A novel machine learning tool for current and future flood susceptibility mapping by integrating remote sensing and geographic information systems
JOURNAL OF HYDROLOGY(2024)
摘要
Flood mapping is essential for managing and mitigating the risks associated with flood events. This study integrates weighted overlay analysis and analytical network process to create a flood susceptibility map. Twelve factors were selected to assess flood risk in the region, and an analytical network process was used to derive weights of factors. Weighted overlay analysis was then used to apply weights to the flood conditioning factors to prepare the flood susceptibility map. The most prominent historical flood extent was derived by using Landsat 8, Sentinel-2 and Sentinel-1 satellite imageries, spectral indices and decision tree model to generate a flood inventory map. The flood susceptibility map was assessed for accuracy using the flood inventory map, achieving an overall accuracy rate of 88.3 %. The primary factors affecting flood risk were elevation, slope, and distance from the stream, with high-risk areas mainly located downstream. To develop the flood risk assessment model, the essential factors were classified into four main categories: topographical, environmental, hydrological, and anthropological. All possible input combinations were checked using the outlier robust extreme learning machine for one to twelve input variables, and the best model was selected using the outlier robust extreme learning machine with the input combination of five variables (i.e., normalizes difference vegetation index, geology, precipitation, elevation, and slope). Based on the Sixth Intergovernmental Panel on climate change report, the developed outlier robust extreme learning machine was used to project potential flood areas in the next 78 years. Based on the different scenarios, the results indicated that 10.93 %, 8.55 %, 8.09 %, 11.99 %, and 16.28 % of all areas are projected to be highly and very highly flood susceptible. The tools and methods outlined in this study can assist decision-makers in preparing for and responding to floods. Moreover, the method developed in this study can be implemented in watersheds and urban areas and help manage flood risk in urban drainage systems.
更多查看译文
关键词
Analytical network process (ANP),Climate change,Flood susceptibility mapping,Machine learning,Outlier robust extreme learning machine,(ORELM),Remote sensing
AI 理解论文
溯源树
样例
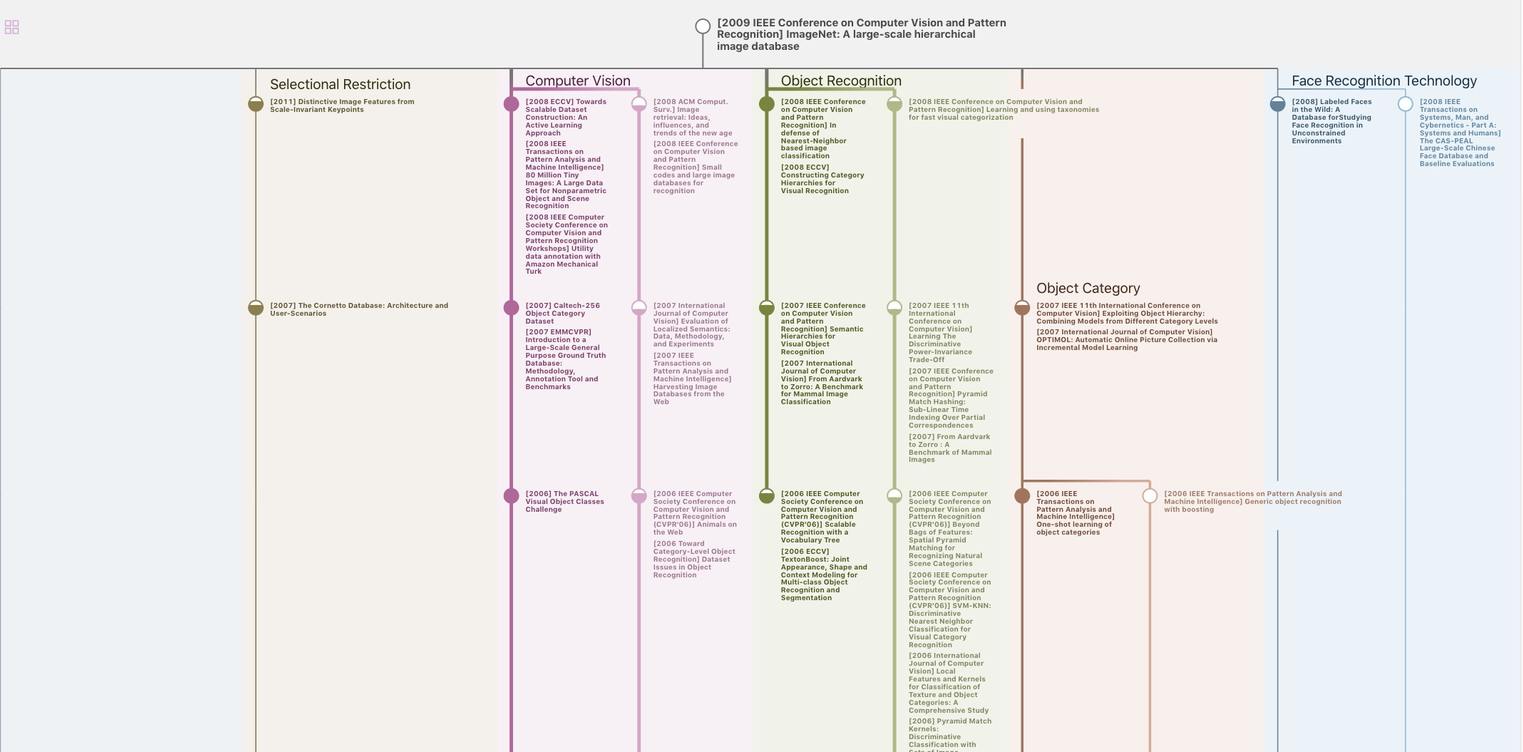
生成溯源树,研究论文发展脉络
Chat Paper
正在生成论文摘要