Comprehensive assessment of triclustering algorithms for three-way temporal data analysis
PATTERN RECOGNITION(2024)
摘要
The analysis of temporal data has gained increasing attention in recent years, aiming to identify patterns and trends that change over time. Temporal triclustering is a promising approach for this purpose, as it allows for the simultaneous clustering of three dimensions of data: objects, attributes, and time. In this work, we present a comparative study and experimental evaluation of state-of-the-art temporal triclustering algorithms. Our study provides a comprehensive quantitative assessment of several triclustering algorithms to unravel their strengths and limitations. To this end, we consider synthetic data with varying sizes and regularities, where true solutions are planted with different coherence and quality criteria, in order to assess the algorithms' performance in datasets with diverse characteristics and assess their capacity to retrieve specific types of hidden patterns. This provides a more comprehensive evaluation of the algorithms and allows for a better understanding of their capabilities and limitations. This study is the first to compare state-of-the-art triclustering algorithms inherently prepared to deal with temporal data and provides new benchmark results for the Temporal Triclustering task. Our results on the algorithms' performance can guide practitioners in selecting the most appropriate algorithm for their specific application.
更多查看译文
关键词
Triclustering,Temporal data,Three-way data
AI 理解论文
溯源树
样例
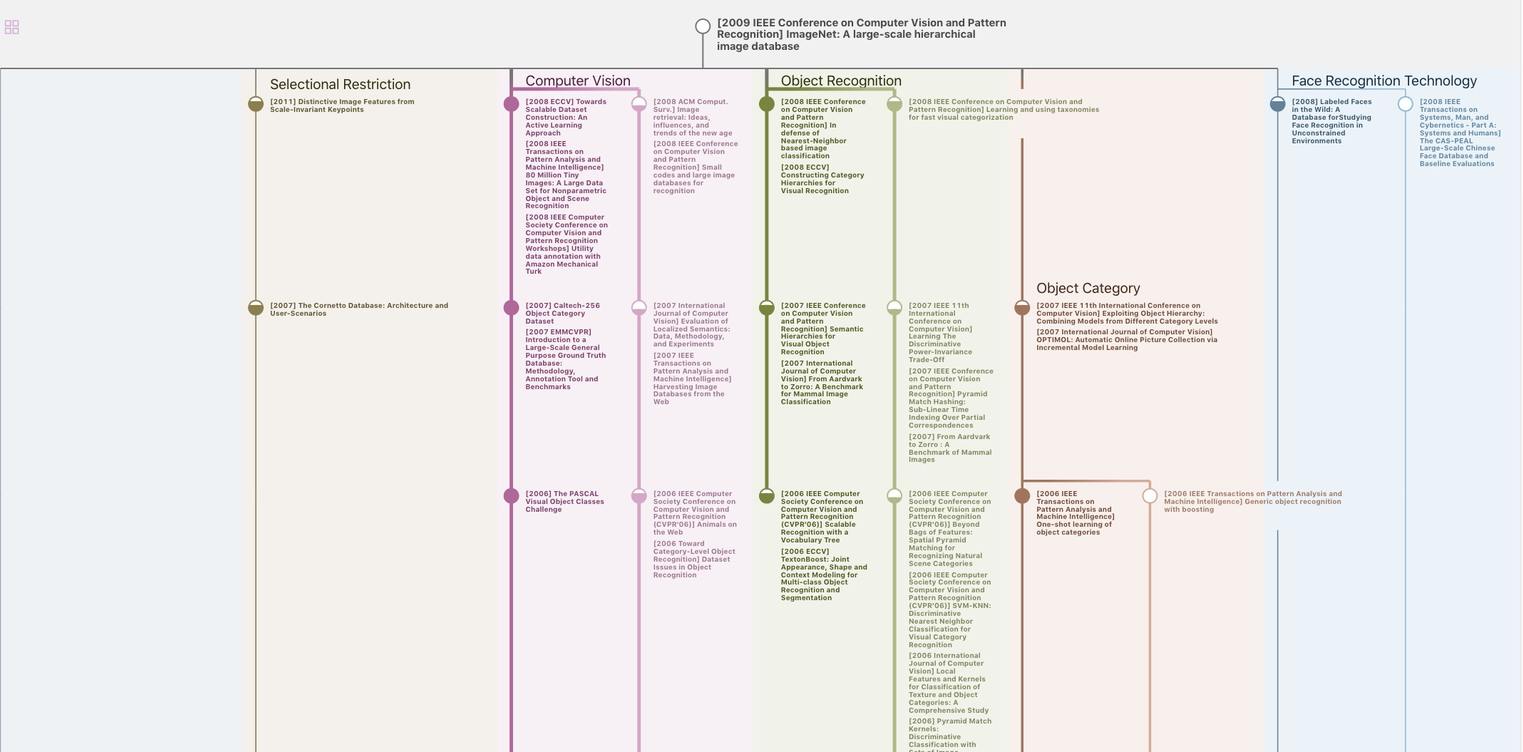
生成溯源树,研究论文发展脉络
Chat Paper
正在生成论文摘要