Exploring explainable artificial intelligence techniques for evaluating cervical intraepithelial neoplasia (CIN) diagnosis using colposcopy images
EXPERT SYSTEMS WITH APPLICATIONS(2024)
摘要
Although artificial intelligence techniques have performed well in analysing medical images, it is essential to consider the degree of understanding or interpretation in evaluations. This is especially important when diagnostic support is involved, as the trustworthiness of machine learning predictions needs to be clarified and evaluated. For deep learning algorithms to be translated into clinical practice, they must be made less opaque. To examine the classification of cervical dysplasia-diagnosed patients, we will explore explainable artificial intelligence (XAI) approaches, by implementing seven different interpretation techniques (Saliency, XRAI, Integrated Gradients, Smooth Gradients, Smooth Integrated Gradients, Grad-CAM, Smoothgrad-CAM). These XAI techniques shall be explored in order to assess the performance of four Convolutional Neural Network models (AlexNet, VGG16, MobileNet, and InceptionNet). Our results show that saliency qualities most closely match expert annotations, and there is a moderate to strong association between a model's sensitivity and the agreement between humans and computers. We also discovered a relevant relationship between expert insights and computational learning. This highlights the potential role of human expertise in influencing effective computer learning.
更多查看译文
关键词
Explainable Artificial Intelligence (XAI),Cervical intraepithelial neoplasia (CIN),Colposcopy,Cervigram images,Evaluation
AI 理解论文
溯源树
样例
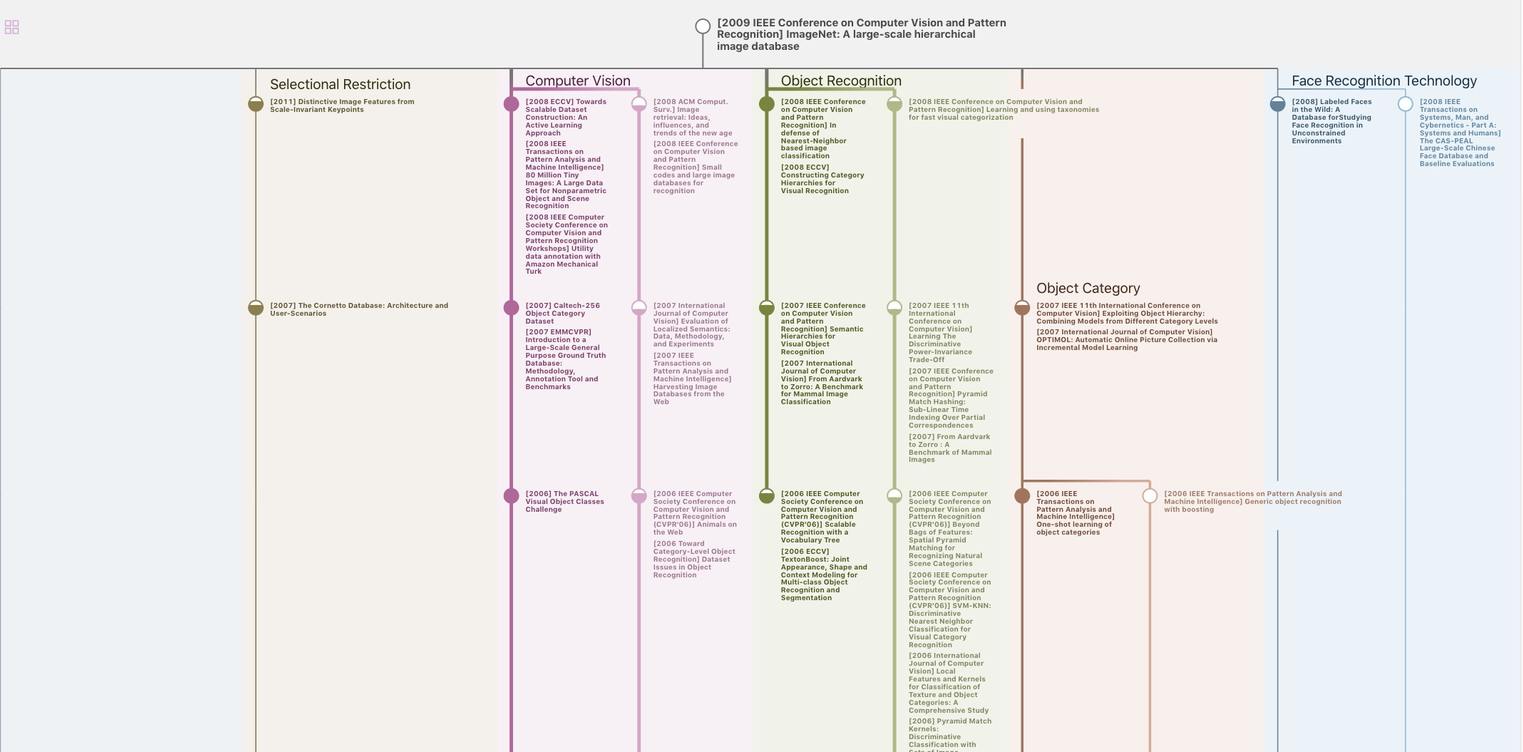
生成溯源树,研究论文发展脉络
Chat Paper
正在生成论文摘要