VFL-Chain: Bulletproofing Federated Learning in the V2X environments
FUTURE GENERATION COMPUTER SYSTEMS-THE INTERNATIONAL JOURNAL OF ESCIENCE(2024)
摘要
Federated Learning (FL) has gained significant traction as a promising approach to enable collaborative machine learning (ML) while safeguarding data privacy across diverse applications, with the Vehicle -to -Everything (V2X) environment being a notable use case. However, conventional FL systems remain susceptible to model poisoning attacks, wherein malicious participants can introduce inaccurate or deliberately misleading local model updates to the central aggregator. In the context of V2X, such attacks could spawn catastrophic outcomes and jeopardize safety -critical applications. To tackle this pressing concern, we introduce a privacy -preserving and verifiable FL framework, dubbed VFL-Chain, designed to facilitate secure and efficient collaboration among intelligent connected vehicles (ICVs). VFL-Chain aspires to deliver a privacy -centric, verifiable FL experience complemented by a robust incentive mechanism tailored for ICVs. Our proposed solution leverages Bulletproofs to enable efficient verification of model update integrity, which are assimilated within smart contracts on an underlying permissioned blockchain. Furthermore, VFL-Chain presents a fair incentive mechanism that rewards honest participation, fostering a resilient and efficient FL implementation. We conduct an exhaustive security analysis and performance assessment of our proposed system, which underscores its efficacy in countering data poisoning attacks and augmenting the accuracy of FL.
更多查看译文
关键词
Federated Learning (FL),Vehicle-to-Everything (V2X),Bulletproofs,Blockchain,Verifiable computation,Incentive mechanism,Contract theory,Quantization
AI 理解论文
溯源树
样例
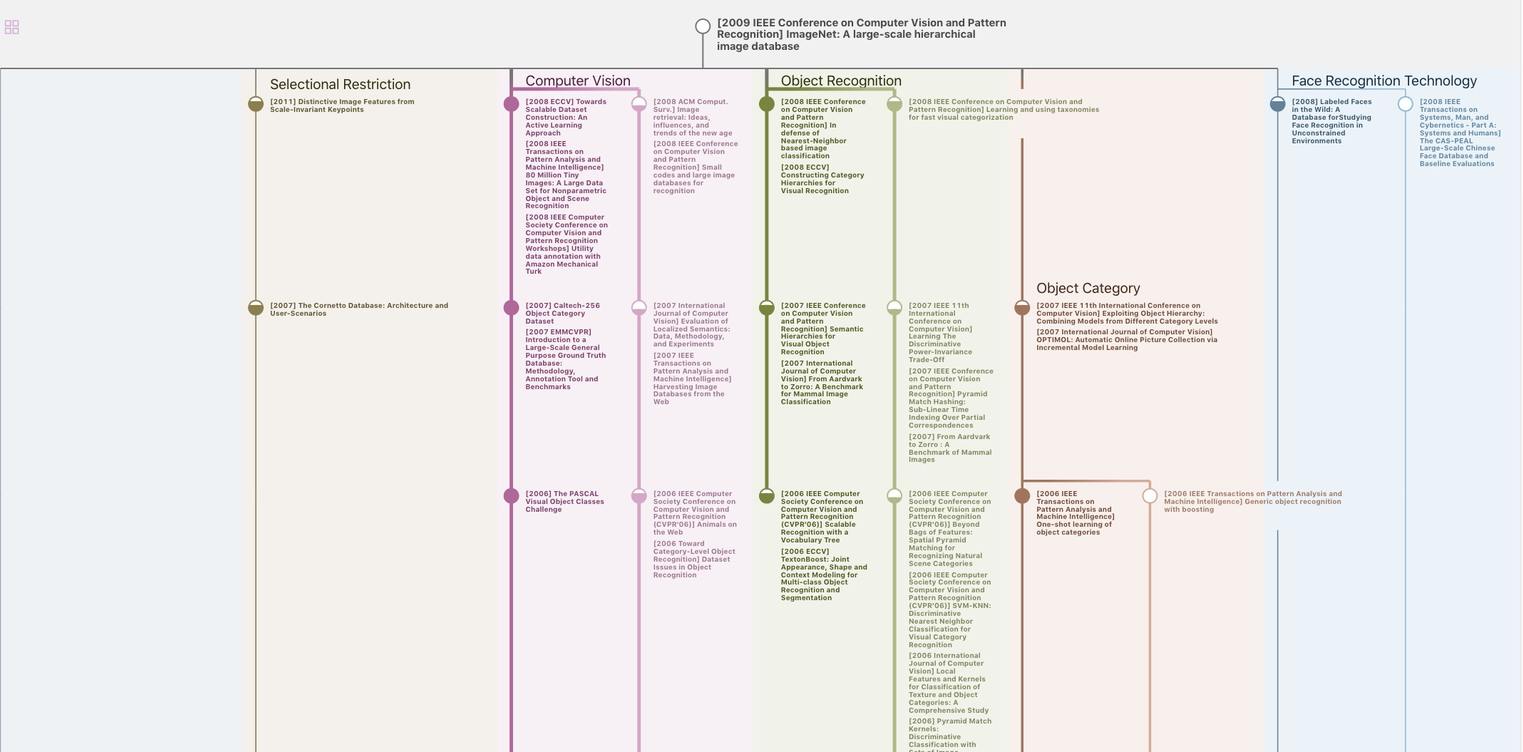
生成溯源树,研究论文发展脉络
Chat Paper
正在生成论文摘要