Digital twins based on machine learning for optimal control of chemical looping hydrogen generation processes
INTERNATIONAL JOURNAL OF HYDROGEN ENERGY(2024)
摘要
For a chemical looping hydrogen generation system, the set of input gas flow rate needed to be adjusted on the reactivity of oxygen carriers, in order to guarantee gas conversion and to obtain higher hydrogen purity. However, the oxygen carriers often decayed upon repeated cycles, leading to pressing need for dynamic alignment between oxygen carrier reactivity and process parameters. Herein, we propose a reaction optimization control method based on machine learning methods that can establish a real-time matching system between the flow rate of input gas and the reactivity of oxygen carriers. The results show that the prediction of hydrogen yield from oxygen carriers when using the optimized Gradient Boosting Decision Tree model is in general agreement with the experimental results (R2 = 0.87, MAE = 0.49). Based on high-precision prediction results, the method is capable of realizing the input gas flow based on the predicted hydrogen production in real time in less than 10s and realizing digital twin with the expansion of the dataset. We envision that the proposed control method can accelerate the industrial application of chemical looping hydrogen generation by predicting accurate operating parameters that can reduce fuel costs and guarantee reactivity of oxygen carriers.
更多查看译文
关键词
Machine learning,Oxygen carrier,Digital twin system,Chemical looping hydrogen generation
AI 理解论文
溯源树
样例
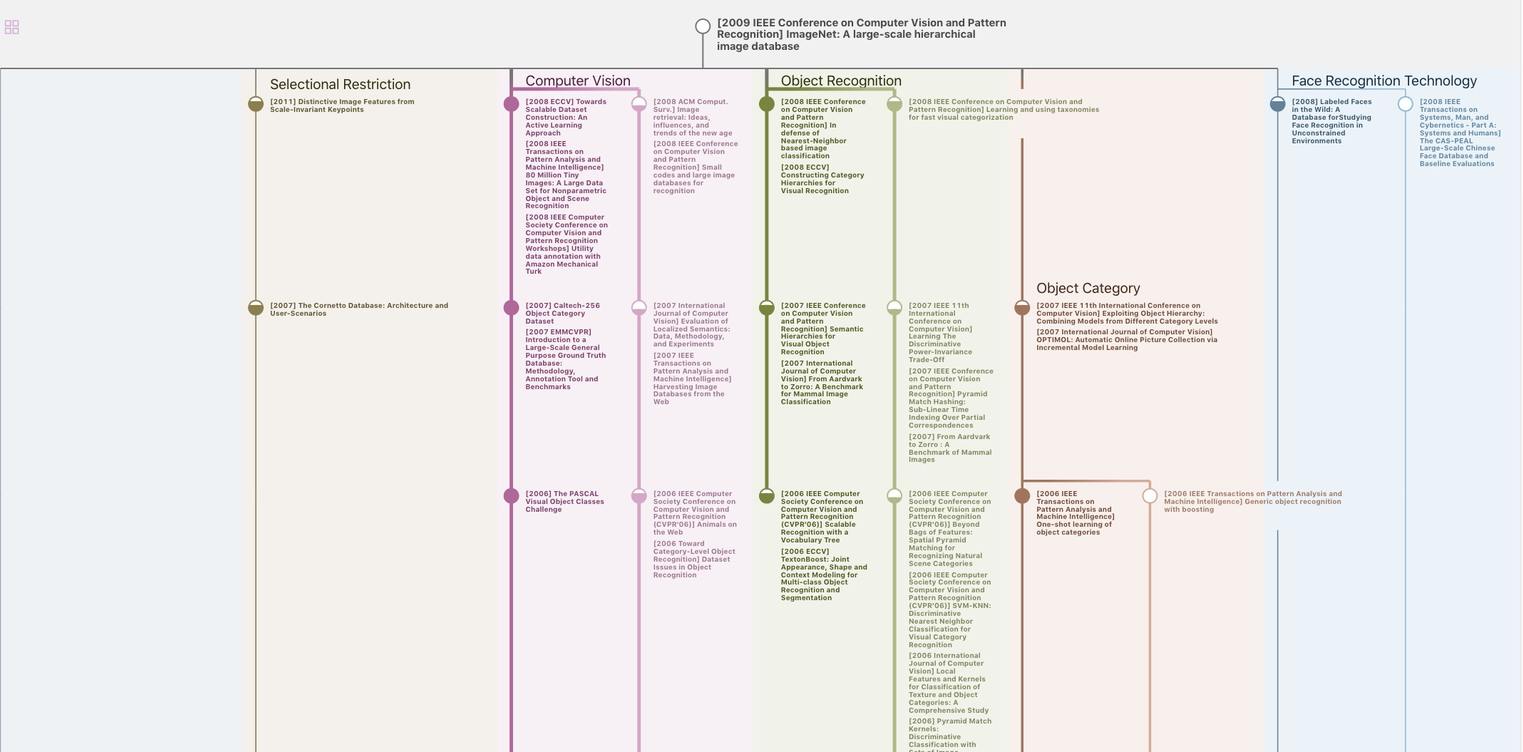
生成溯源树,研究论文发展脉络
Chat Paper
正在生成论文摘要