文
溯源树
样例
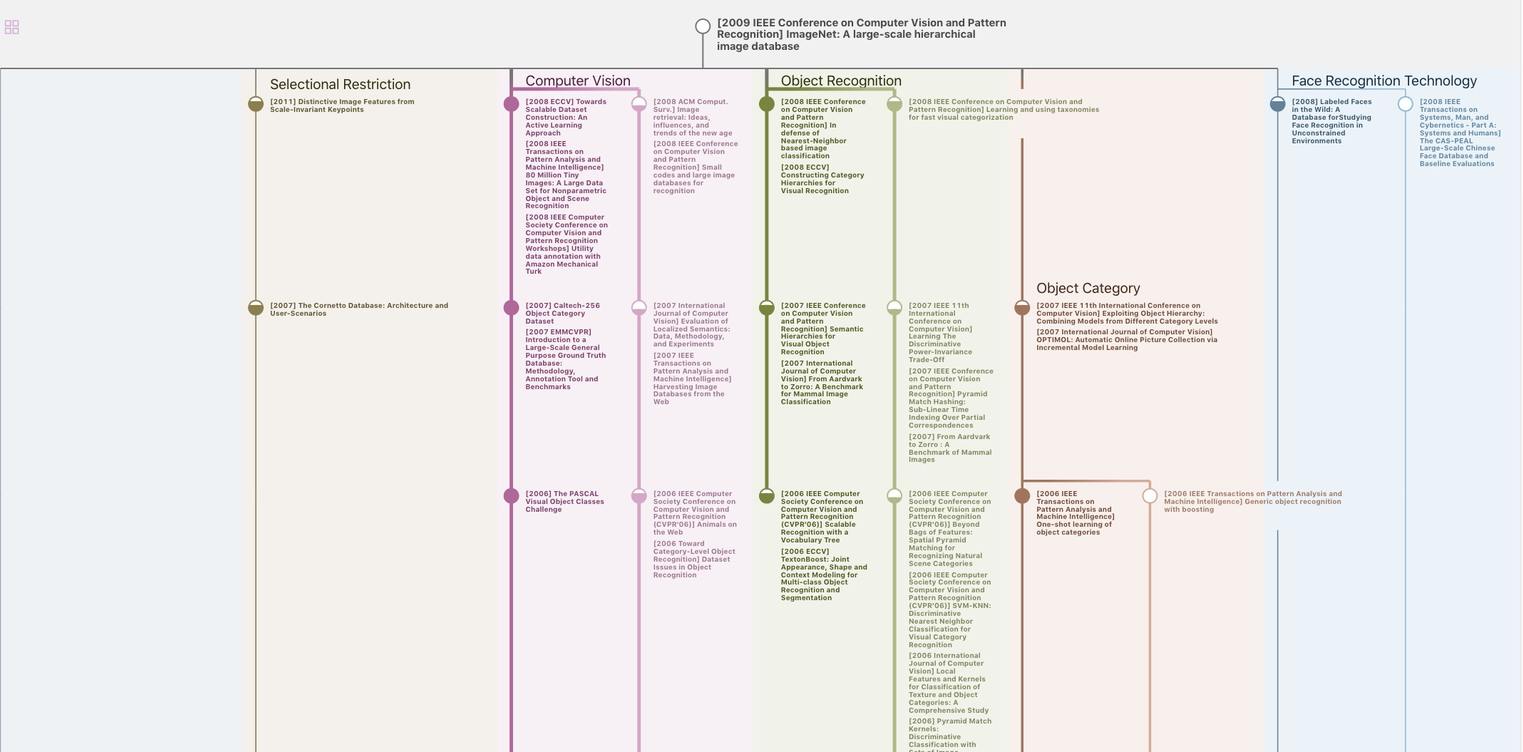
生成溯源树,研究论文发展脉络
Chat Paper
正在生成论文摘要