A new adaptive feature fusion and selection network for intelligent transportation systems
CONTROL ENGINEERING PRACTICE(2024)
摘要
Drive bearings, as essential components of vehicles, play a crucial role in ensuring the safety and reliability of intelligent transportation systems. By monitoring and analyzing the operating condition of drive bearings, it is possible to troubleshoot the transmission system and improve the service life of the vehicle. However, due to the complexity of monitoring devices, noise interference, and the correlation between data features, traditional feature extraction methods often difficult to obtain satisfactory diagnostic results. To address this issue, this study proposes a novel adaptive feature fusion and selection method for vehicle fault diagnosis systems. First, the CNN-Transformer-LSTM backbone network is used to simultaneously extract local and global features to fully capture the complex patterns and dynamic changes in the data. Secondly, by introducing a self -attention mechanism, the importance of each feature is adaptively adjusted to highlight key information in the complex feature space. Finally, combined with the improved group LASSO technology, redundant features are effectively removed and the accuracy of feature selection is further improved. Extensive experimental results demonstrate that the proposed method outperforms other competing methods in terms of diagnostic accuracy, achieving prediction accuracy up to 99%.
更多查看译文
关键词
Fault diagnosis,Adaptive feature fusion,Feature selection,Compact neural network
AI 理解论文
溯源树
样例
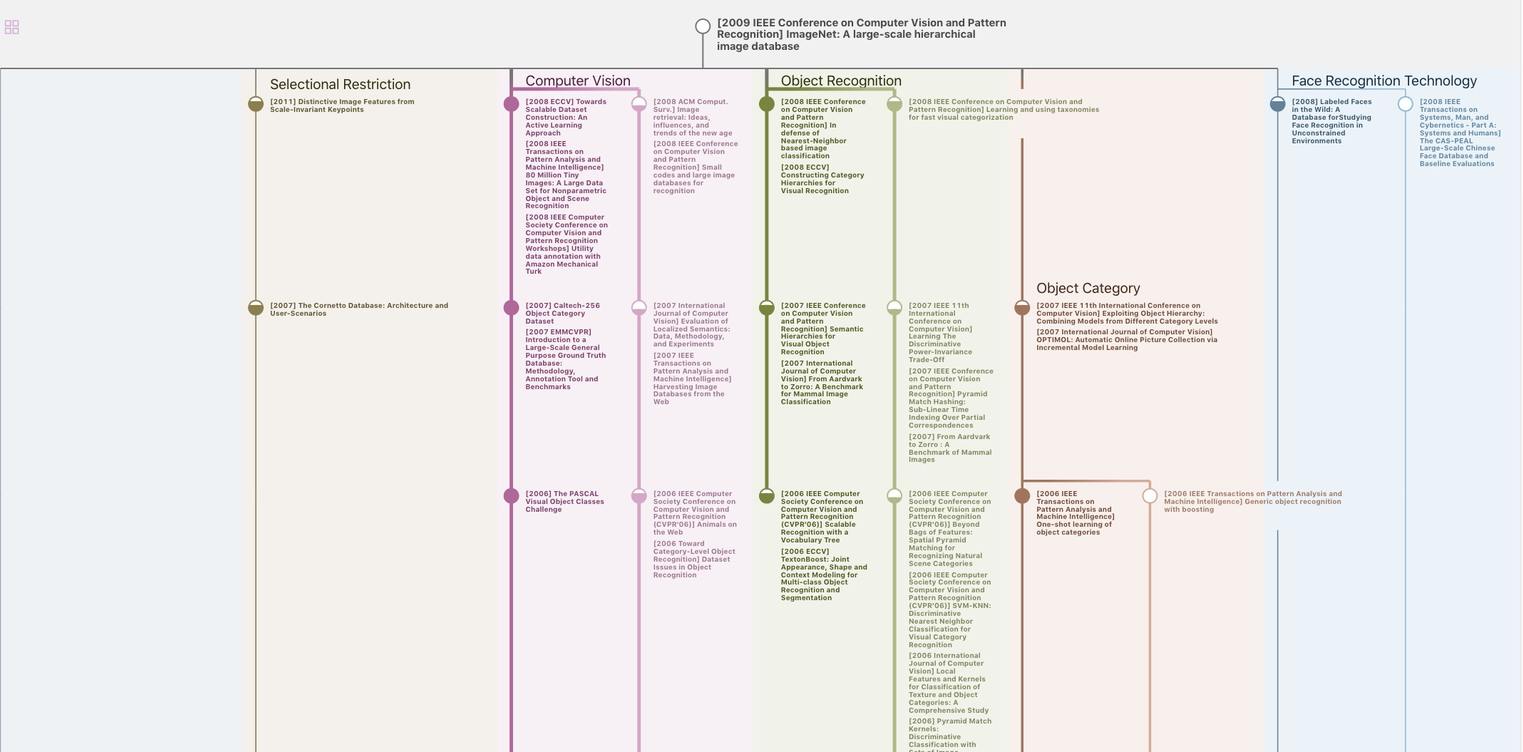
生成溯源树,研究论文发展脉络
Chat Paper
正在生成论文摘要