Combination prediction of underground mine rock drilling time based on seasonal and trend decomposition using Loess
ENGINEERING APPLICATIONS OF ARTIFICIAL INTELLIGENCE(2024)
摘要
The rock drilling process is a critical component of underground mining, and its operation time is a crucial factor in mine planning and production scheduling optimization; consequently, it is essential to make an accurate prediction of rock drilling operation time using historical time series data. This study proposes a combination prediction model for underground mine rock drilling time based on Seasonal and Trend decomposition using Loess (STL). The STL model decomposes the historical time series data into the trend, seasonal, and random components, uses the Deep Belief Network - Extreme Learning Machine (DBN-ELM) model to predict the trend component, the Support Vector Regression (SVR) model to predict the seasonal component, and the historical mean value to predict the random component, and then overlays and reconstructs the prediction results of the three components to obtain the predicted values of the final working hours of rock drilling operations. The experimental results indicate that the Mean Absolute Percentage Error (MAPE) of the prediction result of the trend component with the highest weight proportion is 0.0159%, whereas the MAPE of the prediction result of the three components superposition reconstruction model is 0.2135%, representing a significant improvement in prediction accuracy compared to each comparison model and a broad range of practical applications.
更多查看译文
关键词
Underground mining,Rock drilling time,Time series prediction,STL,Deep learning
AI 理解论文
溯源树
样例
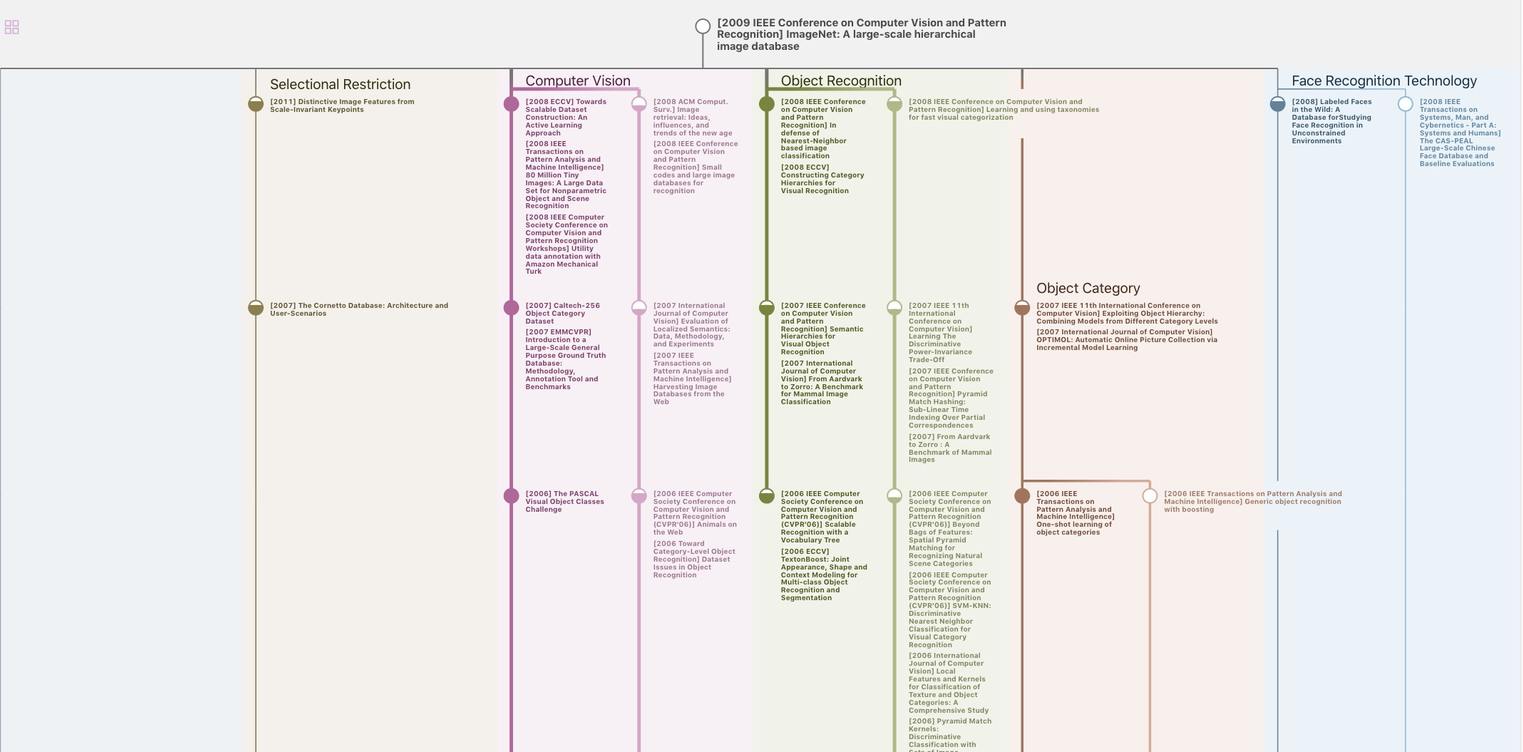
生成溯源树,研究论文发展脉络
Chat Paper
正在生成论文摘要