R2-trans: Fine-grained visual categorization with redundancy reduction
IMAGE AND VISION COMPUTING(2024)
摘要
Fine-grained visual categorization (FGVC) aims to discriminate similar subcategories, whose main challenge is the large intraclass diversities and subtle inter-class differences. Existing FGVC methods usually select discriminant regions found by a trained model, which is prone to neglect other potential discriminant information. On the other hand, the massive interactions between the sequence of image patches in ViT make the resulting class token contain lots of redundant information, which may also impact FGVC performance. In this paper, we present a novel approach for FGVC, which can simultaneously make use of partial yet sufficient discriminative information in environmental cues and also compress the redundant information in class-token with respect to the target. Specifically, our model calculates the ratio of high-weight regions in a batch, adaptively adjusts the masking threshold, and achieves moderate extraction of background information in the input space. Moreover, we also use the Information Bottleneck (IB) approach to guide our network to learn a minimum sufficient representations in the feature space. Experimental results on three widely-used benchmark datasets verify that our approach can achieve better performance than other state-of-the-art approaches and baseline models. The code of our model is available at: https://github.com/SYe-hub/R-2-Trans.
更多查看译文
关键词
Fine-grained visual categorization,Batch -based dynamic mask,Information bottleneck
AI 理解论文
溯源树
样例
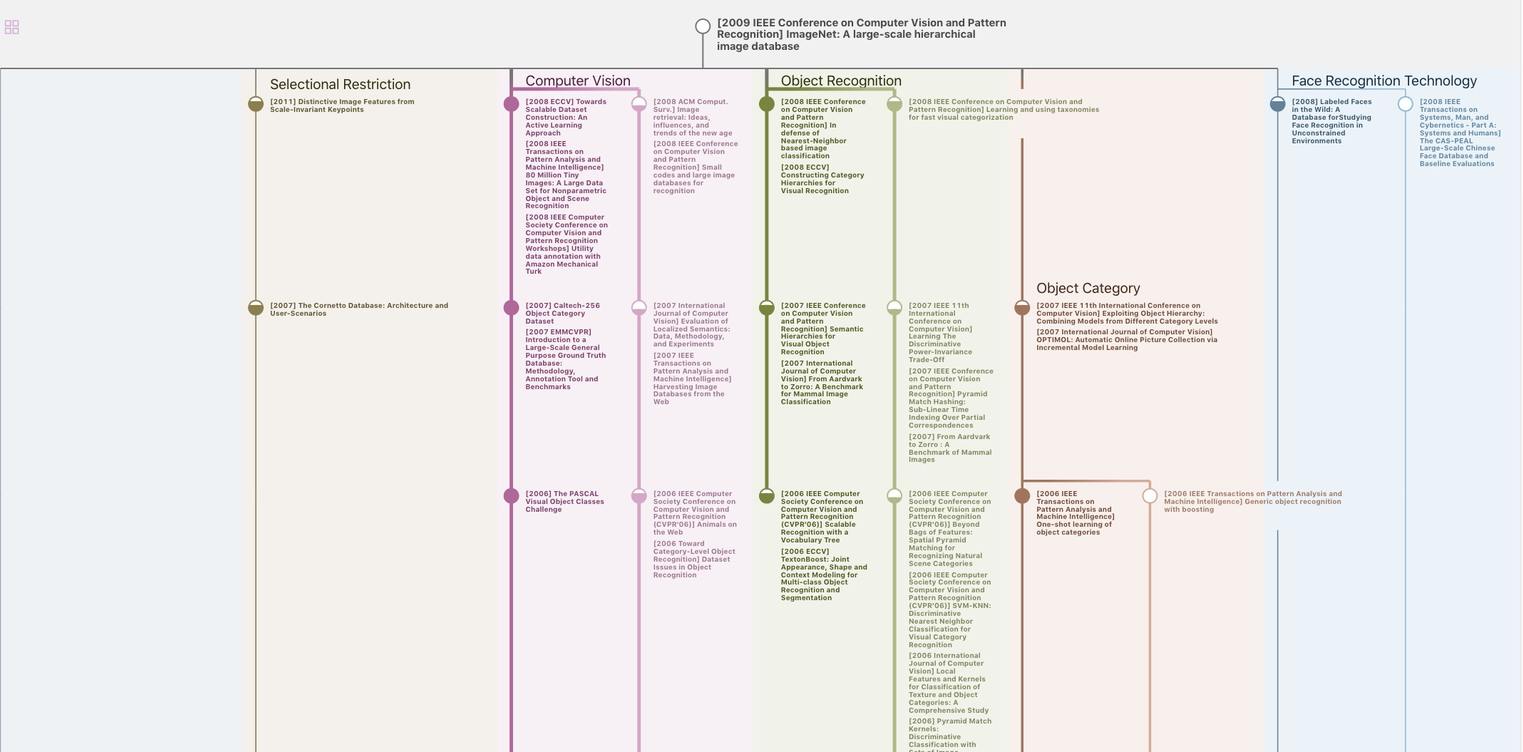
生成溯源树,研究论文发展脉络
Chat Paper
正在生成论文摘要