Ultra-early medical treatment-oriented system identification using High-Dimension Low-Sample-Size data
IFAC JOURNAL OF SYSTEMS AND CONTROL(2024)
摘要
Ultra-early detection of diseases with High-Dimension Low-Sample-Size (HDLSS) data has been effectively addressed by the Dynamical Network Biomarkers (DNBs) theory. After ultra-early detection, it is crucial to consider ultra-early medical treatment for the detected disease. From the viewpoint of control engineering, ultra-early medical treatment is achieved by increasing the system's stability and preventing the bifurcation, called re-stabilization. To implement effective re-stabilization, the system matrix is necessary. However, the available data in biological systems are often HDLSS, which is insufficient to identify the system matrix. In this paper, to realize HDLSS-based ultra-early medical treatment, we investigate an HDLSS data-based system matrix estimation method. First, HDLSS data is applied to compute the sample covariance matrix of the steady state. By assuming that the system matrix is sparse and the structure of the system matrix is known, it can utilize the Lyapunov equation to estimate the system matrix from the covariance matrix. The Lyapunov equation-based method gives a unique optimal estimation if the covariance matrix is full-rank. Otherwise, the optimal estimation is not unique. The sample covariance matrix computed from the HDLSS data is not full-rank. Thus, we apply shrinkage estimation to overcome the under-determined issue to obtain a well-conditioned covariance matrix with full rank. In addition, we confirm the effectiveness of the proposed method through numerical simulations. (c) 2024 The Author(s). Published by Elsevier Ltd. This is an open access article under the CC BY license (http://creativecommons.org/licenses/by/4.0/).
更多查看译文
关键词
Lyapunov equation,System identification,Computational statistics,Underdetermined system
AI 理解论文
溯源树
样例
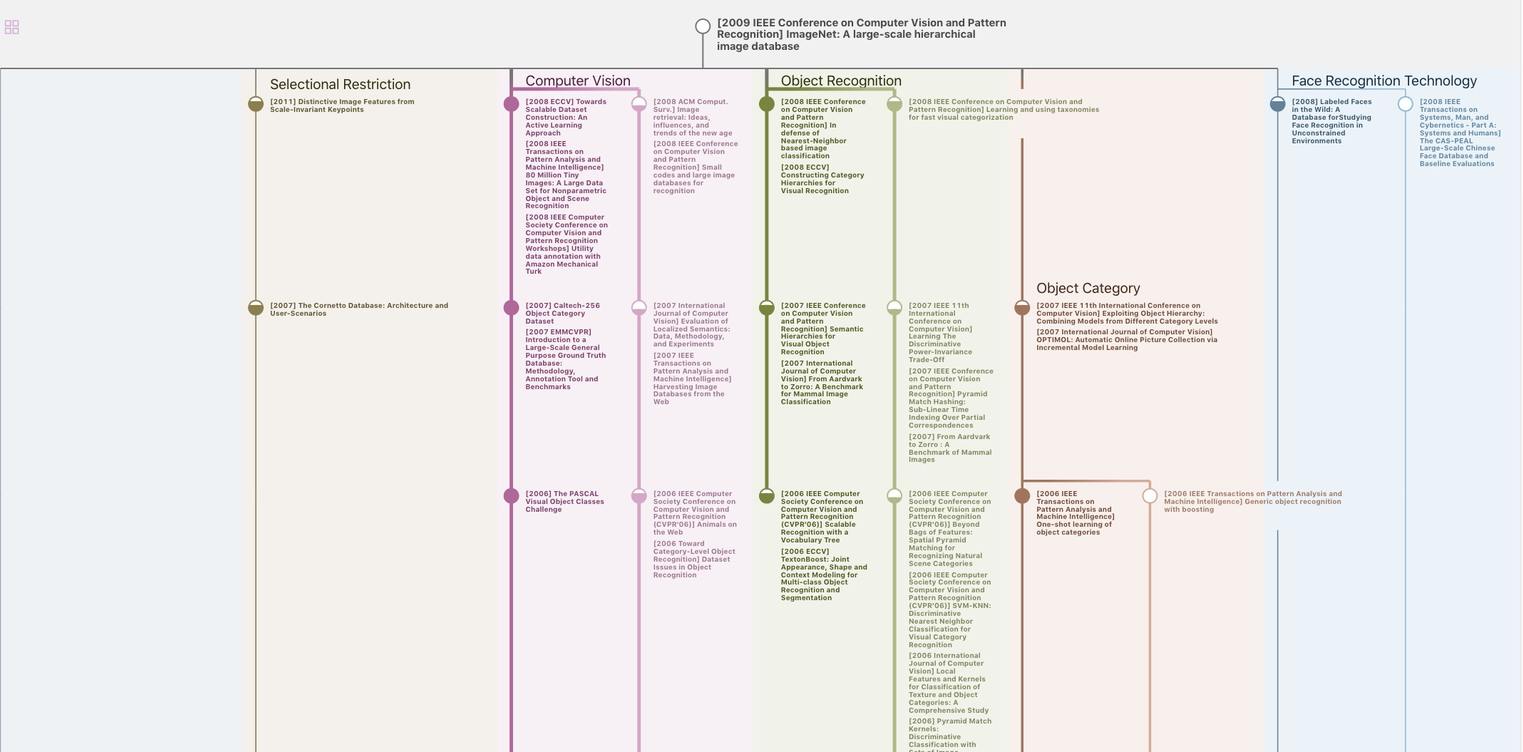
生成溯源树,研究论文发展脉络
Chat Paper
正在生成论文摘要