Explainable anomaly detection in spacecraft telemetry
ENGINEERING APPLICATIONS OF ARTIFICIAL INTELLIGENCE(2024)
摘要
As spacecraft missions become more complex and ambitious, it becomes increasingly important to track the status and health of the spacecraft in real-time to ensure mission success. Anomaly detection is a crucial part of spacecraft telemetry analysis, allowing engineers to quickly identify unexpected or abnormal behaviour reflected on spacecraft data and take appropriate corrective action. Traditional statistical methods based on threshold setting are often inadequate for detecting anomalies in this context, requiring the development of more sophisticated techniques that can handle the high -dimensional, non-linear, and non -stationary nature of spacecraft telemetry data such as machine learning -based techniques. This article presents an approach for anomaly detection using machine -learning techniques for spacecraft telemetry. The identification of anomaly types present on two real telemetry datasets from NASA is performed to incorporate information of magnitude, frequency, and waveform from known anomalies into the feature extraction process. Then, a machine -learningbased model is trained with the obtained features and tested with unknown real data. The proposed method achieves 95.3% of precision and 100% of Recall, giving a F05 score of 96.2% in both datasets, outperforming the metrics obtained on the existing related works, demonstrating that the inclusion of known anomalies can improve the performance of the data -driven models. Finally, an explainability analysis is performed to understand why a particular data instance has been identified as anomalous, proving the effectiveness of the feature extraction process.
更多查看译文
关键词
Anomaly detection,Spacecraft telemetry,Explainable machine learning,Time-series analysis
AI 理解论文
溯源树
样例
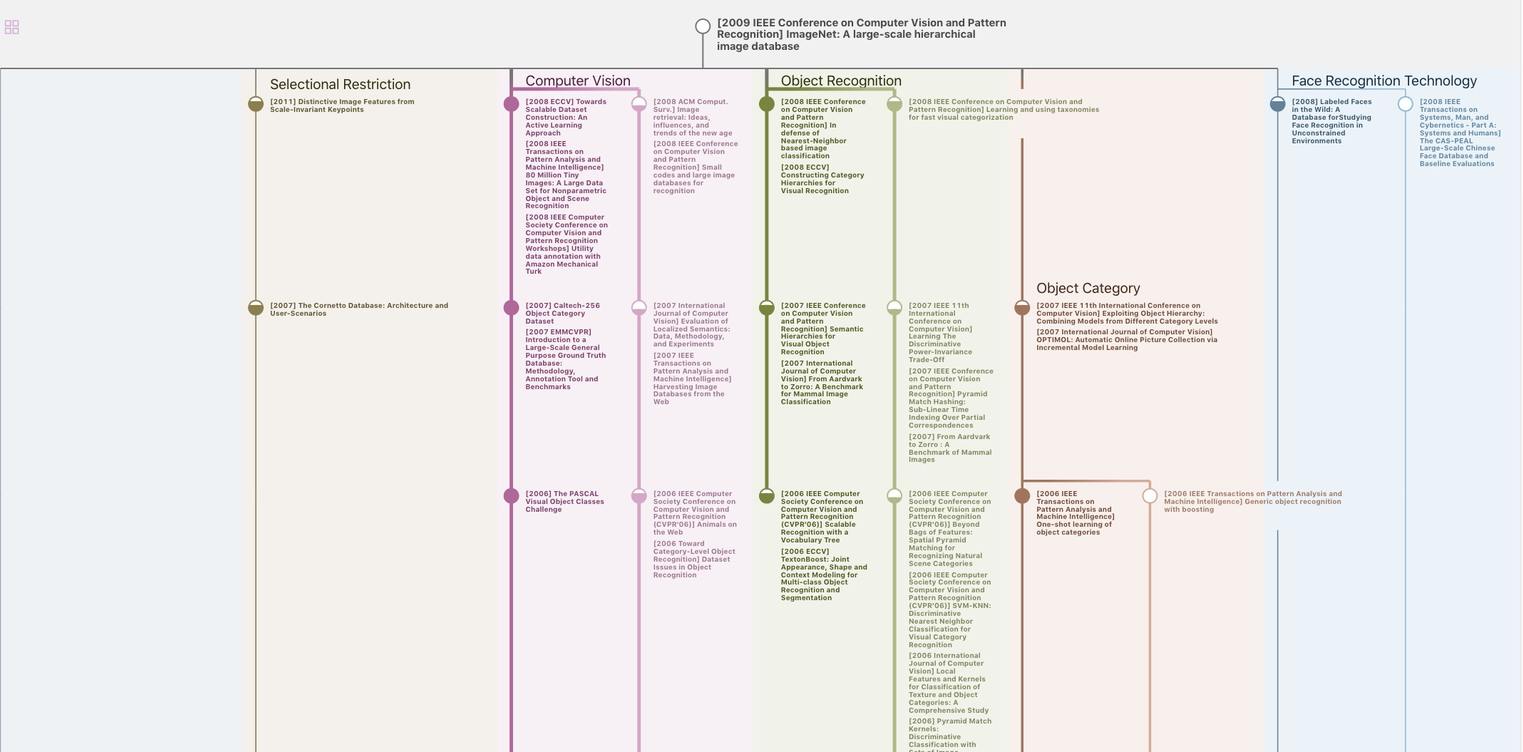
生成溯源树,研究论文发展脉络
Chat Paper
正在生成论文摘要