Cross-entropy based importance sampling for composite systems reliability evaluation with consideration of multivariate dependence
INTERNATIONAL JOURNAL OF ELECTRICAL POWER & ENERGY SYSTEMS(2024)
摘要
Multivariate kernel density estimation (KDE) is powerful for dependence modeling, but producing a non-sparse probability density estimation model. To address this issue, a sparse density estimation technique termed as generalized cross-entropy (GCE) density estimator, is proposed to estimate the joint probability density function (PDF) of correlated random variables (RVs). Moreover, to effectively accelerate the composite systems reliability evaluation, a unified cross-entropy based importance sampling (CEIS) method accommodating both GCE and KDE density estimators is presented, and a creative CE optimization approach is also developed based on the composition sampling algorithm, through which the analytical updating rules of IS-PDF parameters can be derived even though GCE and KDE estimators do not belong to the exponential distribution family (EF). Compared to the KDE estimator, the GCE estimator encounters much less dimensionality difficulty in CE optimization due to the model sparsity merit, and consequently achieves more accurate IS-PDF parameters and better CEIS efficiency. The superiority of GCE estimator over KDE estimator in correlation modeling and CEIS is verified by several numerical tests.
更多查看译文
关键词
Reliability evaluation,Kernel density estimation,Generalized cross -entropy,Sparsity,Importance sampling
AI 理解论文
溯源树
样例
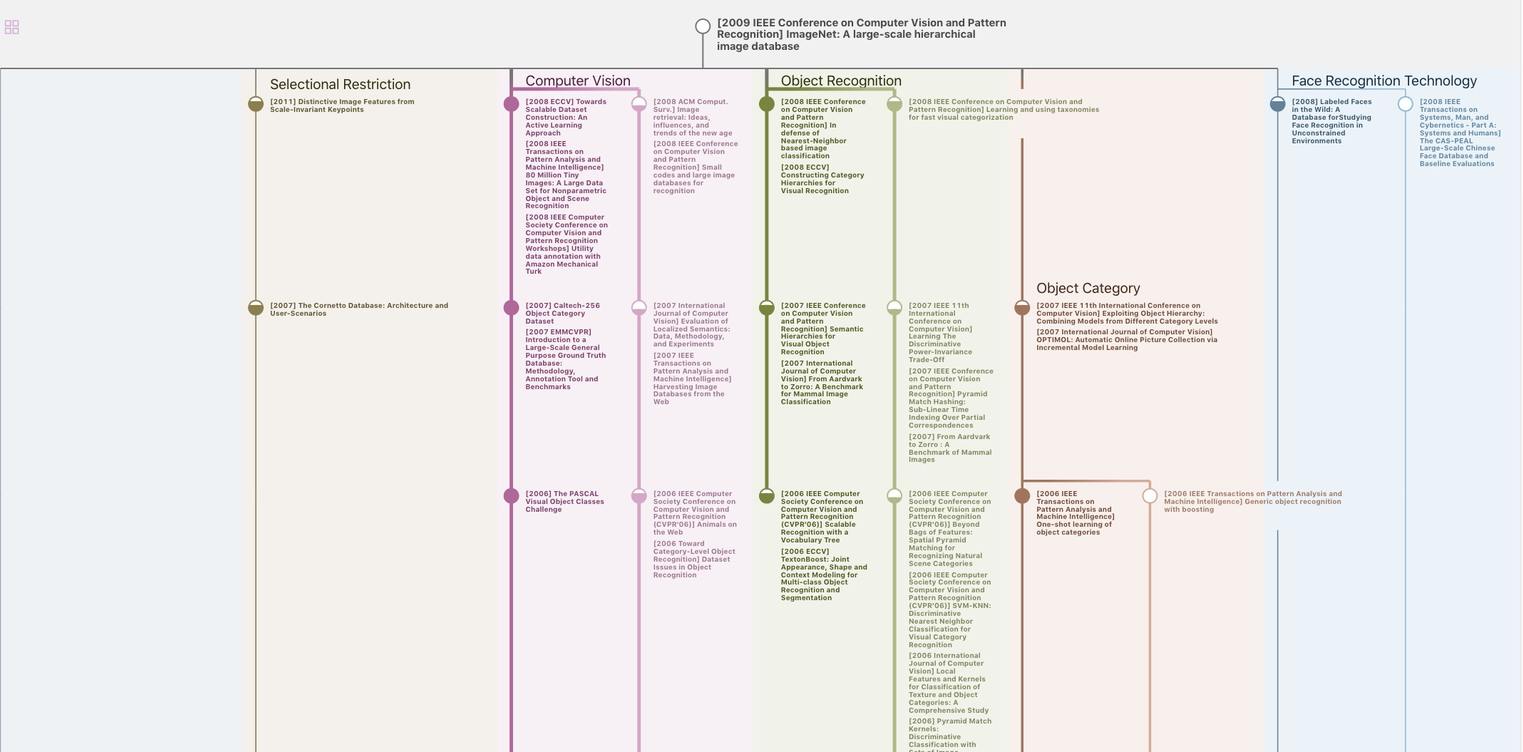
生成溯源树,研究论文发展脉络
Chat Paper
正在生成论文摘要