Machine learning as a characterization method for analysis and design of perovskite solar cells
MATERIALS TODAY PHYSICS(2024)
Abstract
Device-level characterization is the primary means of testing the performance of a device and identifying the microscale factors that affect its performance. With the accumulation of data on perovskite solar cells (PSCs), machine learning (ML) may become a potential tool for characterizing properties of PSCs. Here, we use ML techniques and 698 sets of rigorously cleaned experimental data to construct ML characterization of device properties for PSCs. The applicable range of the constructed ML characterization is 20.7 % of PSCs. It not only accurately predicts the four photovoltaic parameters ((power conversion efficiencies (PCE), short-circuit current density (Jsc), open-circuit voltage (Voc), fill factor (FF)) and EQE curves,but also analyze the game effects of four major types of different scale features (perovskite, transport barriers, transport materials, cell architecture). ML characterization displays an incredible ability for performance prediction and analysis of multiscale data. In particular, it produces a series of microscale findings with experimental guiding and material/device design significance, which may require a large number of experiments to be obtained. These results show that devicelevel characterization through ML can save a lot of time, resources and has huge potential for applications.
MoreTranslated text
Key words
Perovskite solar cells,Device characterization,SHAP,Interpretable machine learning,Feature interaction
AI Read Science
Must-Reading Tree
Example
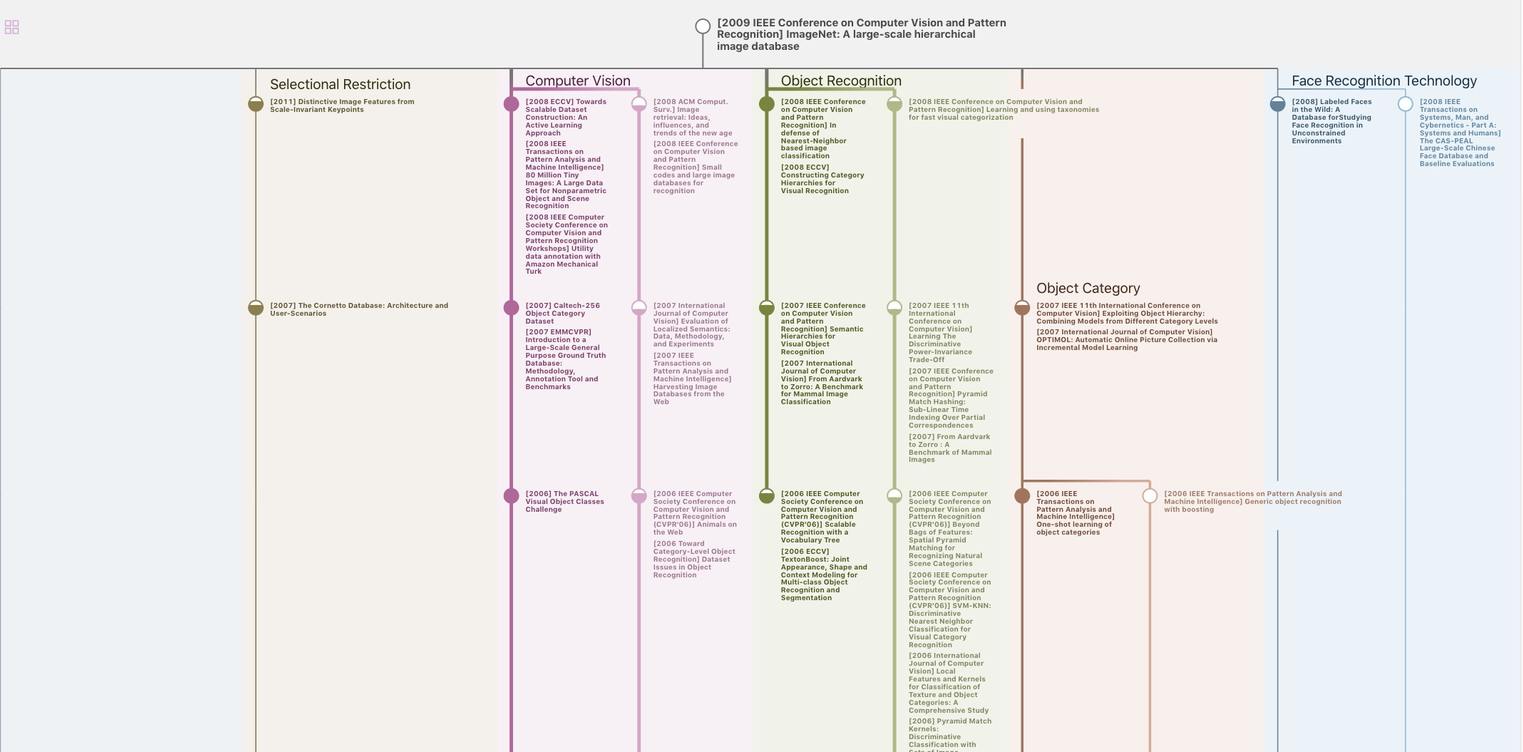
Generate MRT to find the research sequence of this paper
Chat Paper
Summary is being generated by the instructions you defined