Multi-agent reinforcement learning-enhanced autonomous calibration method for wastewater treatment modeling: Long-term validation of a full-scale plant
JOURNAL OF WATER PROCESS ENGINEERING(2024)
摘要
Wastewater modeling using an activated sludge model (ASM) has been used in water industries but is still known as the difficult and challenging model for full-scale wastewater treatment plants (WWTPs) due to its mathematical nonlinearities and the complex interactions between the ASM model parameters. This study suggests a novel autonomous calibration method for the ASM model using multi -agent reinforcement learning (MARL) which can search the feasible parameter spaces and automatically calibrate the kinetic parameters of the ASM model. Eight kinetic parameters in the ASM-soluble microbial product (ASM-SMP) model are selected to be calibrated for describing the biological nutrient removal processes. A game abstraction mechanism based on a two -stage attention network (G2ANet)-one of the MARL algorithms-dynamically suggests the calibrated values of the eight parameters to describe the activities of microorganisms by considering the biological interactions between the parameters. The G2ANet automatically decides the kinetic parameter values by observing the influent characteristics of a target plant without any guidance by the operators. The G2ANet-enhanced calibrated model is satisfactorily accurate, showing good agreement with measured values of effluent chemical oxygen demand (COD) and total nitrogen (TN) concentrations; it decreases the modeling errors by 87 % and 58 % for COD and TN, respectively. Its performance is additionally evaluated by analyzing the biological process rates of the biomass from the auto -calibrated model. Moreover, its reliability guarantees its feasible application to a real WWTP by verifying its calibrated biological characteristics under the varying different influent conditions for seasonal months.
更多查看译文
关键词
Activated sludge model,Model calibration,Multi-agent reinforcement learning,Artificial intelligence,Wastewater treatment process
AI 理解论文
溯源树
样例
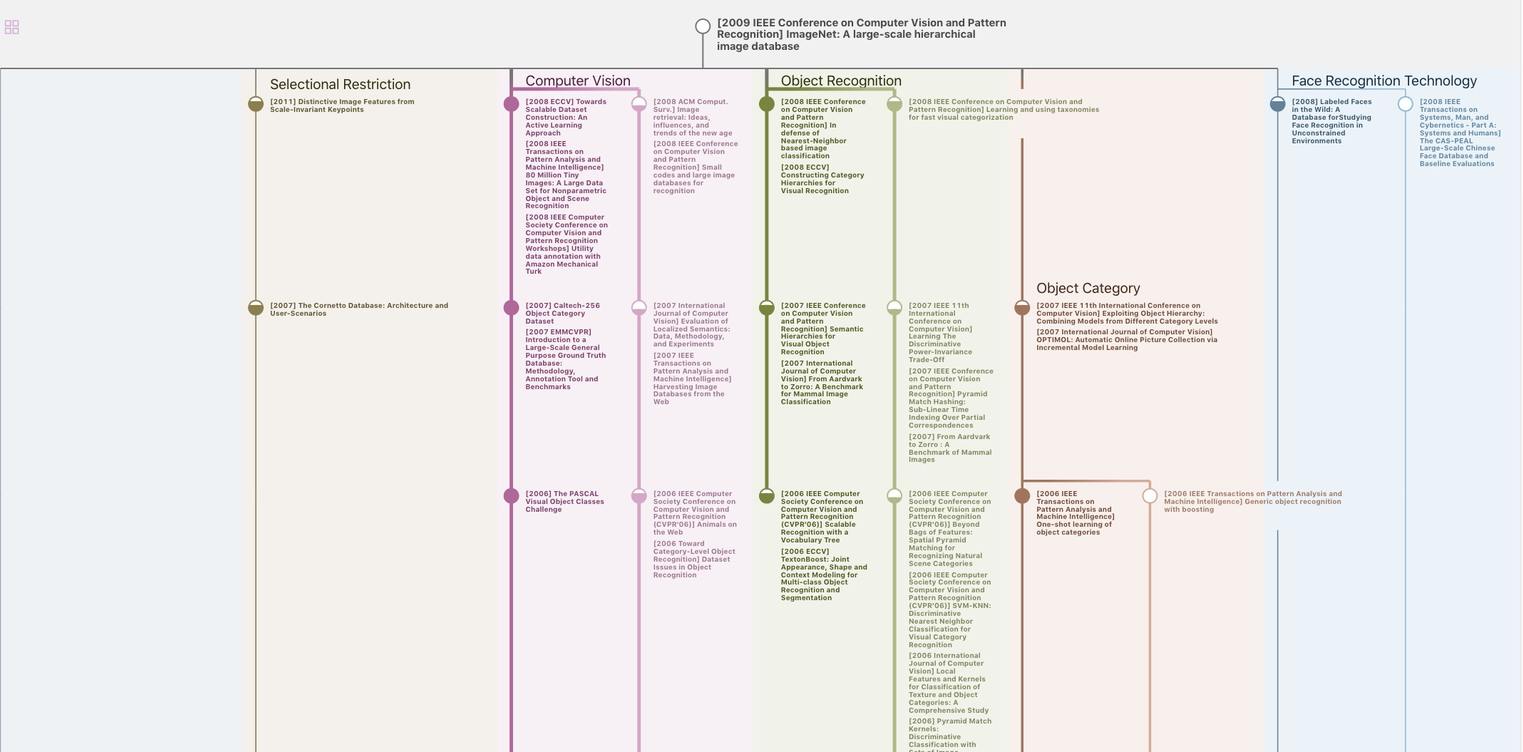
生成溯源树,研究论文发展脉络
Chat Paper
正在生成论文摘要