Modeling risk characterization networks for chemical processes based on multi-variate data
ENERGY(2024)
摘要
Risk characterization plays a crucial role in ensuring safe and stable chemical processes. However, existing studies cannot mine the deep-level relationships between multi-variate process data, resulting in un-detailed characterization results. Hence a multi-variate data-driven modeling method of risk characterization networks is proposed for chemical processes in this paper. The method particularly combines the Spearman's rank correlation coefficient and Apriori algorithm. Firstly, the Spearman's rank correlation coefficient is used to analyze the correlations between multi-variate process data. Secondly, the Apriori algorithm is further applied to mine the internal association rules of process data. Thirdly, a risk characterization network model is established using the complex network theory. To illustrate its validity, the Tennessee Eastman Process (TEP) and a catalytic cracking process are selected as test cases. Results show that the risk states of chemical processes can be effectively characterized, and the risk roots can also be reasonably identified and traced.
更多查看译文
关键词
Chemical processes,Risk characterization,Multi-variate data,Spearman 's rank correlation coefficient,Apriori algorithm,Complex networks
AI 理解论文
溯源树
样例
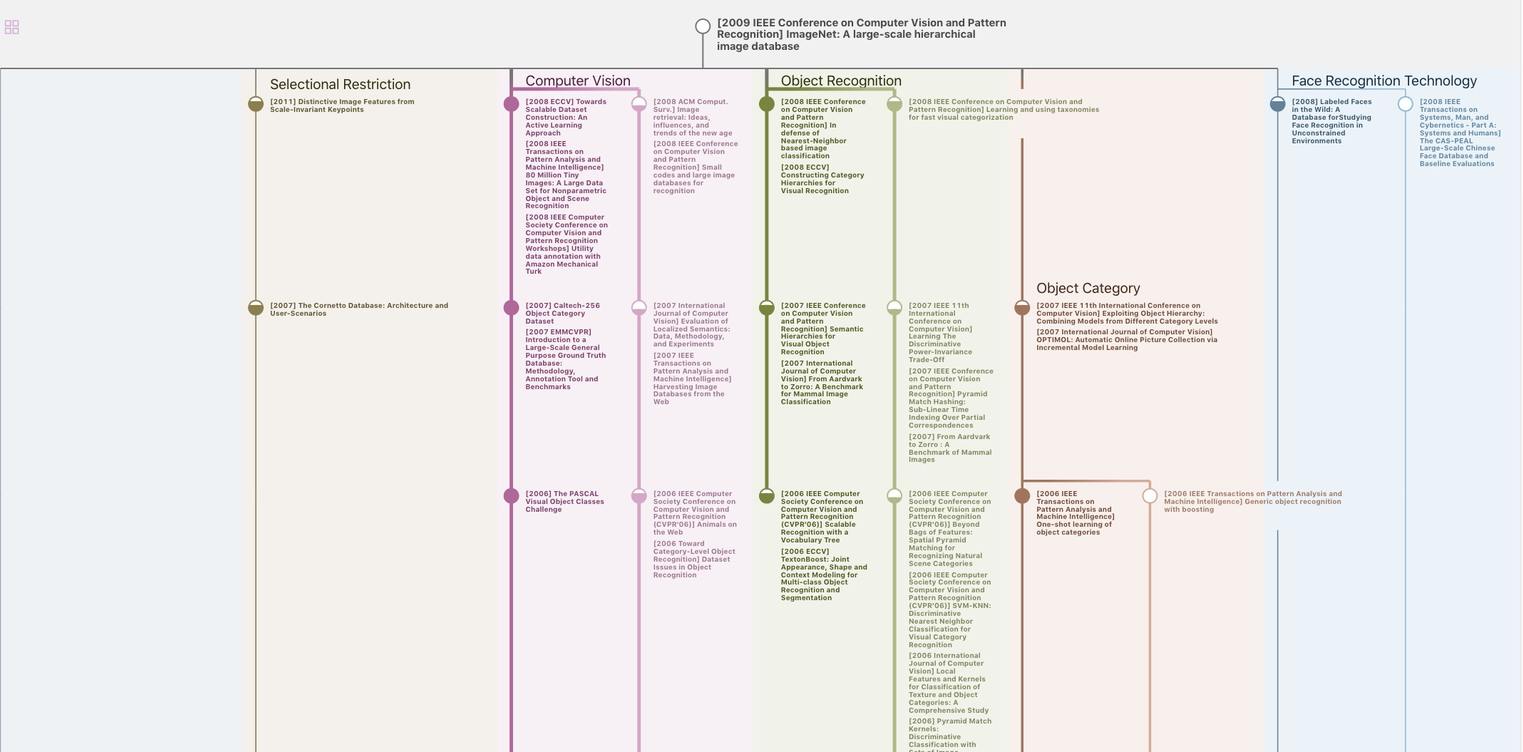
生成溯源树,研究论文发展脉络
Chat Paper
正在生成论文摘要