A data-driven data-augmentation method based on Slim-generative Adversarial Imputation Networks for short-term ship-motion attitude prediction
OCEAN ENGINEERING(2024)
摘要
The performance of data -driven complex ship -motion attitude prediction models not only depends on the structure and algorithm design of the model but is also affected by the quantity and quality of training samples. Insufficient available data, resulting from factors such as low sampling rates and high costs, could inhibit the achievement of optimal prediction accuracy. This limitation in turn increases the model's expected generalization error of the model and causes overfitting in regression models. Nevertheless, by artificially expanding the training dataset, data -augmentation methods enhance the resistance of the model to overfitting, thereby improving the prediction performance. This study introduces a novel data -driven model for ship -motion prediction based on the principles of Slim Generative Adversarial Imputation Networks (SGAINs). The model not only increases the amount of data but also considers temporal correlation features in the enhancement process, thus uniquely addressing the issue of poor accuracy of prediction models caused by insufficient training datasets. The simulation results demonstrate the effectiveness and superiority of the proposed model for short-term ship -motion attitude data augmentation.
更多查看译文
关键词
Sensor data augmentation,Deep learning,Generative adversarial imputation networks,Ship motion attitude prediction
AI 理解论文
溯源树
样例
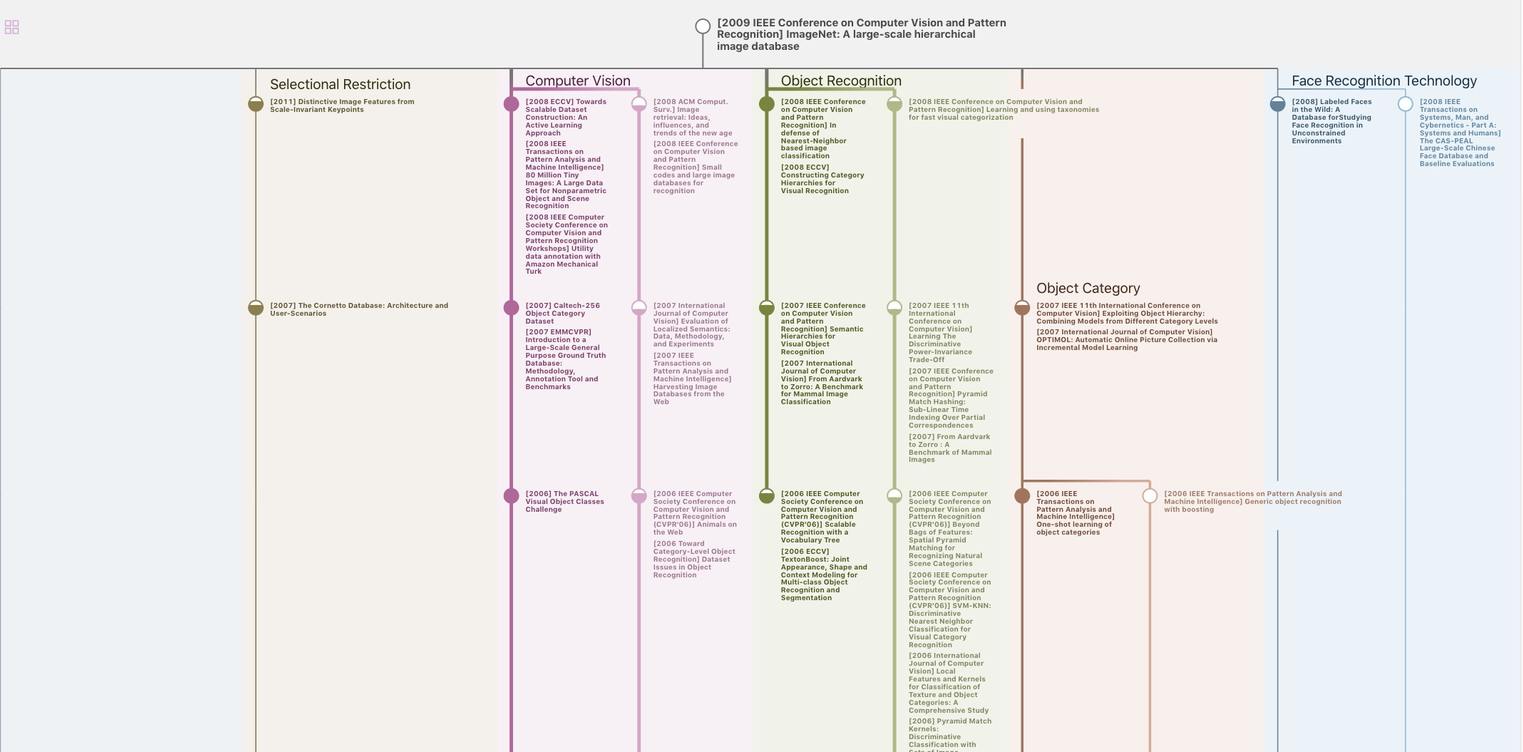
生成溯源树,研究论文发展脉络
Chat Paper
正在生成论文摘要