Incomplete multi-view clustering via local and global bagging of anchor graphs
EXPERT SYSTEMS WITH APPLICATIONS(2024)
Abstract
Anchor graphs are powerful tools for large-scale applications, but their quality depends on the selection of representative anchors, which is a difficult endeavor. Another challenge is to cluster data with incomplete multi -view features, which have missing values in some views. This paper proposes a novel method for incomplete multi -view clustering based on local and global bagging of anchor graphs. Our method does not require a specific anchor selection scheme but leverages bagging techniques to reduce the variance and discover the intrinsic anchor graph. Specifically, we first construct bagged partial anchor graphs for each incomplete view by randomly sampled anchors. Then, we integrate the bagged partial anchor graphs from multiple views into a unified and complete anchor graph. Finally, we apply global bagging to the unified anchor graph to further boost performance. Our method can generate robust anchor graphs for incomplete multi -view clustering scenarios, as evidenced by extensive experiments.
MoreTranslated text
Key words
Incomplete multi-view clustering,Anchor graph,Bagging,Ensemble learning,Spectral clustering
AI Read Science
Must-Reading Tree
Example
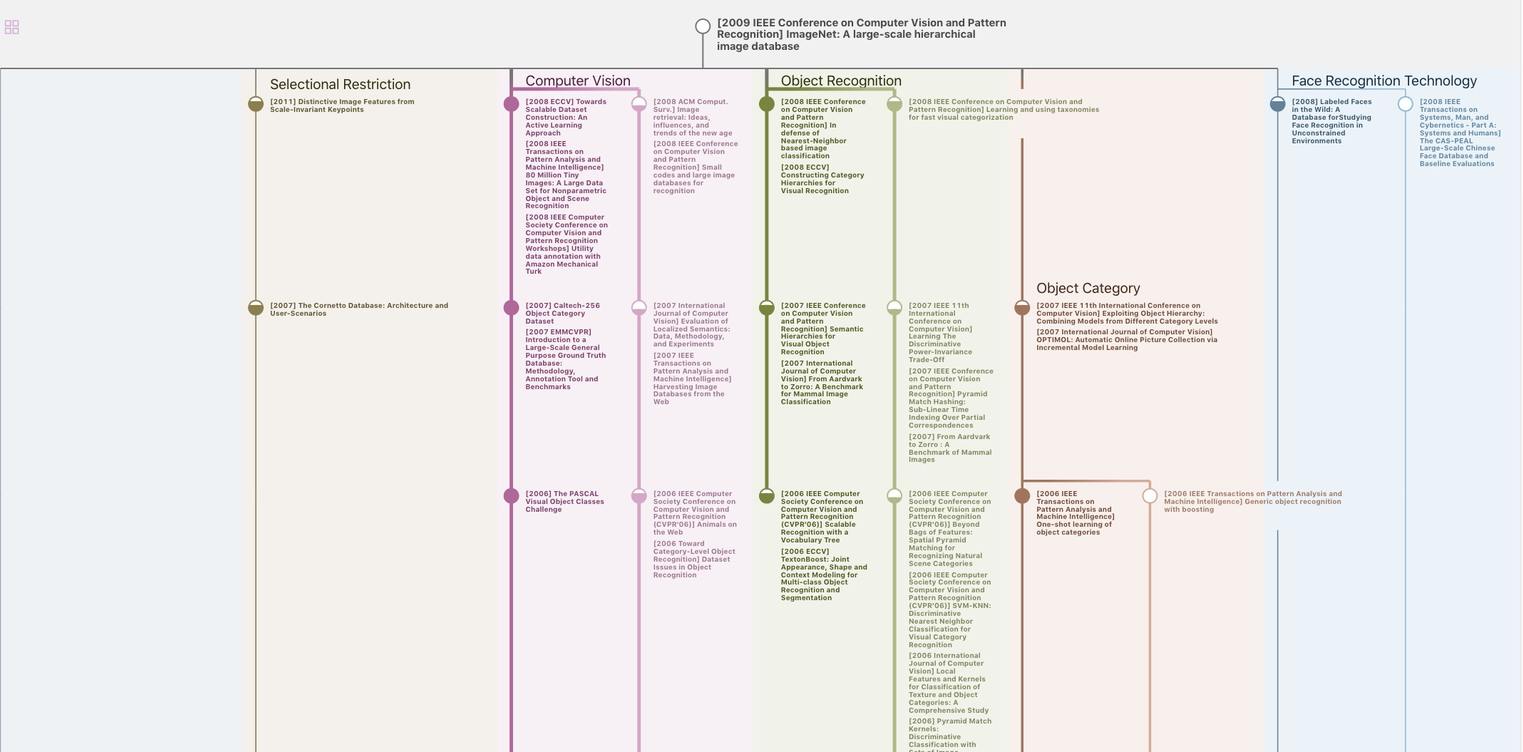
Generate MRT to find the research sequence of this paper
Chat Paper
Summary is being generated by the instructions you defined