Contrastive feature-based learning-guided elevated deep reinforcement learning: Developing an imbalanced fault quantitative diagnosis under variable working conditions
MECHANICAL SYSTEMS AND SIGNAL PROCESSING(2024)
摘要
Fault diagnosis is subject to the challenge of implementing model learning in the presence of small samples and imbalanced data (i.e., variable operating conditions), which is a fundamental and crucial problem that hinders their applications in real industrial scenarios. Herein, a novel deep reinforcement learning strategy (SIMC-PERDRL) that combines SimCLR and elevated prioritized experience replay (PER) is proposed for machinery fault quantitative diagnosis in nonideal data scenarios. First, unsupervised contrastive learning pre-trains the feature extraction layer to mine optimal discriminative features with more optimal intra-class compactness and inter-class separability to reduce inter-class overlap. Second, the experience priority is quantified by reward and TD error to enhance the learning frequency of rare high-value samples; the reward function is skillfully constructed using adaptive unbalanced distribution, which immensely increases the agent's sensitivity to minorities, and enhances the model's domain adaptability by dynamically fine-tuning the agent's decision through real-time feedback. Moreover, ResNet utilizes the Convolutional Block Attention Module (CBAM) to construct a deep Q-network; thus, the agent's learning ability of critical fault features is enhanced. Finally, SIMC-PERDRL was validated online using three rotating machinery datasets. The results indicate that the method can automatically realize accurate qualitative identification under different rotational speeds, different loads, and class unbalanced conditions, with excellent effectiveness, stability, and versatility.
更多查看译文
关键词
Fault quantitative diagnosis,Class imbalance,Dueling Double Deep Q Network (D3QN),Contrastive Learning,Prioritized experience replay
AI 理解论文
溯源树
样例
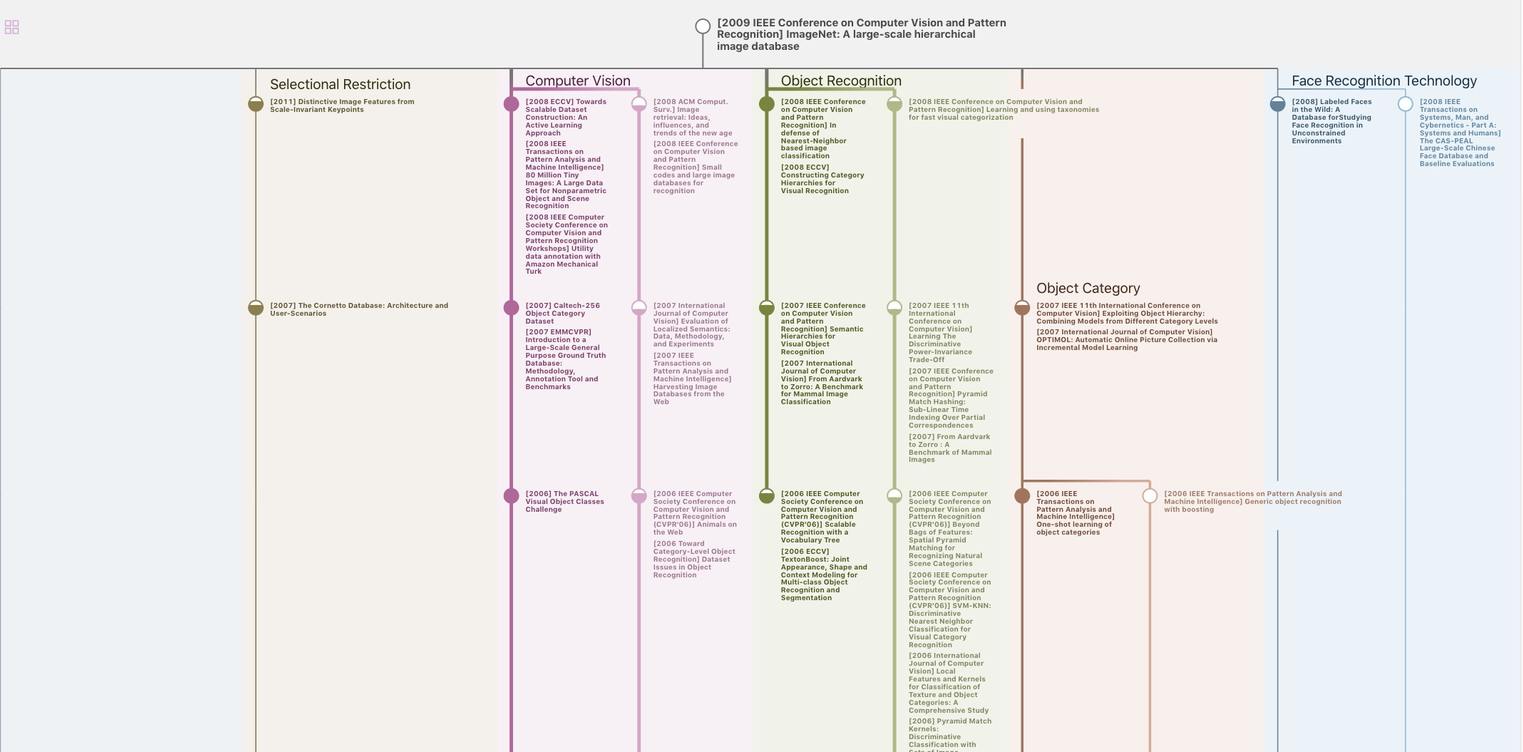
生成溯源树,研究论文发展脉络
Chat Paper
正在生成论文摘要