Framework for automatic production simulation tuning with machine learning
Procedia CIRP(2024)
摘要
Production system simulation is a powerful tool for optimizing the use of resources on both the planning and control level. However, creating and tuning such models manually is a tedious and error-prone task. Despite some approaches to automate this process, the state-of-the-art relies on the generation of models, by incorporating the knowledge of experts. Nevertheless, effectively creating and tuning such production simulations is, thus, a key driver for reducing costs, carbon footprint, and tardiness and therefore an essential factor in today´s production. Beneficial would be automated and flexible frameworks, since these are applicable to different use cases requiring less effort. Yet, in the age of Industry 4.0, data is ubiquitous and easily available and can serve as a basis for virtual models representing reality. Increasingly, these virtual models shall be interlinked with the current state of real-world systems to form so-called digital twins. As automated and flexible frameworks are missing, this paper proposes a novel approach where observed real system behavior is used and fed into a large-scale machine learning model trained on a plethora of possible parameter sets. The main target is to train this machine learning model to minimize the reality gap between the behavior of the simulated and real system by selecting corresponding simulation system parameters. By estimating those parameters an enhancement of the simulation will emerge. An interlink to real systems can be derived resulting in a digital shadow which is capable to forecast the future similarly to reality. The approach to overcoming the gap between reality and simulation (real2sim) is validated in simulations.
更多查看译文
关键词
Production Simulation,Machine Learning,Simulation Tuning,Simulation Generation
AI 理解论文
溯源树
样例
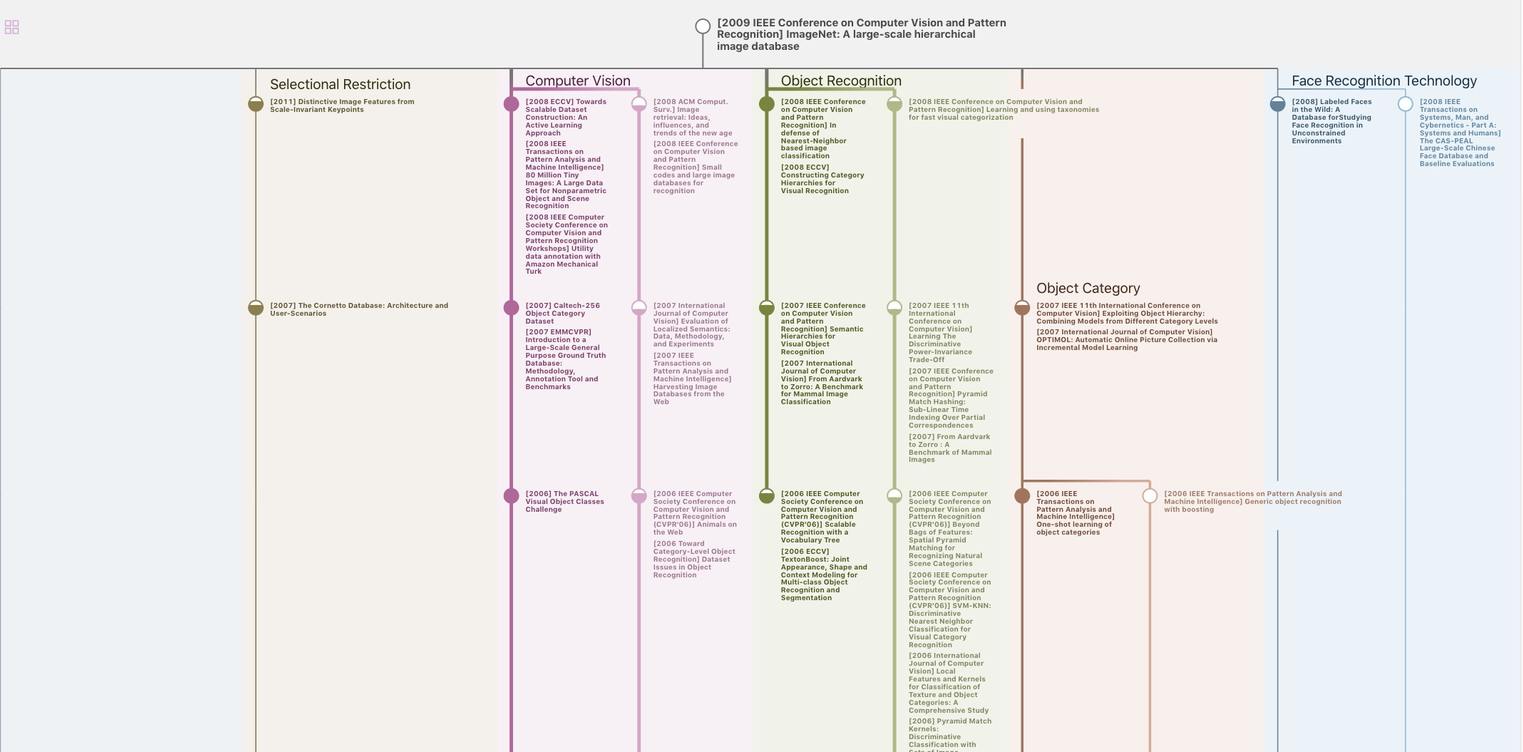
生成溯源树,研究论文发展脉络
Chat Paper
正在生成论文摘要