MHSAN: Multi-view hierarchical self-attention network for 3D shape recognition
Pattern Recognition(2024)
摘要
Multi-view learning has demonstrated promising performance for 3D shape recognition. However, existing multi-view methods usually focus on fusing multiple views and ignore the structural and discriminative information carried by 2D views. In this paper, we propose a multi-view hierarchical self-attention network (MHSAN) to explore the geometric and discriminative information from complex 2D views. Specifically, MHSAN consists of two self-attention networks. First, a global self-attention network is adopted to exploit the structure information by embedding position information of views. Then, the discriminative self-attention network learns discriminative information from the views with high classification scores. Through the proposed MHSAN, the geometric and discriminative information is condensed as the novel representation of 3D shapes. To validate the effectiveness of our proposed method, extensive experiments have been conducted on three 3D shape benchmarks. Experimental results demonstrate that our method is generally superior to the state-of-the-art methods in 3D shape classification and retrieval tasks.
更多查看译文
关键词
3D shape recognition,Self-attention,Multi-view learning,View aggregation
AI 理解论文
溯源树
样例
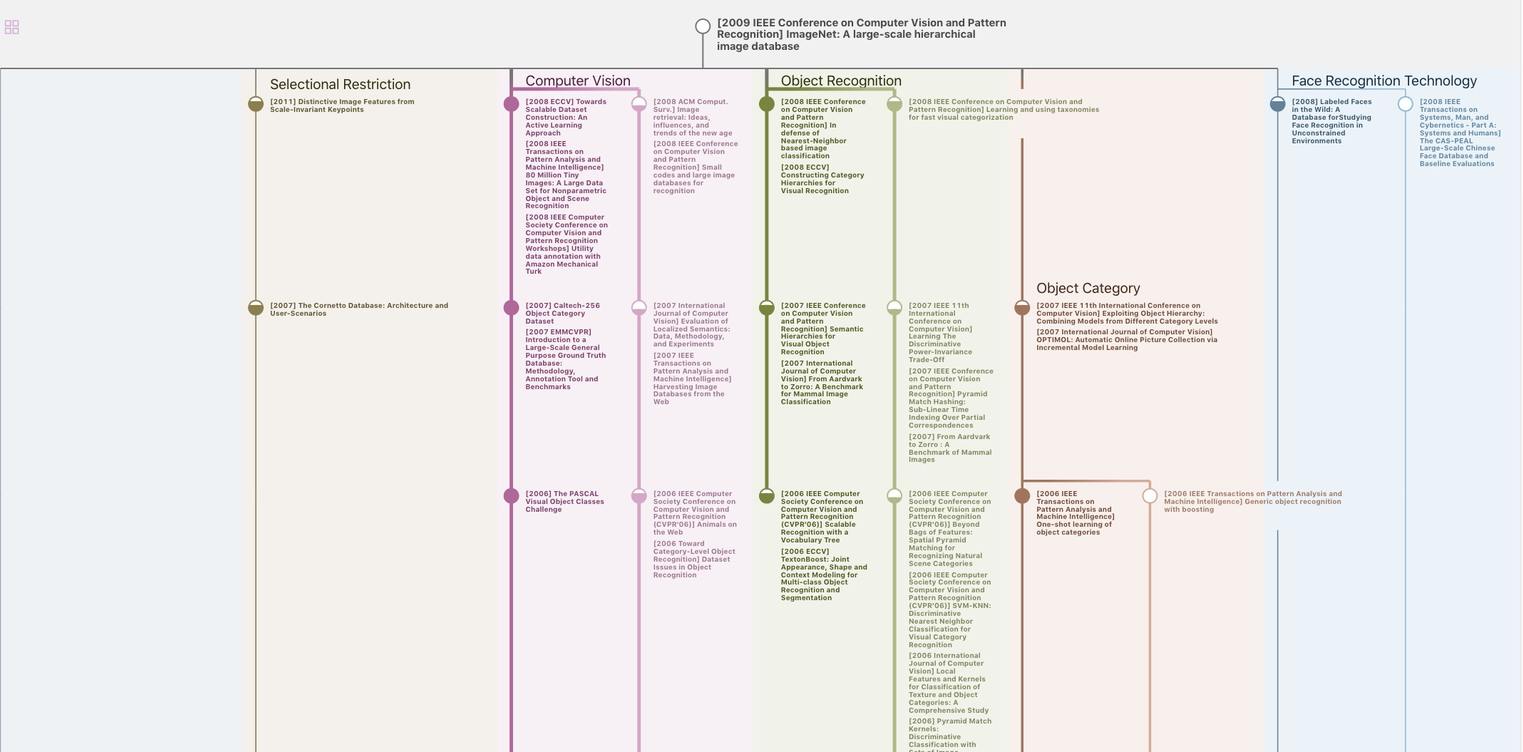
生成溯源树,研究论文发展脉络
Chat Paper
正在生成论文摘要