A multilevel interleaved group attention-based convolutional network for gas detection via an electronic nose system
ENGINEERING APPLICATIONS OF ARTIFICIAL INTELLIGENCE(2024)
Abstract
In this paper, an E-nose system for industrial exhaust detection is established and a novel deep learning model called the multilevel interleaved group attention-based convolutional network (MIGACN) is proposed for processing sensor array signals to identify 10 kinds of industrial pollution gases. First, a homemade E-nose system consisting of 15 gas sensors was constructed to acquire industrial pollution gas samples. Second, for sensor array signal processing, two novel feature learning modules are proposed at both the temporal level and the sensor level based on the actual physical significance of the sensor signals to enable the network to automatically extract intrinsic features of the sensor response signals. Third, we introduce a data augmentation module to avoid the problem of insufficient model training due to limited data volume and achieve dynamic gas detection through sliding windows. The proposed MIGACN directly uses the original response of the sensors as the input to automatically extract the intrinsic signal features without tedious manual empirical feature extraction. The experimental results show that the MIGACN achieves better classification performance and excellent stability than do other advanced deep learning methods. MIGACN obtains 90.76% classification accuracy without the data augmentation module. With the data augmentation module, the MIGACN obtains a testing accuracy of 98.06% from the early sensor response stage. In addition, the MIGACN achieves the highest average dynamic detection accuracy of 98.19% with the data augmentation module, which highlights its advantages in practical applications.
MoreTranslated text
Key words
Electronic nose,Interleaved group convolution,Temporal attention module,Channel attention module,Data augmentation,Industrial pollution gas
AI Read Science
Must-Reading Tree
Example
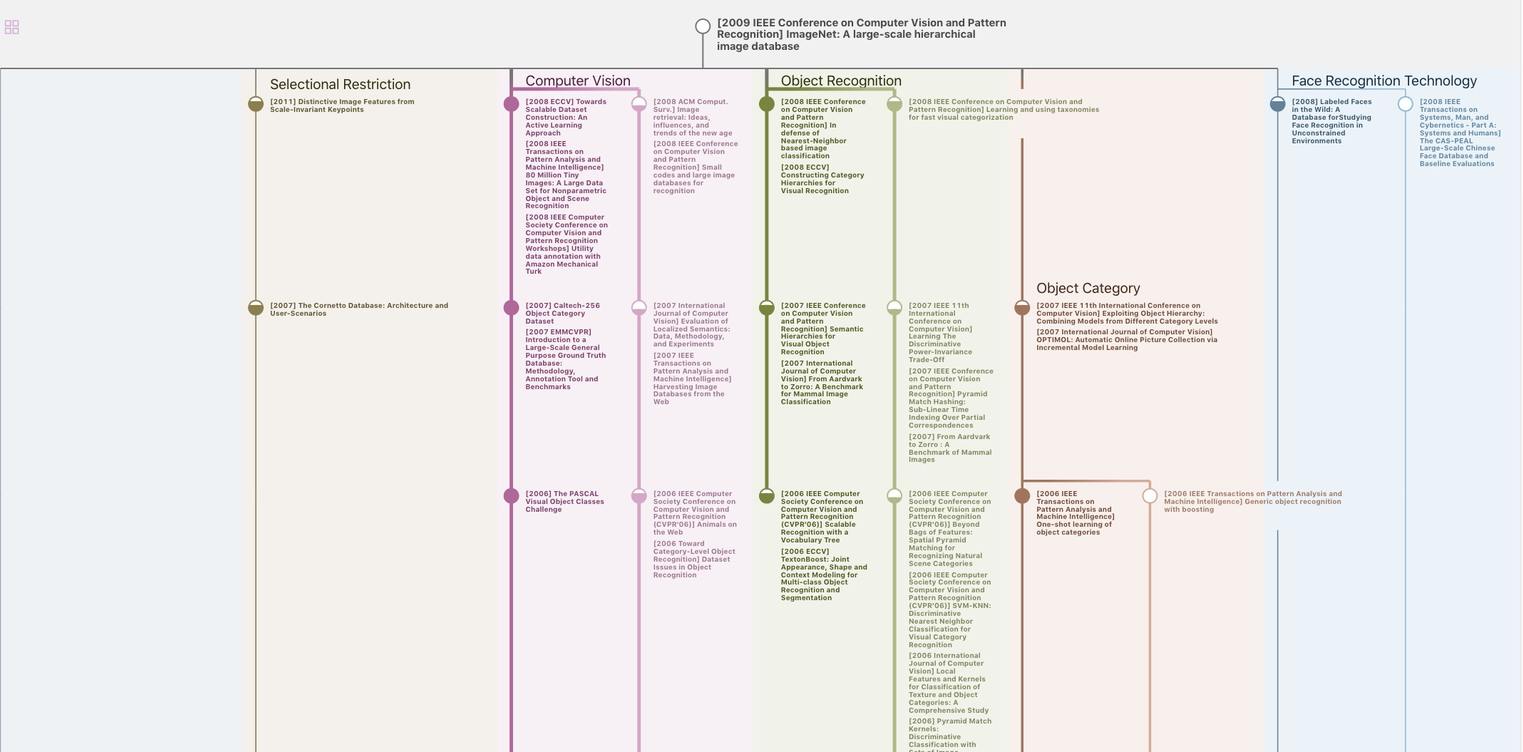
Generate MRT to find the research sequence of this paper
Chat Paper
Summary is being generated by the instructions you defined