Similarity quantification of soil spatial variability between two cross-sections using auto-correlation functions
ENGINEERING GEOLOGY(2024)
摘要
In geotechnical engineering, an appreciation of local geological conditions from similar sites is beneficial and can support informed decision -making during site characterization. This practice is known as "site recognition", which necessitates a rational quantification of site similarity. This paper proposes a data -driven method to quantify the similarity between two cross -sections based on the spatial variability of one soil property from a spectral perspective. Bayesian compressive sensing (BCS) is first used to obtain the discrete cosine transform (DCT) spectrum for a cross-section. Then DCT-based auto -correlation function (ACF) is calculated based on the obtained DCT spectrum using a set of newly derived ACF calculation equations. The cross-sectional similarity is subsequently reformulated as the cosine similarity of DCT-based ACFs between cross -sections. In contrast to the existing methods, the proposed method explicitly takes soil property spatial variability into account in an innovative way. The challenges of sparse investigation data, non -stationary and anisotropic spatial variability, and inconsistent spatial dimensions of different cross -sections are tackled effectively. Both numerical examples and real data examples from New Zealand are provided for illustration. Results show that the proposed method can rationally quantify cross-sectional similarity and associated statistical uncertainty from sparse investigation data. The proposed method advances data -driven site characterization, a core application area in data -centric geotechnics.
更多查看译文
关键词
Geotechnical site investigation,Site similarity,Auto -correlation,Bayesian compressive sensing
AI 理解论文
溯源树
样例
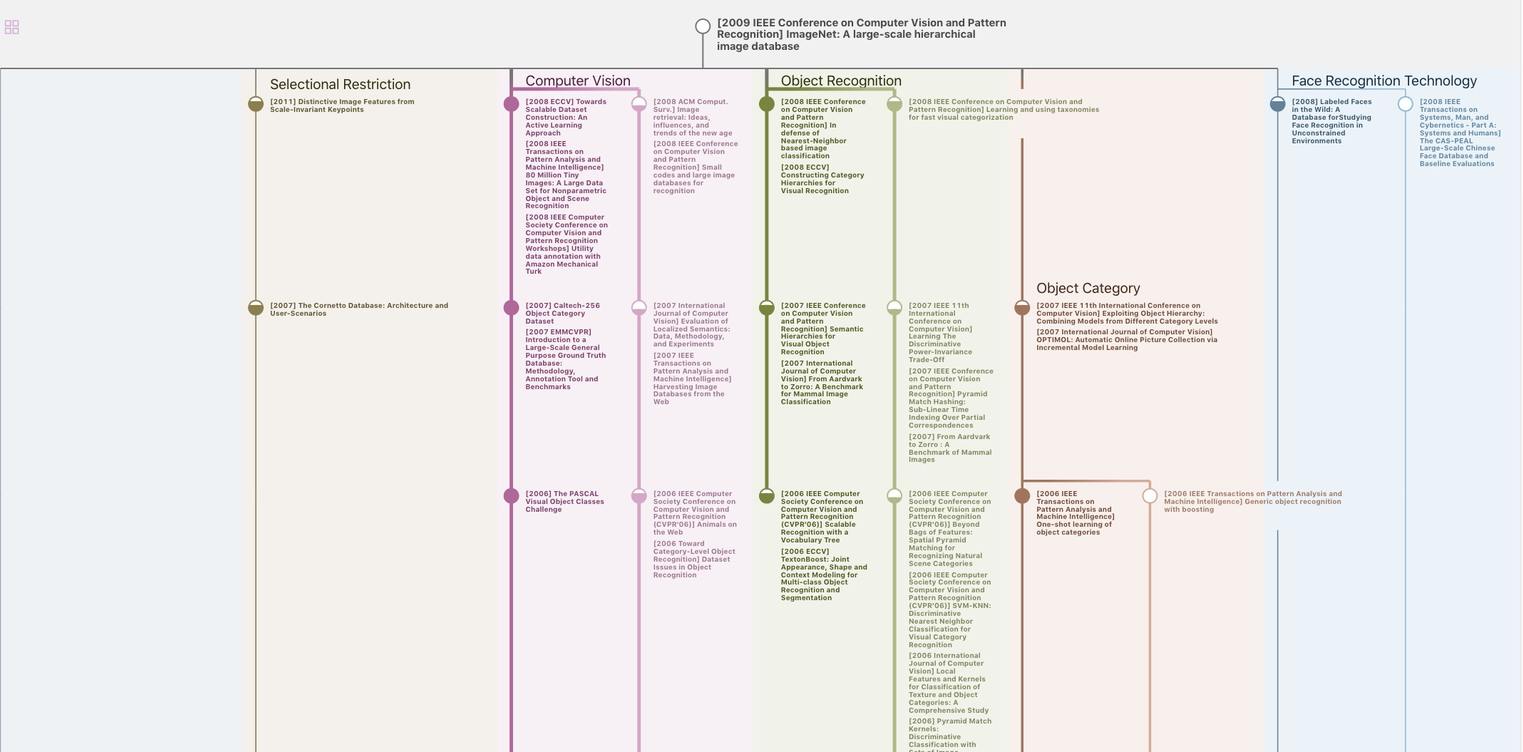
生成溯源树,研究论文发展脉络
Chat Paper
正在生成论文摘要