SegNet: A segmented deep learning based Convolutional Neural Network approach for drones wildfire detection
REMOTE SENSING APPLICATIONS-SOCIETY AND ENVIRONMENT(2024)
Abstract
This research addresses the pressing challenge of enhancing processing times and detection capabilities in Unmanned Aerial Vehicle (UAV)/drone imagery for global wildfire detection, despite limited datasets. Proposing a Segmented Neural Network (SegNet) selection approach, we focus on reducing feature maps to boost both time resolution and accuracy significantly advancing processing speeds and accuracy in real-time wildfire detection. This paper contributes to increased processing speeds enabling real-time detection capabilities for wildfire, increased detection accuracy of wildfire, and improved detection capabilities of early wildfire, through proposing a new direction for image classification of amorphous objects like fire, water, smoke, etc. Employing Convolutional Neural Networks (CNNs) for image classification, emphasizing on the reduction of irrelevant features vital for deep learning processes, especially in live feed data for fire detection. Amidst the complexity of live feed data in fire detection, our study emphasizes on image feed, highlighting the urgency to enhance real-time processing. Our proposed algorithm combats feature overload through segmentation, addressing challenges arising from diverse features like objects, colors, and textures. Notably, a delicate balance of feature map size and dataset adequacy is pivotal. Several research papers use smaller image sizes, compromising feature richness which necessitating a new approach. We illuminate the critical role of pixel density in retaining essential details, especially for early wildfire detection. By carefully selecting number of filters during training, we underscore the significance of higher pixel density for proper feature selection. The proposed SegNet approach is rigorously evaluated using real -world dataset obtained by a drone flight and compared to state-of-the-art literature.
MoreTranslated text
Key words
Segment Neural Network,Machine learning,Unmanned Aerial Vehicle,Convolution Neural Network,Wildfire,Detection,Computer vision
AI Read Science
Must-Reading Tree
Example
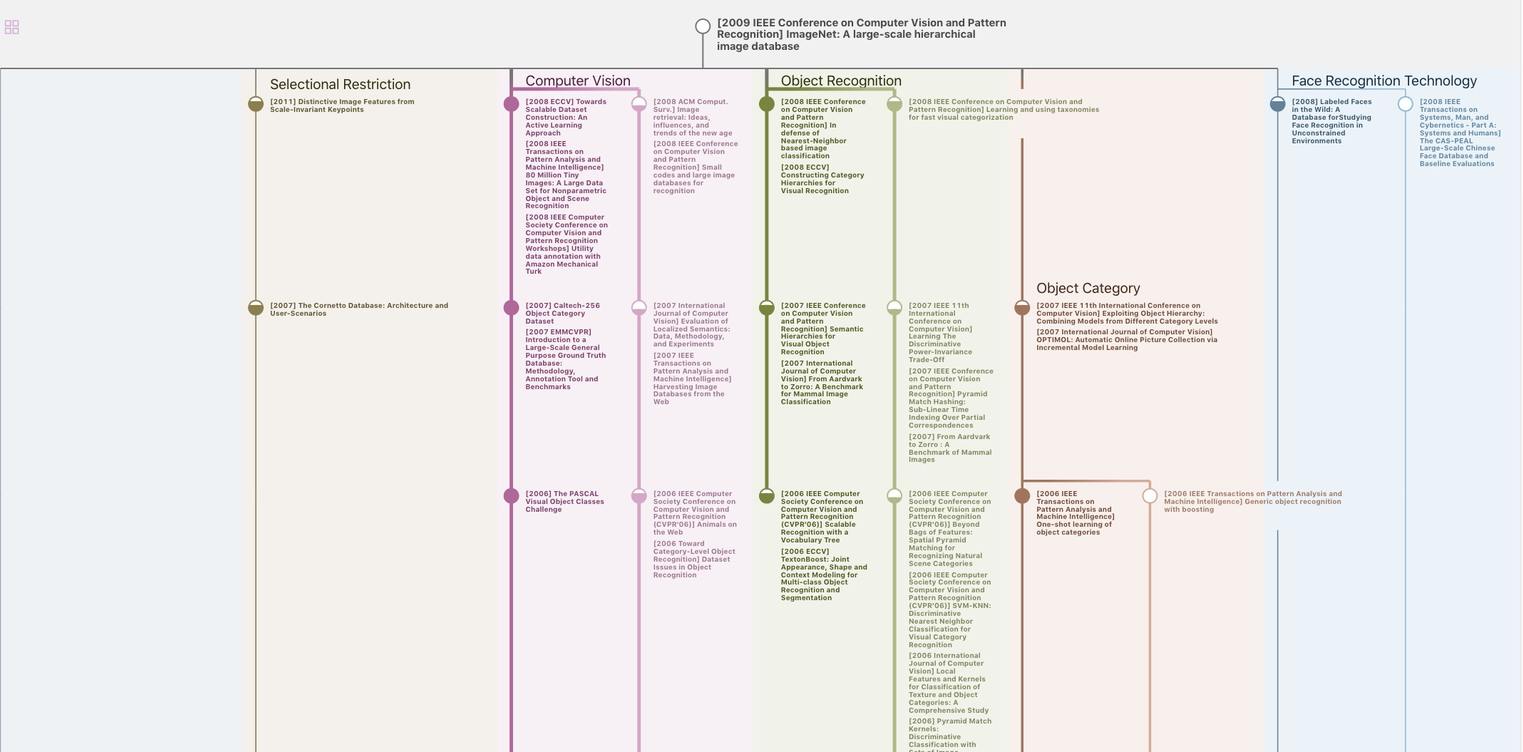
Generate MRT to find the research sequence of this paper
Chat Paper
Summary is being generated by the instructions you defined