RLTube: Reinforcement learning based deposition path planner for thin-walled bent tubes with optionally varying diameter manufactured by wire-arc additive manufacturing
MANUFACTURING LETTERS(2024)
摘要
This study presents RLTube, an algorithm that uses reinforcement learning (RL) to compute the deposition path for thin-walled bent tubes produced by wire-arc additive manufacturing. Rigid mathematical rules are used by state-of-the-art methods and the developed Brute Force Approach (BFA) to achieve this goal. In contrast, RLTube offers greater flexibility, adaptability and efficiency. This RL-based architecture uses 2D images of bent tubes as input, eliminating the need for additional feature extraction steps. As a result, RLTube deposition paths outperform BFA in terms of the developed evaluation criteria reflecting their quality. (c) 2024 The Author(s). Published by Elsevier Ltd on behalf of Society of Manufacturing Engineers (SME). This is an open access article under the CC BY license (http://creativecommons.org/licenses/by/4.0/).
更多查看译文
关键词
Wire -arc additive manufacturing,Machine learning,Reinforcement learning,Path planning,Bent tube
AI 理解论文
溯源树
样例
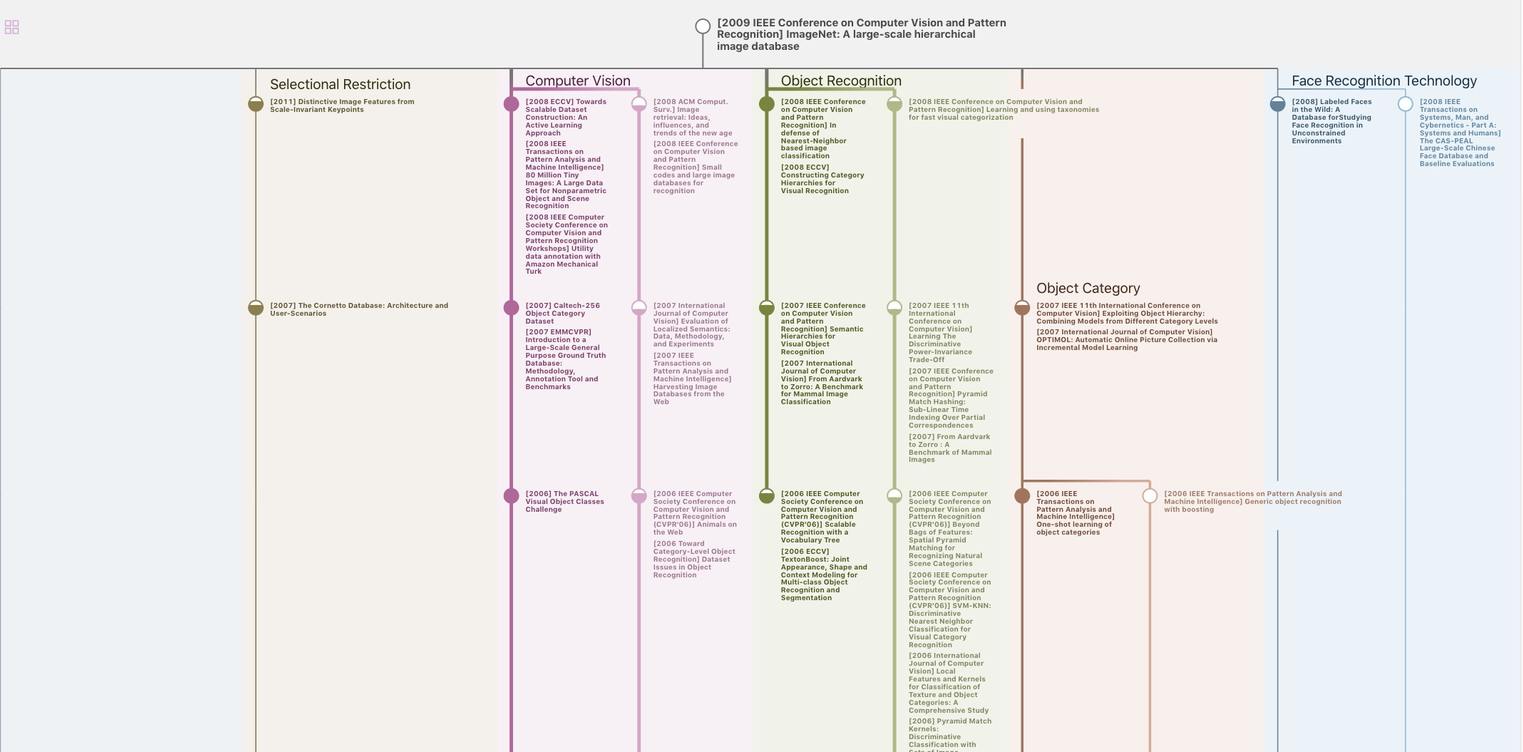
生成溯源树,研究论文发展脉络
Chat Paper
正在生成论文摘要