WildARe-YOLO: A lightweight and efficient wild animal recognition model
ECOLOGICAL INFORMATICS(2024)
摘要
For the protection of endangered species and successful wildlife population monitoring, wild animal recognition is essential. While deep learning models like YOLOv5 have shown promise in real-time object recognition, their practical applicability may be constrained by their high processing requirements. In this paper, we suggest a faster and lighter version of YOLOv5s for wild animal recognition. To lower computational costs for model parameters and floating-point operations (FLOPs) for the backbone, our suggested model includes Mobile Bottleneck Block modules and an improved StemBlock. We also use Focal-EIoU as a loss function to gauge the accuracy of the predicted bounding boxes during inference and employ a BiFPN-based neck. We tested our technique on three datasets, including Wild Animal Facing Extinction, Fishmarket, and MS COCO 2017. Additionally, our technique is compared with state-of-the-art deep learning models, and from the baseline model we recorded a 17.65% increase in FPS, 28.55% model parameters reduction, and 50.92% in FLOPs reduction. Furthermore, our model has a faster model loading time, which is critical for deployment in remote areas. This enables real-time species recognition on basic hardware, aiding conservation efforts through rapid analysis. The model advances deep learning in ecology by balancing efficiency with performance.
更多查看译文
关键词
Wild animal recognition,Deep learning,Lightweight,Efficient,Loss function
AI 理解论文
溯源树
样例
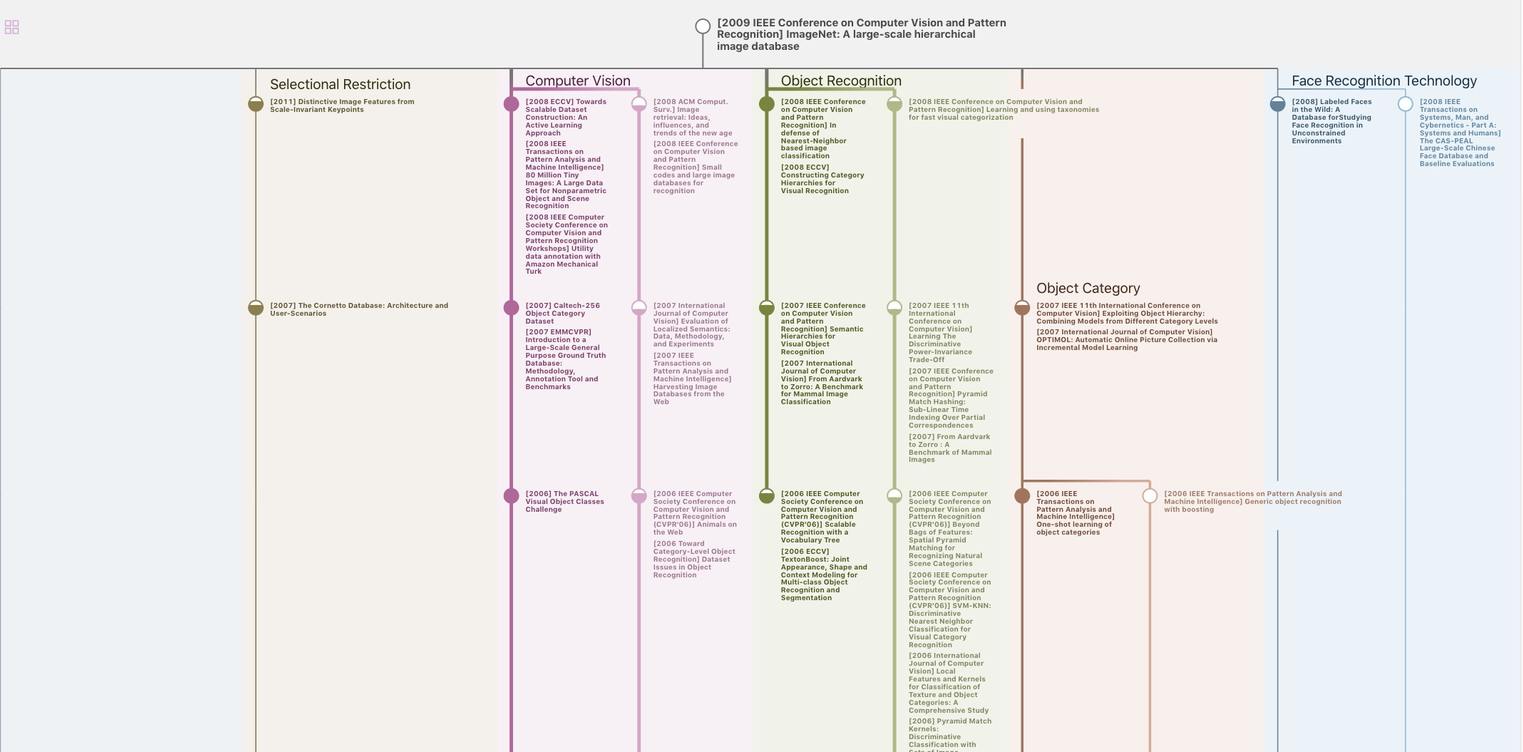
生成溯源树,研究论文发展脉络
Chat Paper
正在生成论文摘要