Transferable graph auto-encoders for cross-network node classification
PATTERN RECOGNITION(2024)
摘要
Node classification is a popular and challenging task in graph neural networks, and existing approaches are mainly developed for a single network. With the advances in domain adaptation, researchers tend to leverage knowledge extracted from a fully -labeled source network to further improve the node classification performance in an unlabeled target network. This learning paradigm refers to cross -network node classification, which is the topic we studied in this paper. Specifically, we propose a novel model named Transferable Graph Auto -Encoders (TGAE), which first encodes the initial network data into latent representations and then decodes the learned features to preserve graph information. In the encoding phase, TGAE adopts the attentional mechanism to fuse the local and global information of nodes to discover latent node representations. To obtain transferable features between the source and target networks, TGAE aligns their distributions based on the learned representations by reducing marginal and conditional distribution differences. In the decoding phase, the latent representations are subjected to pairwise and reconstruction constraints, thus preserving structural proximity and graph topology information to learn discriminative features. Besides, a node classifier is trained to enhance the discriminant of the node representations further. Experimental results on several real -world datasets demonstrate that the proposed model achieves state-of-the-art performance in cross -network node classification tasks compared with existing methods.
更多查看译文
关键词
Cross-network node classification,Transfer learning,Domain adaptation,Graph auto-encoder,Graph convolutional network
AI 理解论文
溯源树
样例
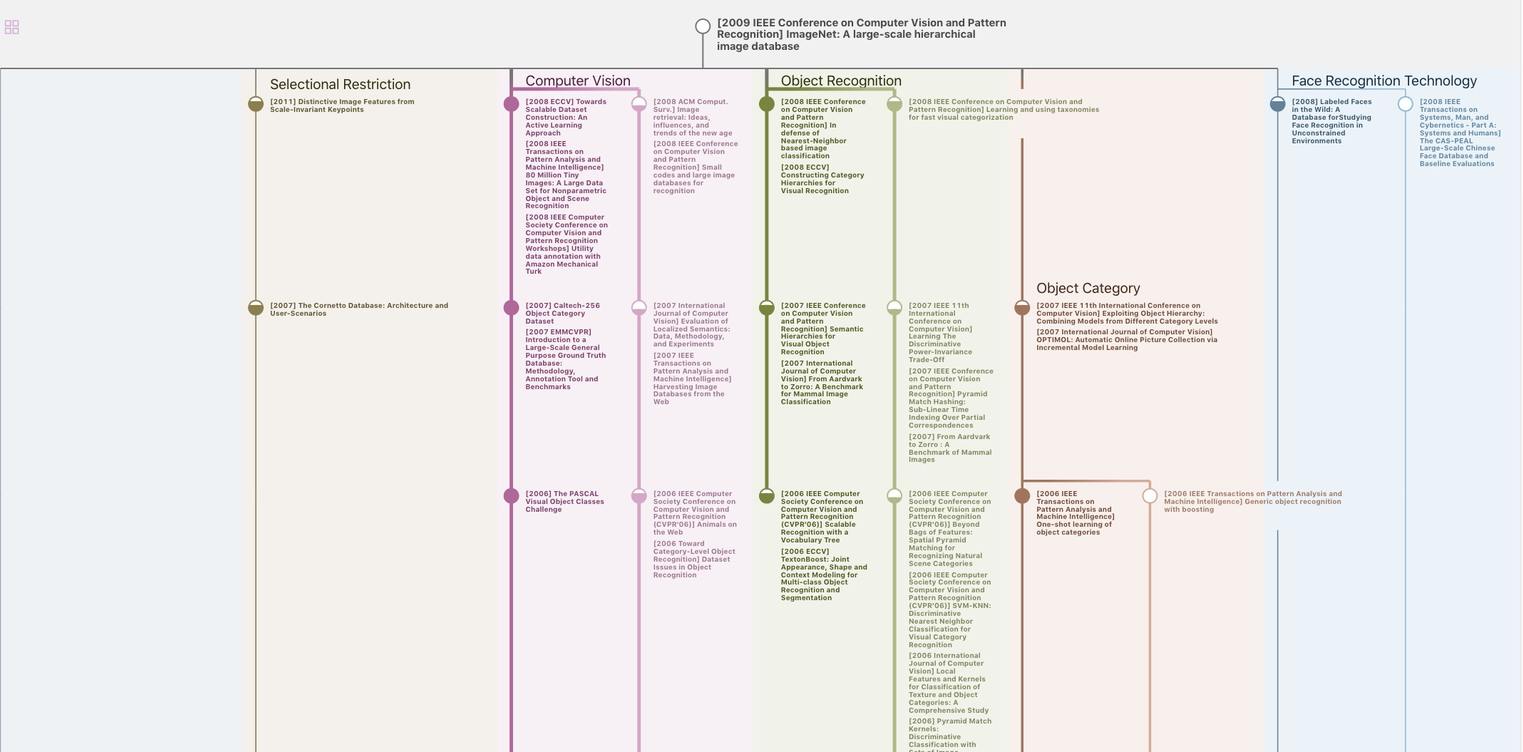
生成溯源树,研究论文发展脉络
Chat Paper
正在生成论文摘要