Exploring the potentials of artificial intelligence towards carbon neutrality: Technological convergence forecasting through link prediction and community detection
COMPUTERS & INDUSTRIAL ENGINEERING(2024)
Abstract
To explore future opportunities for artificial intelligence (AI) in achieving carbon neutrality goals, this study introduces a forecasting framework that combines link prediction and community detection. The proposed framework begins by utilizing patent information and the International Patent Classification to construct a cooccurrence network. Subsequently, it is computed and validated using two emerging technology datasets: one related to AI towards carbon neutrality and the other to Virtual Reality. A feature selection method and classification model are employed in this process. Finally, predicted convergence links are clustered using network modularity detection to unveil future trends. Through a comprehensive comparison of various similarity indicators in link prediction, this study identifies local indices (Resource Allocation, Adam-Adar) and global indices (Rooted Pagerank, SimRank) as the most featured prediction indicators. These research findings address a gap in the existing literature by providing insights into the rationale behind the selection of link similarity indicators, thereby resolving the issue of randomness in indicator selection. This study also finds that among all single and ensemble models, tree-based models, particularly Random Forest, emerge as the best-performing classifiers. The predicted results suggest that AI technologies towards carbon neutrality can be categorized into two future trends: theoretical methodological foundations and application sectors. This enables the identification and recommendation of future technological potentials on both micro and macro levels. Specifically, it facilitates the discovery of the most promising subdivision technology fields in the future, operating at the microlevel of patent main grouping, often characterized by significant cross-disciplinary links, and at the macrolevel, by visualizing potential technological convergence networks and clustering the trends. The analytical processes and quantitative outcomes of this study can support strategic decision-making for AI, offering valuable insights for both policy-makers and practitioners in their pursuit of green innovation solutions towards carbon neutrality.
MoreTranslated text
Key words
Carbon neutrality,Artificial intelligence,Technological forecasting,Link prediction,Community detection
AI Read Science
Must-Reading Tree
Example
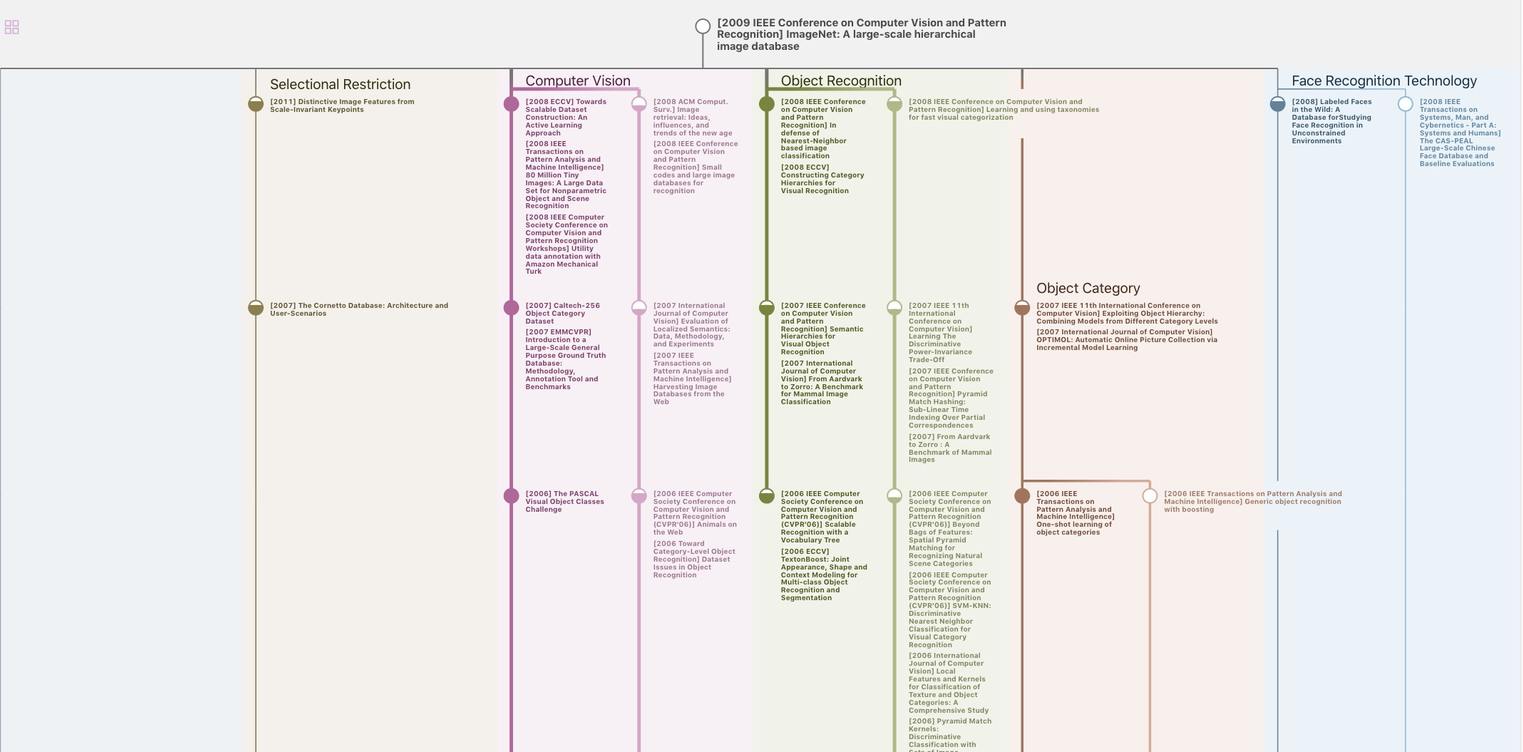
Generate MRT to find the research sequence of this paper
Chat Paper
Summary is being generated by the instructions you defined