A generalized optimization-based generative adversarial network
EXPERT SYSTEMS WITH APPLICATIONS(2024)
摘要
Interest in Generative Adversarial Networks (GANs) continues to grow, with diverse GAN variations emerging for applications across various domains. However, substantial challenges persist in advancing GANs. Effective training of deep learning models, including GANs, heavily relies on well-defined loss functions. Specifically, establishing a logical and reciprocal connection between the training image and generator is crucial. In this context, we introduce a novel GAN loss function that employs the Sugeno complement concept to logically link the training image and generator. Our proposed loss function is a composition of logical elements, and we demonstrate through analytical analysis that it outperforms an existing loss function found in the literature. This superiority is further substantiated via comprehensive experiments, showcasing the loss function's ability to facilitate smooth convergence during training and effectively address mode collapse issues in GANs.
更多查看译文
关键词
Generative adversarial network,Loss function,Sugeno complement,Generative,Discriminative
AI 理解论文
溯源树
样例
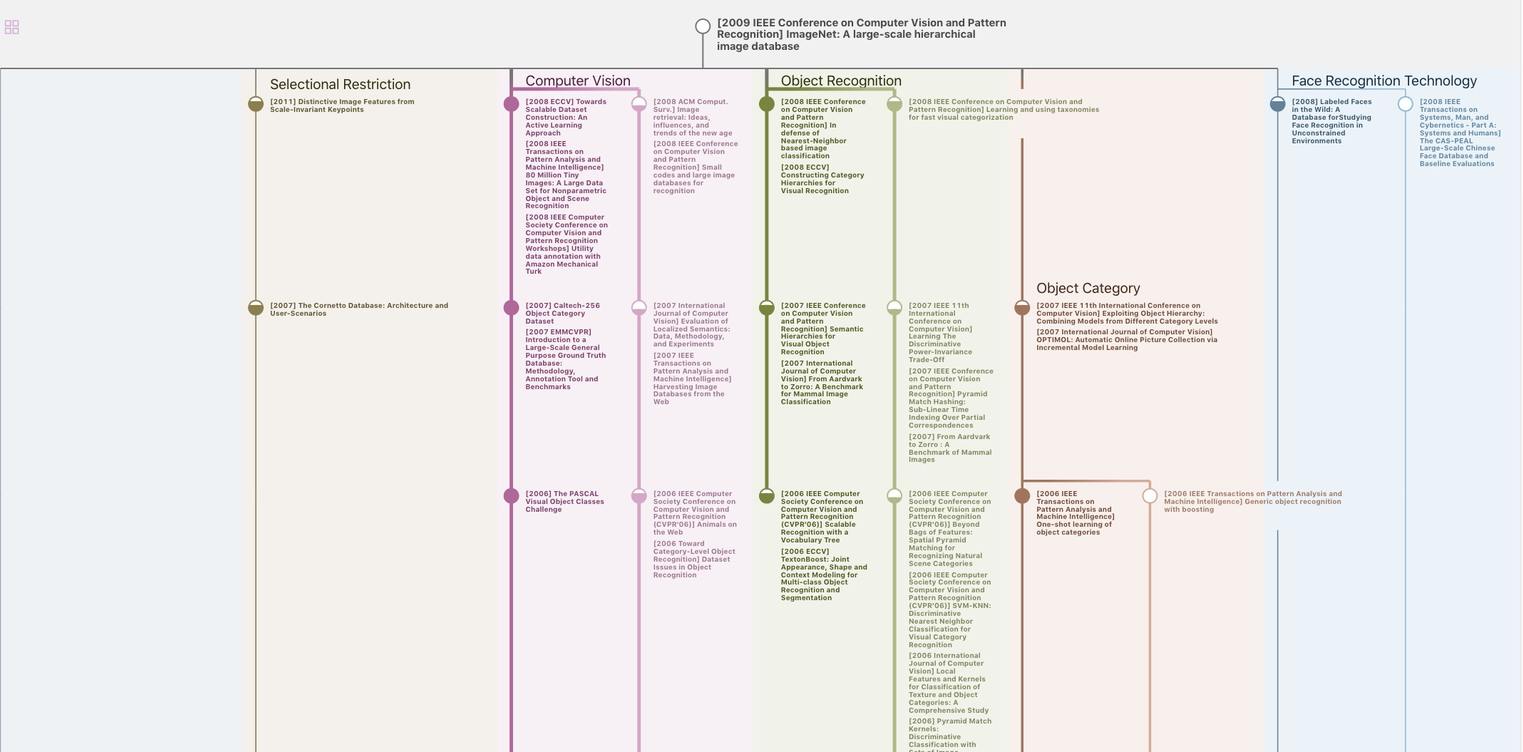
生成溯源树,研究论文发展脉络
Chat Paper
正在生成论文摘要