Correlation-attention guided regression network for efficient crowd counting
JOURNAL OF VISUAL COMMUNICATION AND IMAGE REPRESENTATION(2024)
摘要
As a valuable component of intelligent video surveillance, crowd counting has received lots of attention. In practice, however, crowd counting always suffers from the problem of the scale change of pedestrians. To mitigate this limitation, we propose a novel correlation -attention guided regression network to estimate the number of people, termed CGR-Net. To make the generation process of spatial attention and channel attention independent of each other, we design a parallel channel/spatial-wise attention module (PCSAM) to avoid error accumulation. A pixel -wise assisted attention module (PAAM) is developed for learning crowd uneven distribution on the different image pixels to further enhance the ability of the CGR-Net. Furthermore, we present a new loss function to ensure the effectiveness and performance of the proposed method. Comprehensive experimental results demonstrate that our model delivers enhanced representation and attains state-of-the-art performance.
更多查看译文
关键词
Crowd counting,Crowd density estimation,Attention mechanism,Regression
AI 理解论文
溯源树
样例
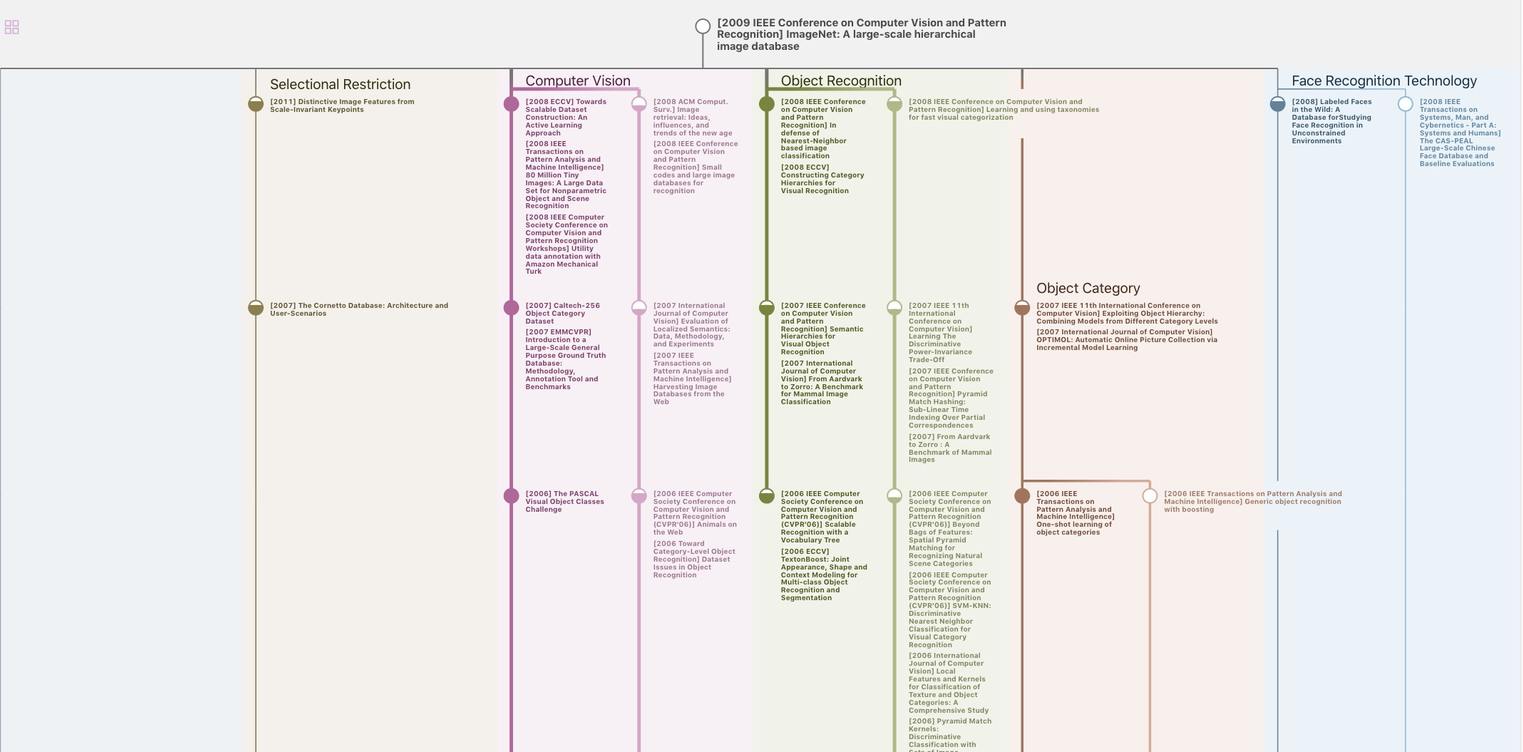
生成溯源树,研究论文发展脉络
Chat Paper
正在生成论文摘要