Meta-learning with elastic prototypical network for fault transfer diagnosis of bearings under unstable speeds
RELIABILITY ENGINEERING & SYSTEM SAFETY(2024)
摘要
Existing studies on meta-learning based few-shot fault diagnosis largely focus on constant speed scenarios, neglecting the consideration of more realistic scenarios involving unstable speeds. In addition, effective measures are highly required to address the complexity of signals in the feature encoding stage and the classification of encoded points. To address these issues, this study proposes a meta-learning approach utilizing an elastic prototypical network (EProtoNet) for few-shot fault transfer diagnosis in scenarios characterized by unstable speeds that better approximate real-world conditions. Firstly, a reinforced feature encoder is devised, incorporating a squeeze and excitation attention mechanism, which enables a deeper exploration of effective features within complex signals encountered during unstable speeds. Secondly, an elastic measurer is introduced, featuring an elastic factor that offers more flexible discrimination between different fault classes. The proposed method is applied to analyze rolling bearing vibration signals with speed fluctuations. Comparative evaluation against existing methods demonstrates that the proposed approach exhibits higher accuracy, reduced result volatility, faster testing speed across various scenarios, and greater suitability for cross-domain few-shot fault diagnosis in the context of unstable speeds.
更多查看译文
关键词
Elastic prototypical network,Meta-learning,Fault transfer diagnosis,Unstable speeds,Reinforced feature encoder,Elastic measurer
AI 理解论文
溯源树
样例
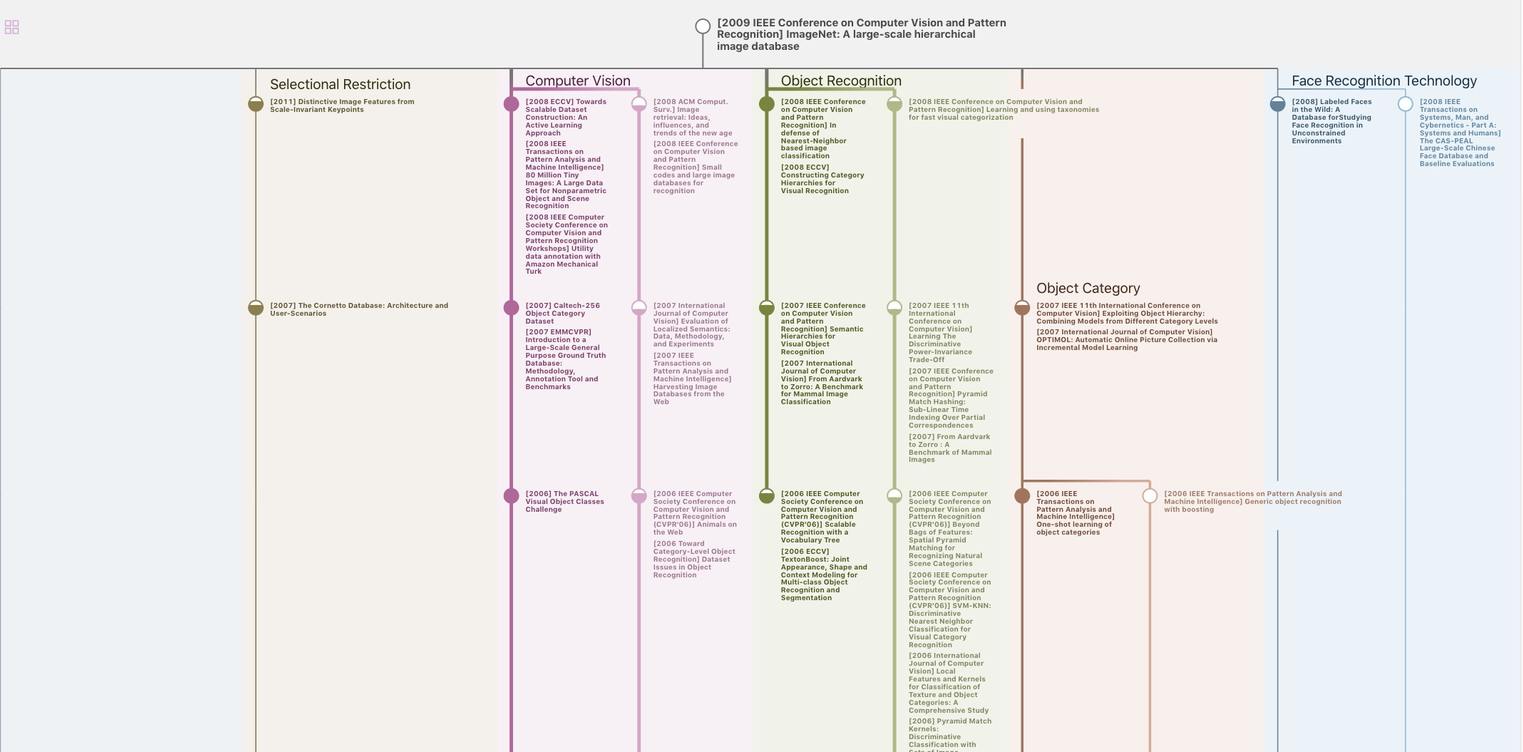
生成溯源树,研究论文发展脉络
Chat Paper
正在生成论文摘要