Fault classification and location of a PMU-equipped active distribution network using deep convolution neural network (CNN)
ELECTRIC POWER SYSTEMS RESEARCH(2024)
摘要
Accurate fault detection and localization play a pivotal role in the reliable and optimal operation of electric power distribution networks. However, the integration of intermittent distributed generation (DG) brings distinctive challenges to traditional fault diagnosis schemes, requiring more robust approaches. This paper proposes an intelligent and robust hierarchical framework for a fault diagnosis approach in power distribution grids integrated with intermittent DG. The approach employs deep convolutional neural networks (CNN), removing the need for hectic signal processing tools for feature extraction. The process starts with modeling a typical distribution network in the real-time digital simulator (RTDS) rack by incorporating the uncertainties of DG generation, load demand, and fault information through various probability density functions. Then, threephase current signal of two cycles (fault and pre-fault) from PMUs are collected by applying faults in different feeder locations. These generated signals are inputted into the proposed CNN models for fault classification, section identification, and localization. The method achieves an accuracy of 99.46% for fault classification, 99.39% for fault section identification, and an error of 0.9% for fault location. Furthermore, the extensive and comparative performance analysis with state-of-the-art fault diagnosis techniques reiterates the effectiveness of the proposed strategy in successful fault diagnosis.
更多查看译文
关键词
Convolutional neural network (CNN),Intermittency,Power distribution grid,Probability density functions (PDF),Distributed generation (DG),Real-time digital simulator (RTDS)
AI 理解论文
溯源树
样例
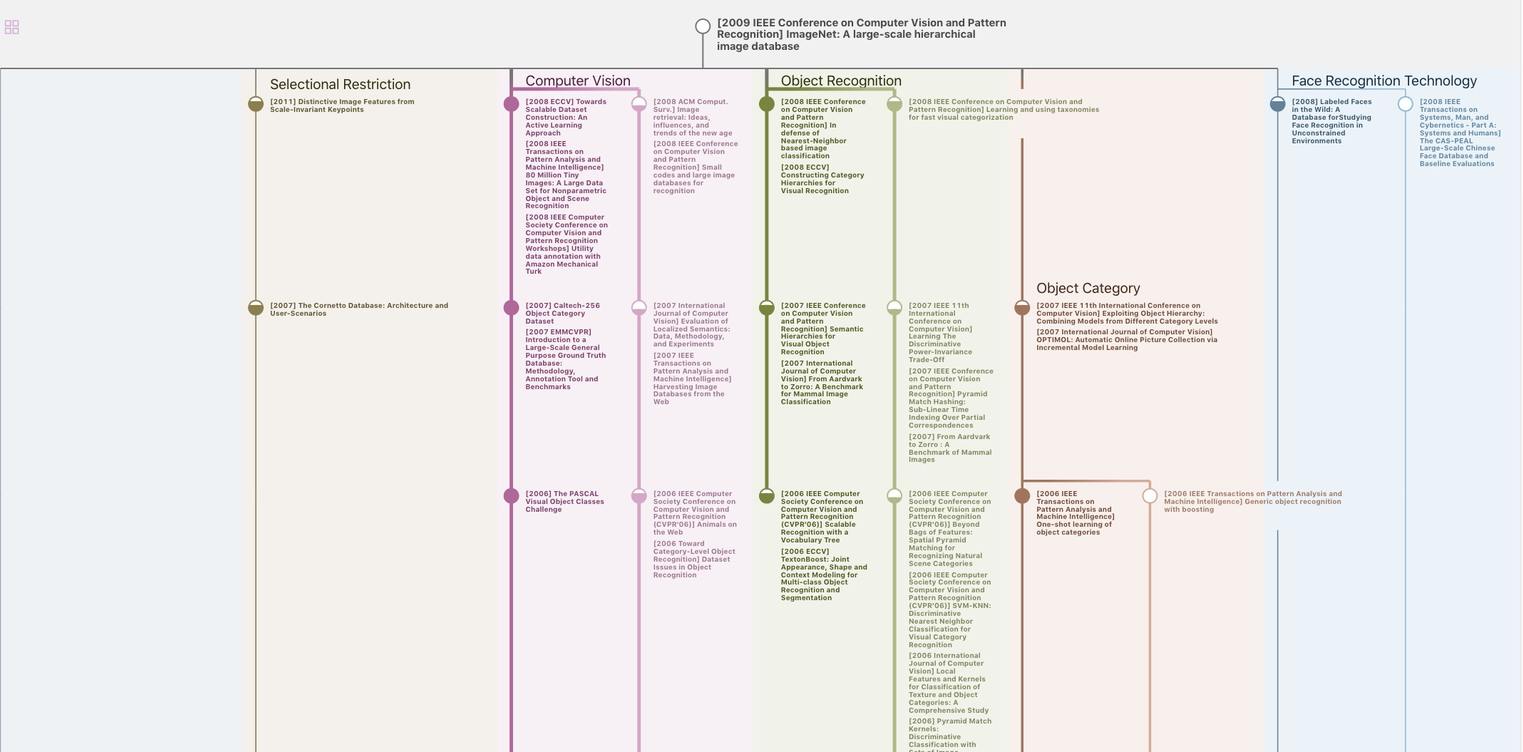
生成溯源树,研究论文发展脉络
Chat Paper
正在生成论文摘要