Probabilistic optimization based adaptive neural network for short-term wind power forecasting with climate uncertainty
INTERNATIONAL JOURNAL OF ELECTRICAL POWER & ENERGY SYSTEMS(2024)
摘要
Advanced wind power prediction technique plays an essential role in the stable operation of the grid with largescale grid integration of wind power. Most research focuses on distance-based static classification where the subjective nature of initial center selection increases the uncertainty of the prediction. And the data classification on a daily basis neglects the potentially significant climate changes at smaller time scales. To address these issues, the improved snake optimization-long short-term memory (ISO-LSTM) model with Gaussian mixture model (GMM) clustering is proposed to forecast wind power from an adaptive perspective. By exploiting the merits of the probabilistic classification, the K-means optimized GMM clustering enables an appropriate feature modelling for substantial climate changes at smaller time scales. Then the ISO algorithm exhibits higher search accuracy and is better suited for finding hyperparameter combinations for LSTM neural networks. The data from the National Aeronautics and Space Administration (NASA) of the US is used to validate the effectiveness of the proposed method. Compared to the traditional K-means clustering, the K-means optimized GMM clustering has increased accuracy by 2.63 %. Simultaneously, with the adoption of the enhanced ISO algorithm, the accuracy further increases by 7.27 %. Different existing models have also been tested; it shows that the proposed model demonstrates higher prediction accuracy.
更多查看译文
关键词
Probabilistic classification,Gaussian mixture model,K -means algorithm,Improved snake optimization,Long short-term memory network
AI 理解论文
溯源树
样例
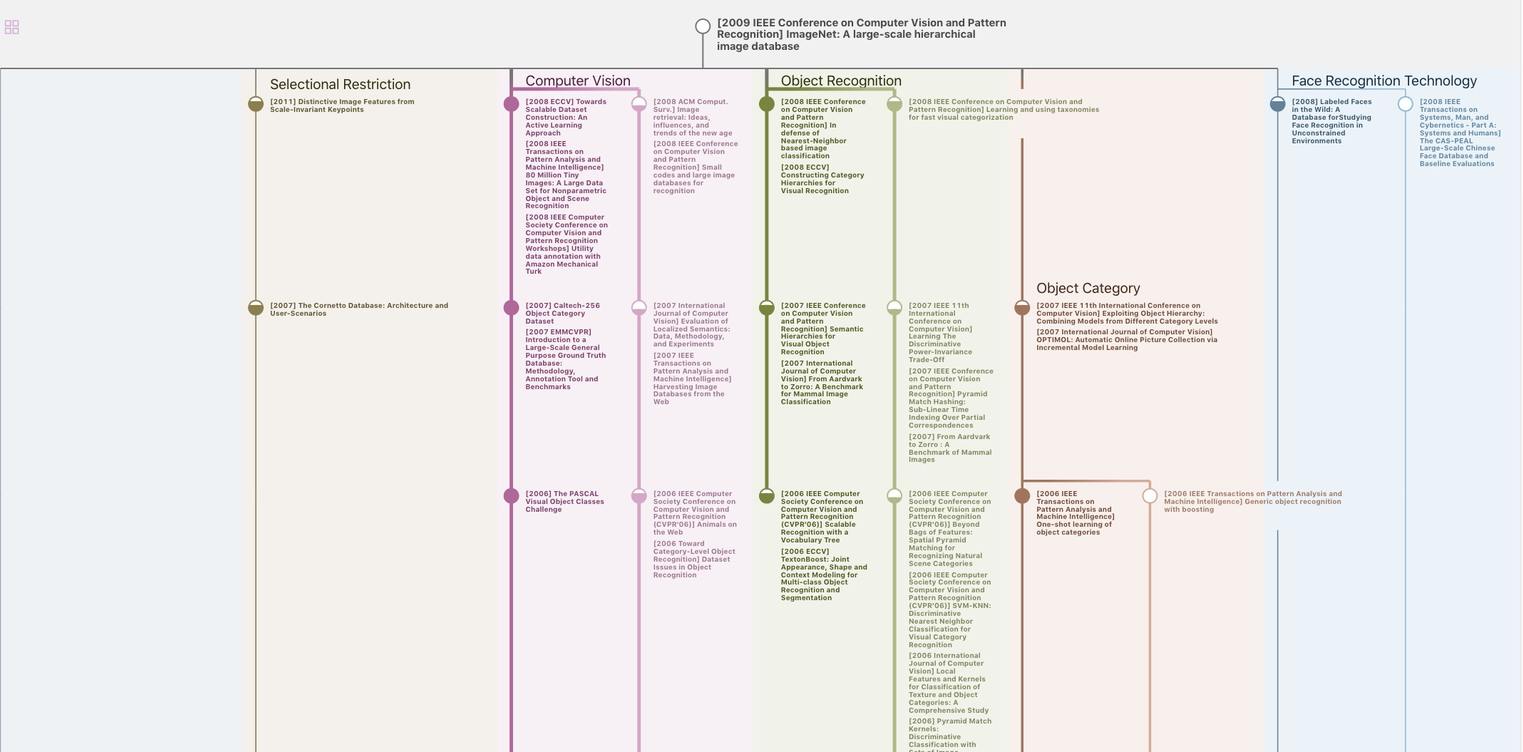
生成溯源树,研究论文发展脉络
Chat Paper
正在生成论文摘要