An approach for handwritten Chinese text recognition unifying character segmentation and recognition
Pattern Recognition(2024)
摘要
Text line recognition methods can be categorized into explicit segmentation based and implicit segmentation based ones. Explicit segmentation based methods require character-level annotation during training, while implicit segmentation based methods, trained on line-level annotated data, face alignment drift challenges. Though some methods have been proposed to address these challenges using weakly supervised object detection, they often rely on cumbersome pseudo-box generation processes and complex decoding. In this paper, we propose a unified framework to overcome these challenges, achieving high accuracy in text recognition and character segmentation. To eliminate the need of character-level annotated real text line data in training, we introduce a novel training paradigm that utilizes character-level annotated synthetic data and line-level annotated real data jointly. For synthetic data, candidate characters are explicitly aligned with labeled characters to generate hard labels for supervising model training. For real data, implicit alignment is produced by Connectionist Temporal Classification (CTC) mapping to provide soft labels for weakly-supervised model training. And for inference, we propose two decoding strategies leveraging the advantages of Non-Maximum Suppression (NMS) and CTC decoding. Extensive experiments on benchmark datasets demonstrate the superior performance of our method in text recognition and character localization, even with minimal amounts of character-level annotated line data.
更多查看译文
关键词
Handwritten Chinese text recognition,Connectionist temporal classification,Character segmentation,Weakly supervised learning
AI 理解论文
溯源树
样例
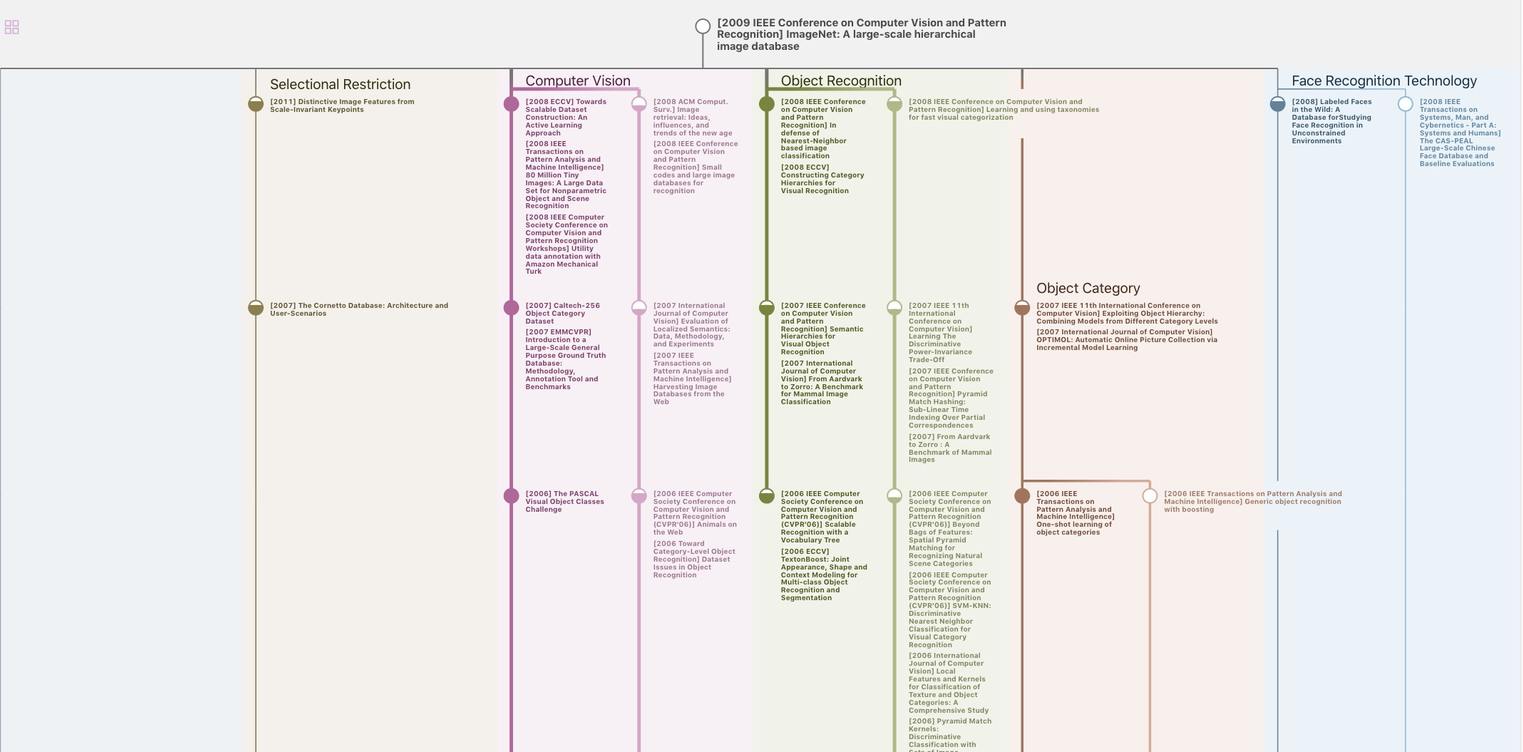
生成溯源树,研究论文发展脉络
Chat Paper
正在生成论文摘要