Exploring varying color spaces through representative forgery learning to improve deepfake detection
Digital Signal Processing(2024)
摘要
In the digital age, the rise of deepfake technology has brought unprecedented challenges to multimedia content authentication. The existing deepfake detection methods generally perform well in known settings. However, generalization and robustness are still challenging tasks. Observing that most conventional methods adopt the RGB color space, we introduce a novel deepfake detection approach by utilizing multiple color spaces to enhance the identification of deepfakes. Overall, our proposed detection framework comprises two primary stages, i.e., representative forgery learning through multi-color space reasoning and the color spaces-based forgery detection network (FDN). The representative forgery learning task is realized in succession through the manipulation cue boosting network (MCBN), color space transformations, and the forgery highlighting network (FHN). MCBN improves the feature representation, alternate color spaces provide distinctive advantages over traditional RGB color space, while FHN plays an auxiliary role, where it not only mines the texture inconsistency but also points out high-level semantic forgery clues, aiding in the robustness ability of FDN to discern subtle alterations in digital imagery accurately. Through rigorous evaluation on the benchmark datasets, including the FaceForensics++, DFDC, and CelebDF datasets, our proposed approach exhibits promising results in identifying forged multimedia content across varying color representations, outperforming the state-of-the-art methods.
更多查看译文
关键词
Deepfake detection,Color spaces,Forgery mining,Handcrafted cues image,Multimedia forensics,Color spaces-based forgery detection network
AI 理解论文
溯源树
样例
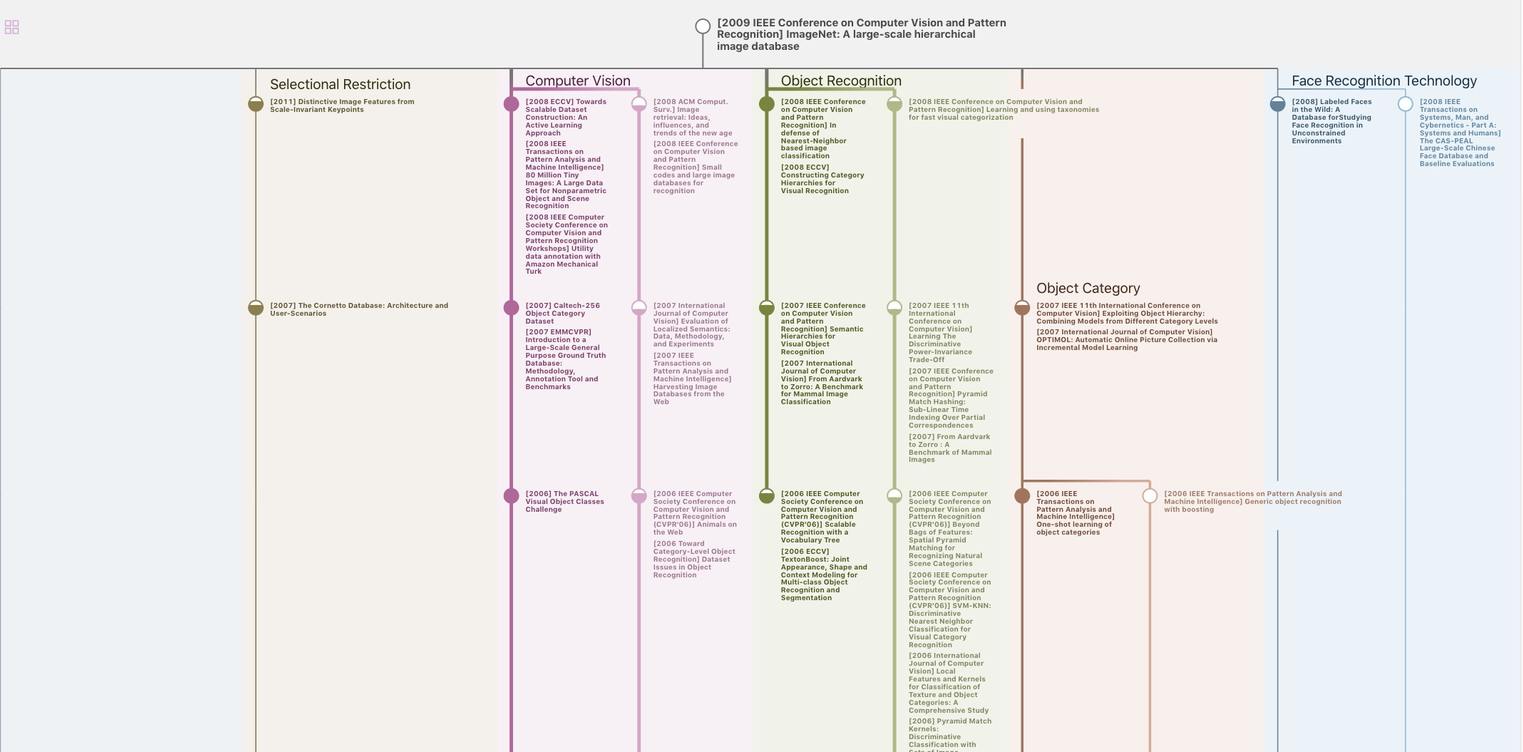
生成溯源树,研究论文发展脉络
Chat Paper
正在生成论文摘要