Electroencephalogram-based emotion recognition using factorization temporal separable convolution network
ENGINEERING APPLICATIONS OF ARTIFICIAL INTELLIGENCE(2024)
摘要
Temporal Convolutional Networks (TCNs) expand their receptive field through dilated convolutions, which is essential for capturing dependencies in longer sequences. This characteristic is especially critical for detecting long-term patterns in Electroencephalogram (EEG) data, making TCNs a suitable choice for EEG -based emotion recognition. In this study, to effectively capture the interaction between features, we incorporate Factorization Machines (FM) into the TCN model, proposing the Factorization Temporal Convolution Network (FTCN) for EEG -based emotion recognition. On one hand, the FTCN model enhances understanding of temporal dynamics by capturing long-term dependencies in time series data through TCN. On the other hand, it combines FM to increase the model's expression ability in the feature dimension, allowing for a more comprehensive understanding of EEG data. Building on this, separable convolutions are incorporated into the FTCN to develop the Factorization Temporal Separable Convolution Network (FTSCN). This approach reduces the model's parameter count by splitting standard convolutions into two simpler operations, thus accelerating training and inference. Experiments on the DEAP and SEED datasets demonstrate the effectiveness of these two models, showcasing competitive recognition accuracy compared to published methods. Specifically, on the DEAP dataset, the recognition accuracies for arousal and valence achieve 97.39% +/- 1.93 and 97.55% +/- 1.65. For the four -class task, the accuracy reaches 95.43% +/- 2.43 ; on the SEED dataset, the recognition accuracy can reach 89.13% +/- 4.49.
更多查看译文
关键词
Electroencephalogram,Emotion recognition,Temporal convolution network,Factorization machine
AI 理解论文
溯源树
样例
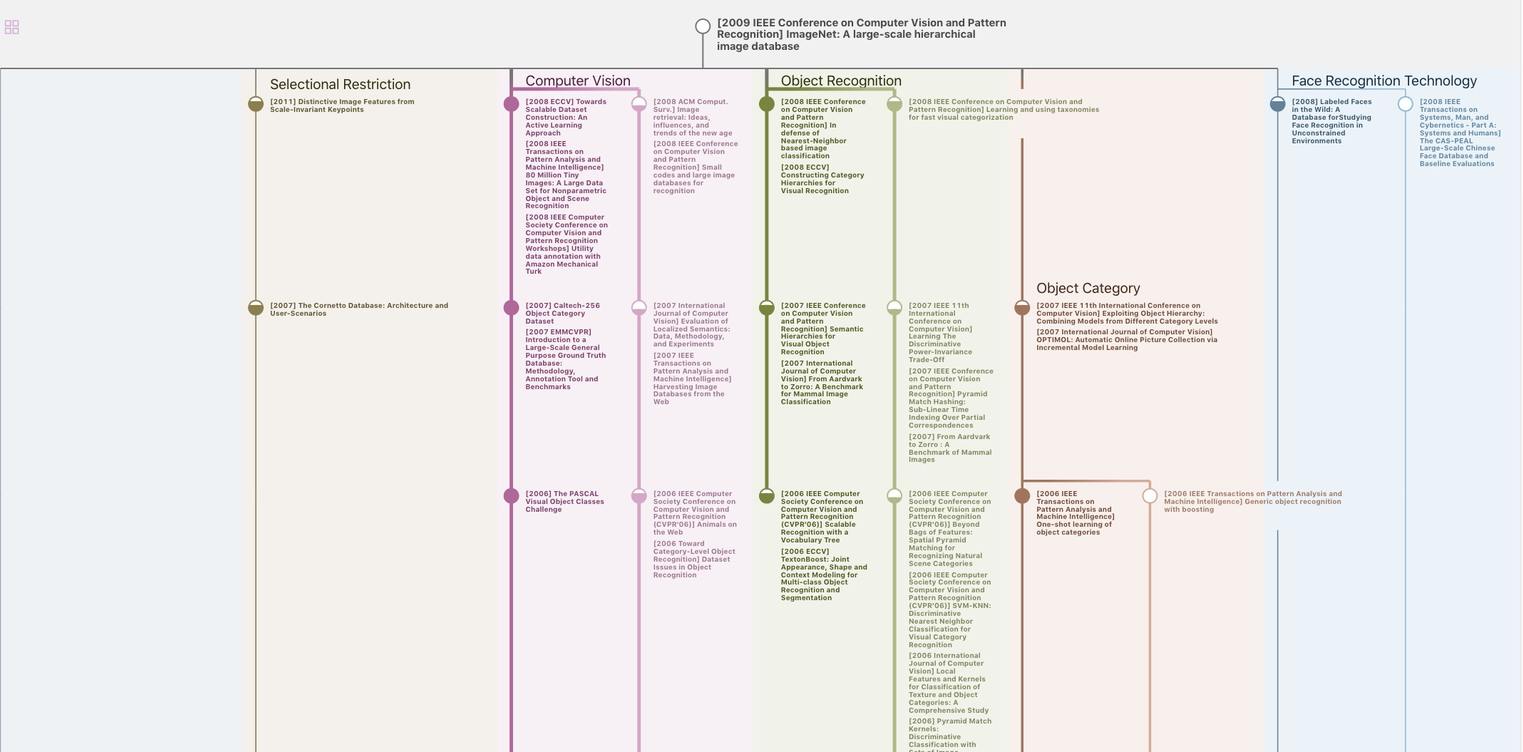
生成溯源树,研究论文发展脉络
Chat Paper
正在生成论文摘要