A review of dose prediction methods for tumor radiation therapy
Meta-Radiology(2024)
摘要
Radiation therapy (RT) is currently the main clinical treatment of tumors. Before treatment initiation, precise delineation of the planned target volume (PTV) and organs at risk (OAR) is essential. This segmentation, together with the dose prediction algorithm, aids in the calculation and evaluation of the dose distribution, and ultimately in the refinement of the treatment plan. To provide a comprehensive view of the current landscape of research on dose prediction methods, we meticulously collected and summarized papers published between 2017 and 2023. First, we present our rigorous literature search approach, providing a statistical analysis of the pooled papers and an elaborate overview of the evaluation metrics that are commonly and consistently employed in this domain. Then, we focus on a detailed survey of the evolutionary trajectories of dose prediction methods. This comprehensive investigation covers a spectrum ranging from traditional Knowledge-Based Planning (KBP) methods to emerging deep learning-based methods, which include input improvement methods, U-Net-based methods, GAN-based methods, and other deep learning-based methods. Throughout this exposition, we have carefully outlined the strengths and limitations inherent in these various approaches. Finally, we conclude with a summary of the primary challenges facing the field and propose several prospective research directions to effectively address them.
更多查看译文
关键词
Automated treatment planning,Deep learning,Knowledge-based planning,Radiation dose prediction
AI 理解论文
溯源树
样例
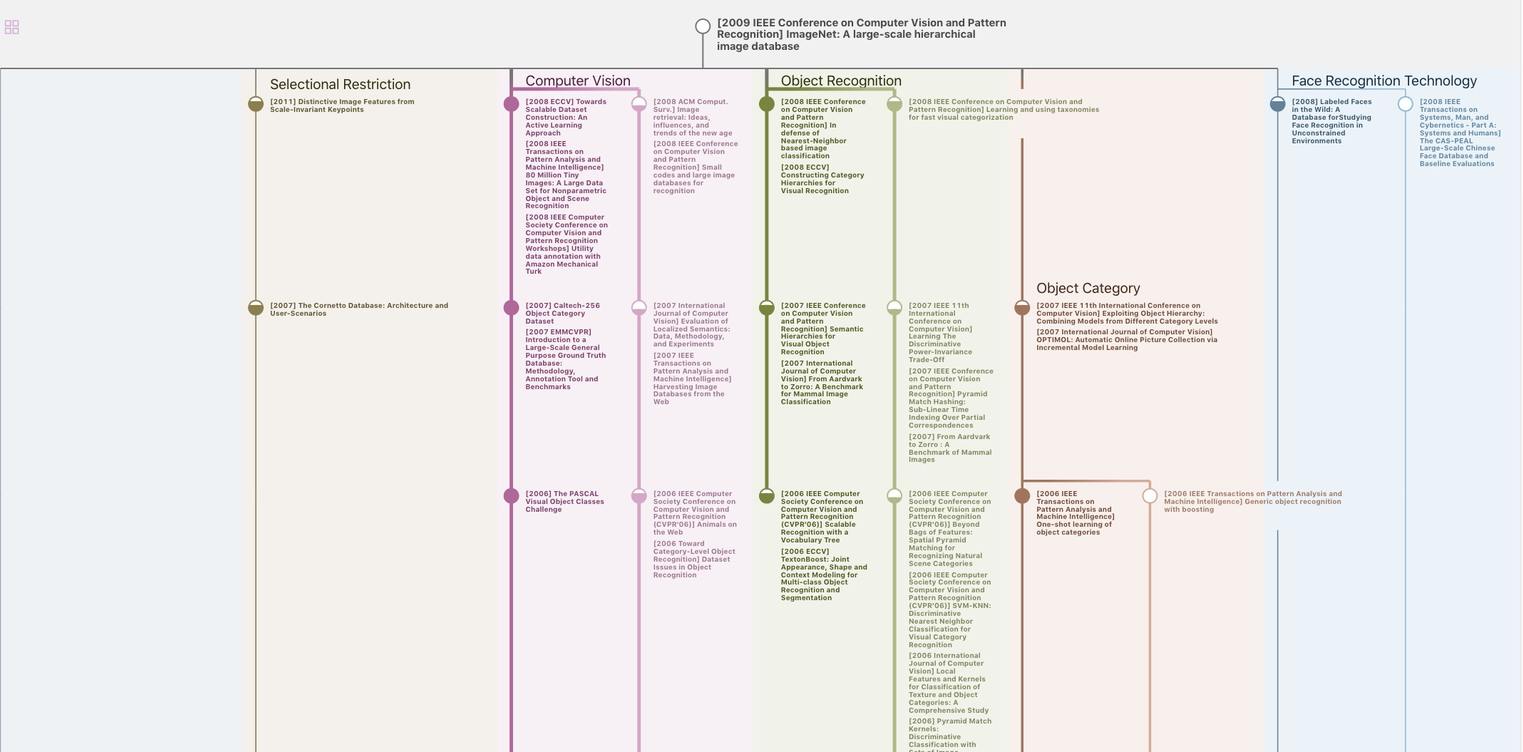
生成溯源树,研究论文发展脉络
Chat Paper
正在生成论文摘要