Archimedes optimisation algorithm quantum dilated convolutional neural network for road extraction in remote sensing images
HELIYON(2024)
Abstract
Roads are closely intertwined with human existence, and the process of extracting road networks has emerged as the most prominent task in remote sensing (RS). The automated road interpretation process of remote sensing images (RSI) efficiently acquires road network data at a reduced expense in comparison to the traditional visual interpretation of RSI. However the manifestation of RSI is completely distinct because of the great difference in length, width, material, and shape of road networks in dissimilar areas. Thus, the extraction of road network data in RSI is still a complex issue. In recent times, DL-based approaches have projected a famous development in image segmentation outcomes, but a lot of them still could not retain boundary data and attain high-resolution road segmentation maps while processing the RSI. Traditional convolutional neural networks (CNNs) demonstrate impressive performance in road extract tasks; however, they frequently encounter difficulties in capturing intricate details and contextual information. The study introduces a novel method, named Archimedes Optimisation Algorithm, Quantum Dilated Convolutional Neural Network for Road Extraction (AOA-QDCNNRE), to tackle the challenges encountered in remote sensing images. The AOA-QDCNNRE technique aims to generate a highresolution road segmentation map using DL with a hyperparameter tuning process. The AOAQDCNNRE technique primarily relies on the QDCNN model, which integrates quantum technology (QC) with dilated convolutions to augment the network's capacity to capture local as well as global contextual information. In addition, the incorporation of the dilated convolutional technique effectively enhances the receptive field without sacrificing spatial resolution, enabling the extraction of precise road features. To develop the road extraction outcomes of the QDCNN approach, the AOA-based hyperparameter tuning process can be exploited. The AOA-QDCNNRE system's simulation results can be tested on benchmark databases, and the results indicate that the AOA-QDCNNRE method surpasses recent algorithms.
MoreTranslated text
Key words
Artificial intelligence,Road extraction,Convolutional neural network,Remote sensing,Dilated convolution
AI Read Science
Must-Reading Tree
Example
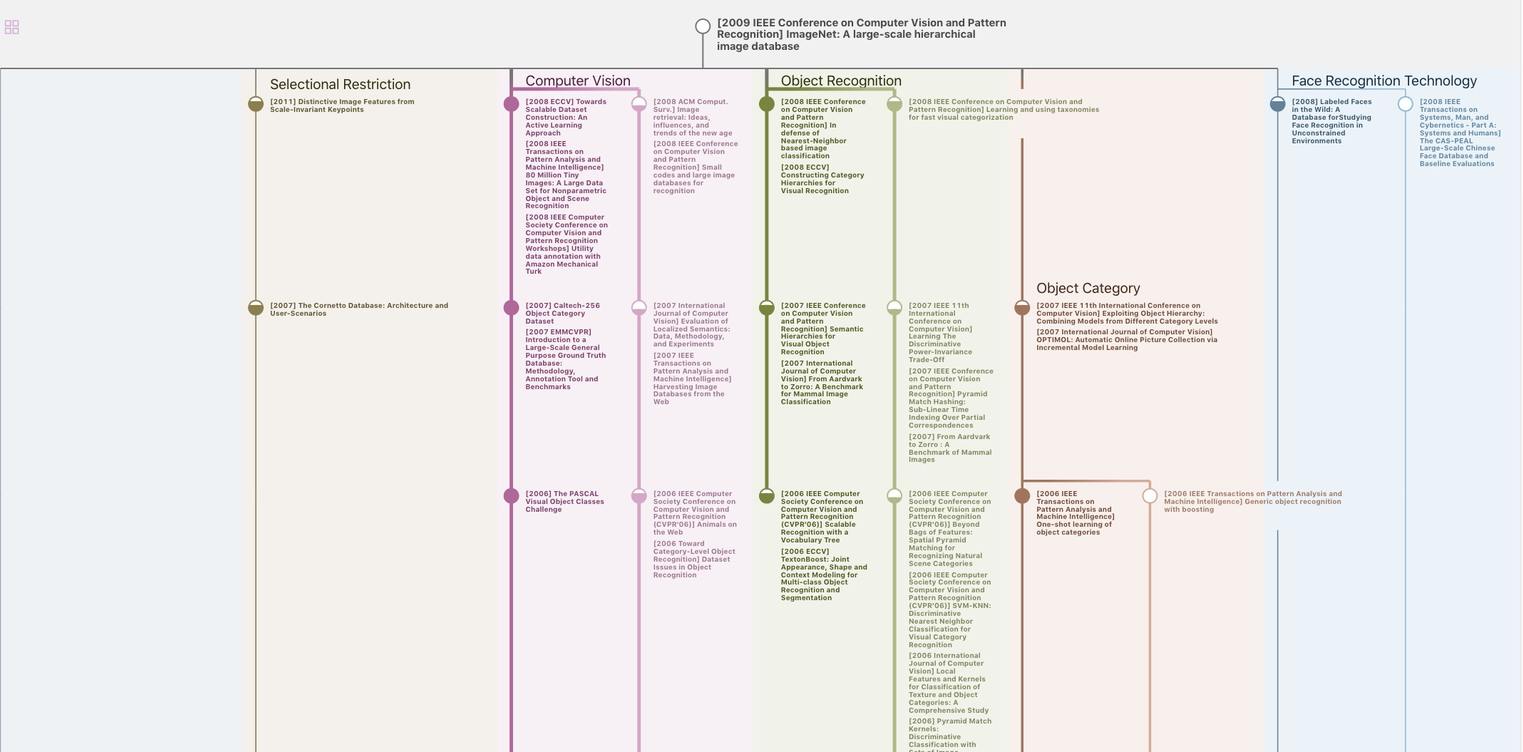
Generate MRT to find the research sequence of this paper
Chat Paper
Summary is being generated by the instructions you defined