Online penetration prediction based on multimodal continuous signals fusion of CMT for full penetration
JOURNAL OF MANUFACTURING PROCESSES(2024)
摘要
Online penetration monitoring for complex butt welding is challenging due to steel plate's groove instability and welding heat deformation. In this paper, automatic cold metal transfer (CMT) welding is used to join two complex bevelled austenitic stainless steel with SS304 as the base metal. This work reports a hybrid approach combining deep learning, computer vision, and sound signal processing to monitor groove welding penetration under full penetration in real time. Sequence signals such as video and sound can complimentarily characterize the melt pool state. In this paper, the proposed Multimodal continuous signals Characteristic Reinforcement Network (MCRNet) utilizes 3D convolution and multiscale convolution with channel attention to considerably improve the performance of lightweight networks. At the same time, a new fusion method with similarity loss is proposed to cope with the input of visual and acoustic signals. That improves the effect by at least 18 % compared with the single-modal signal input. The experimental results show that the Mean Square Error (MSE) of MCRNet improved the performance by 44 % compared with the mainstream deep learning framework. Meanwhile, the inference speed under multimodal input reaches 57 frames per second (FPS). MCRNet finally achieves online penetration accurate prediction of the melt pool.
更多查看译文
关键词
Audio-visual signal,Penetration state,Deep learning
AI 理解论文
溯源树
样例
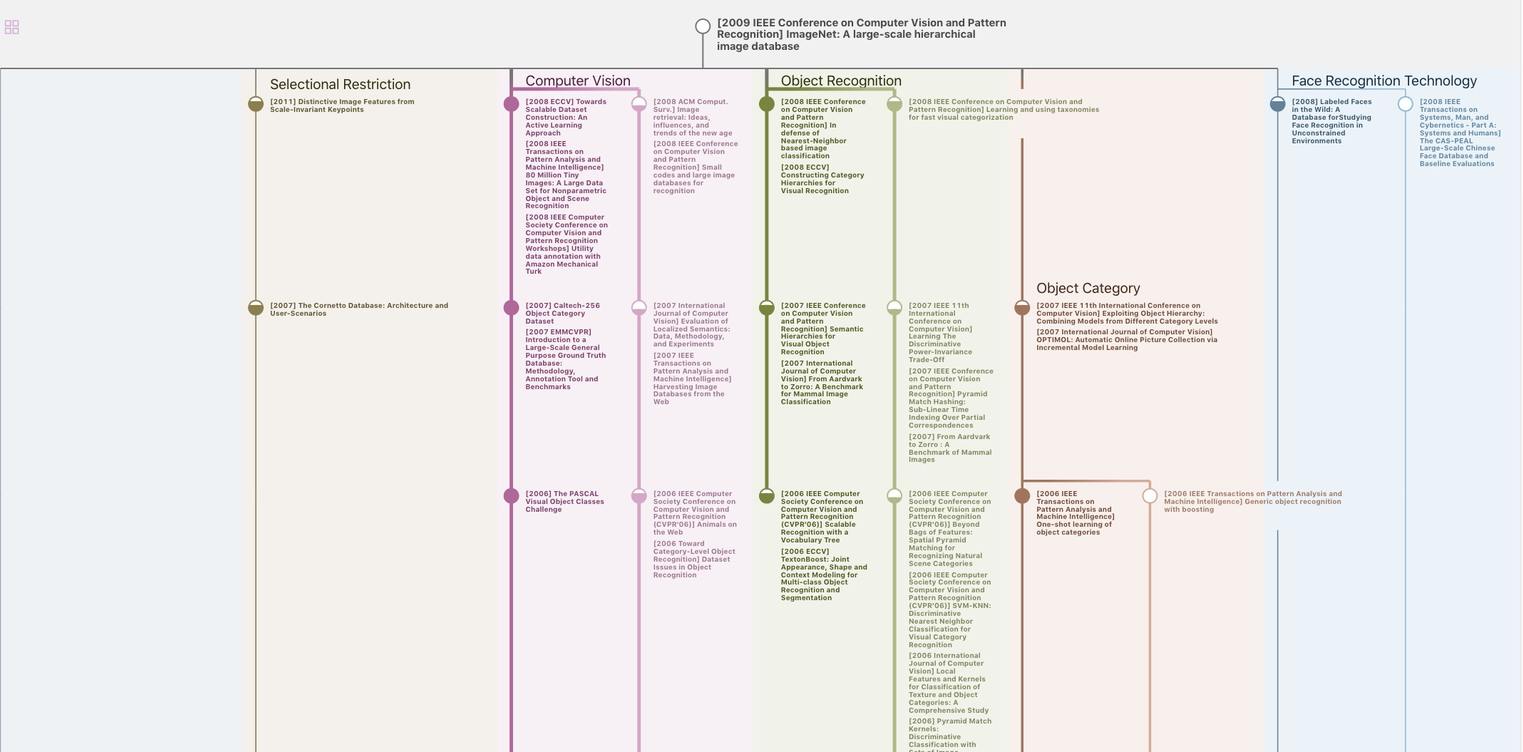
生成溯源树,研究论文发展脉络
Chat Paper
正在生成论文摘要