A novel framework based on two-stage multi-view feature optimization and improved support vector data description for aeroengine bearing early fault detection
RELIABILITY ENGINEERING & SYSTEM SAFETY(2024)
Abstract
Early fault detection is crucial to avoid catastrophic flight accidents caused by unplanned downtime of equipment. Aimed at the shortcomings of feature selection and early fault detection, this paper proposes a method combining two -stage multi -view feature optimization and improved support vector data description for aeroengine bearing early fault detection. First, a comprehensive evaluation index is constructed via combining monotonicity, correlation, robustness index and entropy weight method to be used for initial screening sensitive degradation features from multi -view candidate degradation feature. After that, the hierarchical clustering algorithm and local preserving projection algorithm is introduced to realize sensitive degradation features clustering and dimensionality reduction. On this basis, the Euclidean distance sample reduction and the sparrow search algorithm is utilized to complete the training sample selection and model parameter optimization of different clusters and further use the verification samples to filter the optimal features and model. Finally, based on the optimal degradation features and models, a fusion performance degradation index is built to achieve intelligent detection of early fault. Comparisons with some existing fault detection methods, the proposed method improves degradation trend sensibility and demonstrates potential of good early fault detection engineering applications.
MoreTranslated text
Key words
Early fault detection,Aeroengine bearing,Two-stage feature optimization,Support vector data description,Sample reduction,Sparrow search algorithm
AI Read Science
Must-Reading Tree
Example
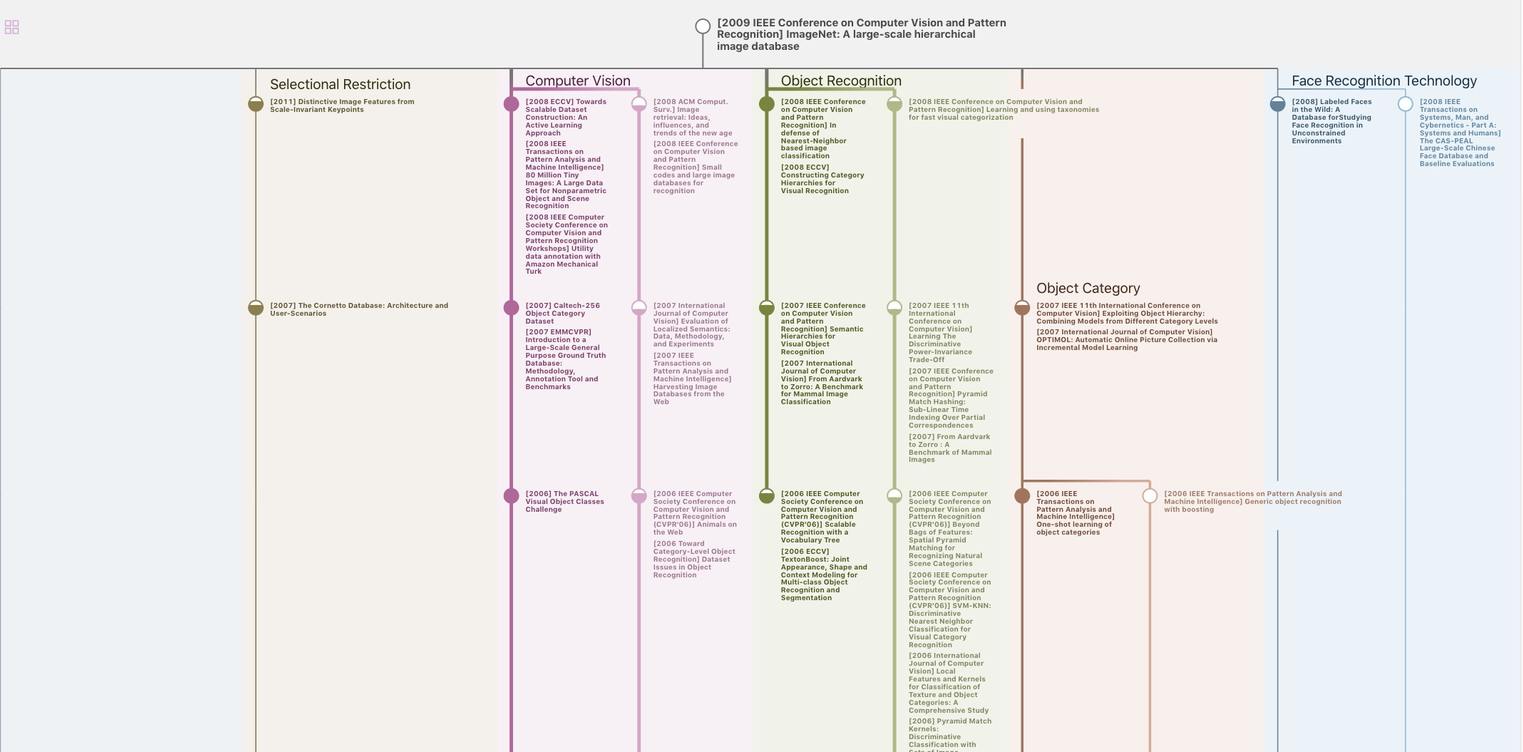
Generate MRT to find the research sequence of this paper
Chat Paper
Summary is being generated by the instructions you defined