Multi-sensor fusion fault diagnosis method of wind turbine bearing based on viewable neural networks
RELIABILITY ENGINEERING & SYSTEM SAFETY(2024)
摘要
Effective condition monitoring and fault diagnosis of rolling bearings, integral components of rotating machinery, are crucial for ensuring equipment reliability. However, existing diagnostic methods based on single signals perform poorly due to the detrimental effects of strong noise. Traditional deep learning approaches often neglect the interdependence between data samples when dealing with rolling bearing faults, thus constraining the accuracy and reliability of fault diagnosis. To tackle these challenges, this study introduces an intelligent diagnostic framework that integrates multi -source information at multiple levels, using acoustic and vibration signals (AVS) data and graph neural networks. Firstly, a data -level fusion method called Correlation Variance Contribution is proposed to effectively integrate vibration signals, addressing the issue of multisource information integration. An Adaptive Convergent Viewable Graph (AcvGraph) is introduced to optimize the representation of original AVS data and fused vibration signals, improving the capturing of correlation relationships within the data and enhancing classification accuracy. Furthermore, an enhanced DiffPool method is utilized to downsample the graph -structured data, reducing feature dimensions while preserving crucial information. Finally, the framework combines and integrates feature vectors from diverse inputs to form global feature vectors, enabling the accurate classification of rolling bearing faults. Exhaustive experiments validate the effectiveness of the proposed framework in utilizing AVS data for detecting different types of faults. Additionally, rigorous comparisons with alternative intelligent diagnosis techniques substantiate the superiority and advancements of the proposed method.
更多查看译文
关键词
Deep learning,Intelligent diagnosis,Acoustic and vibration signals,Multi-source information fusion,Graph neural networks
AI 理解论文
溯源树
样例
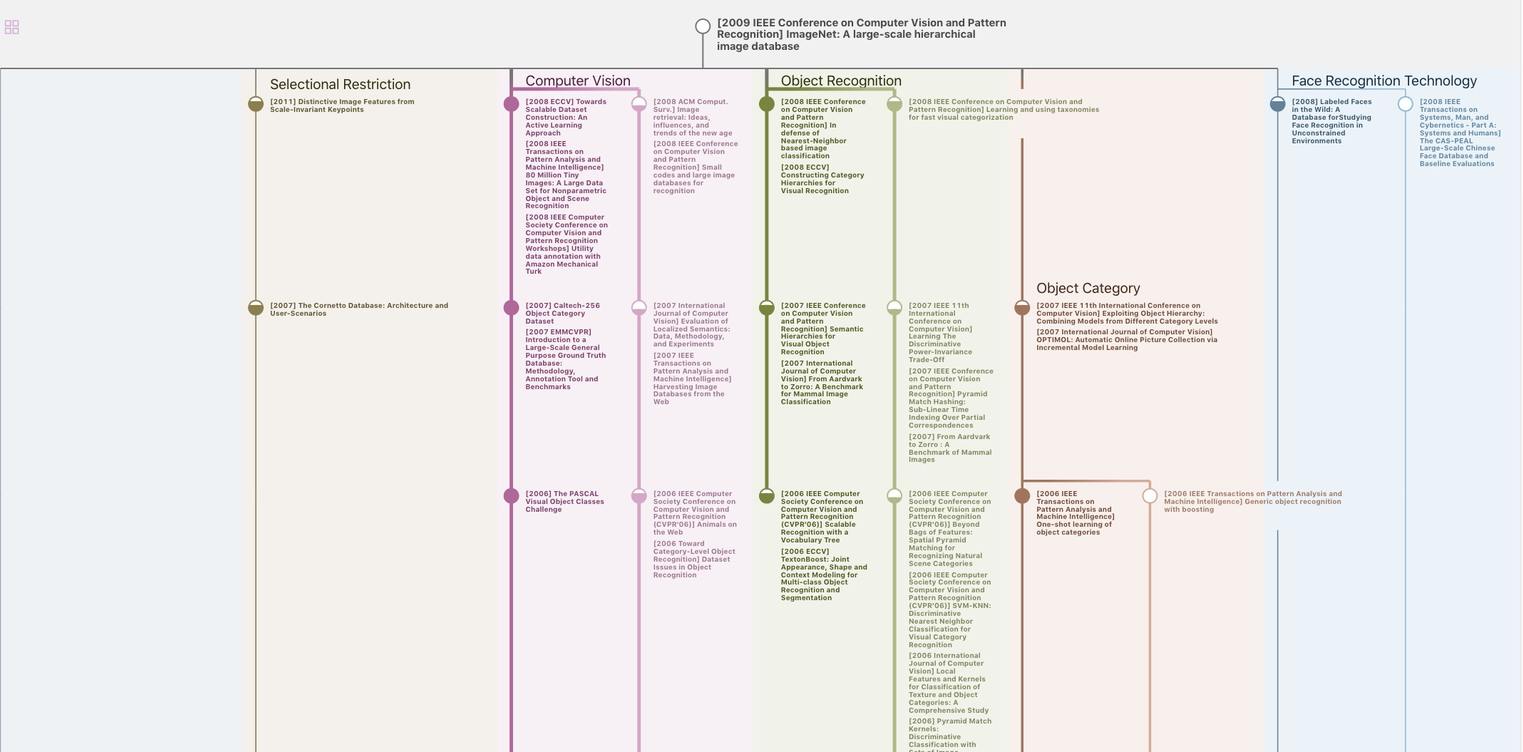
生成溯源树,研究论文发展脉络
Chat Paper
正在生成论文摘要