ICycle-GAN: Improved cycle generative adversarial networks for liver medical image generation
BIOMEDICAL SIGNAL PROCESSING AND CONTROL(2024)
摘要
A high-performance computer -aided diagnosis (CAD) system can enhance the accuracy of liver cancer diagnosis, enabling early detection, diagnosis, and treatment. However, the availability of liver medical image datasets for training CAD systems is limited, and the existing augmentation methods generate images of lower quality, thereby limiting the performance of CAD systems. Therefore, this paper proposes a high -quality liver medical image generation algorithm based on an improved Cycle generative adversarial network (ICycle-GAN). Firstly, a correction network module based on an encoder -decoder structure is introduced into a Cycle generative adversarial network (Cycle-GAN). This module incorporates residual connections to efficiently extract latent feature representations from medical images and optimize them to generate higher -quality images. Secondly, a new loss function is embedded in the network based on the principle of loss correction. This loss function treats blurry images as noisy labels, transforming the unsupervised learning process of medical image transformation into a semi -supervised learning process. Finally, in the comparative experiments, the objective evaluation metrics including structural similarity index measure (SSIM), peak signal-to-noise ratio (PSNR), normalized mean absolute error (NMAE) and Fre ' chet Inception Distance (FID) for the generated liver computer tomography (CT) and magnetic resonance imaging (MRI) images by our proposed algorithm outperform the four mainstream medical image generation algorithms currently available. Moreover, the subjective visual quality of the generated images is also superior to that of the compared methods.
更多查看译文
关键词
Liver image,Computer -aided diagnosis system,Generation of medical image,Cycle-GAN,Correction network
AI 理解论文
溯源树
样例
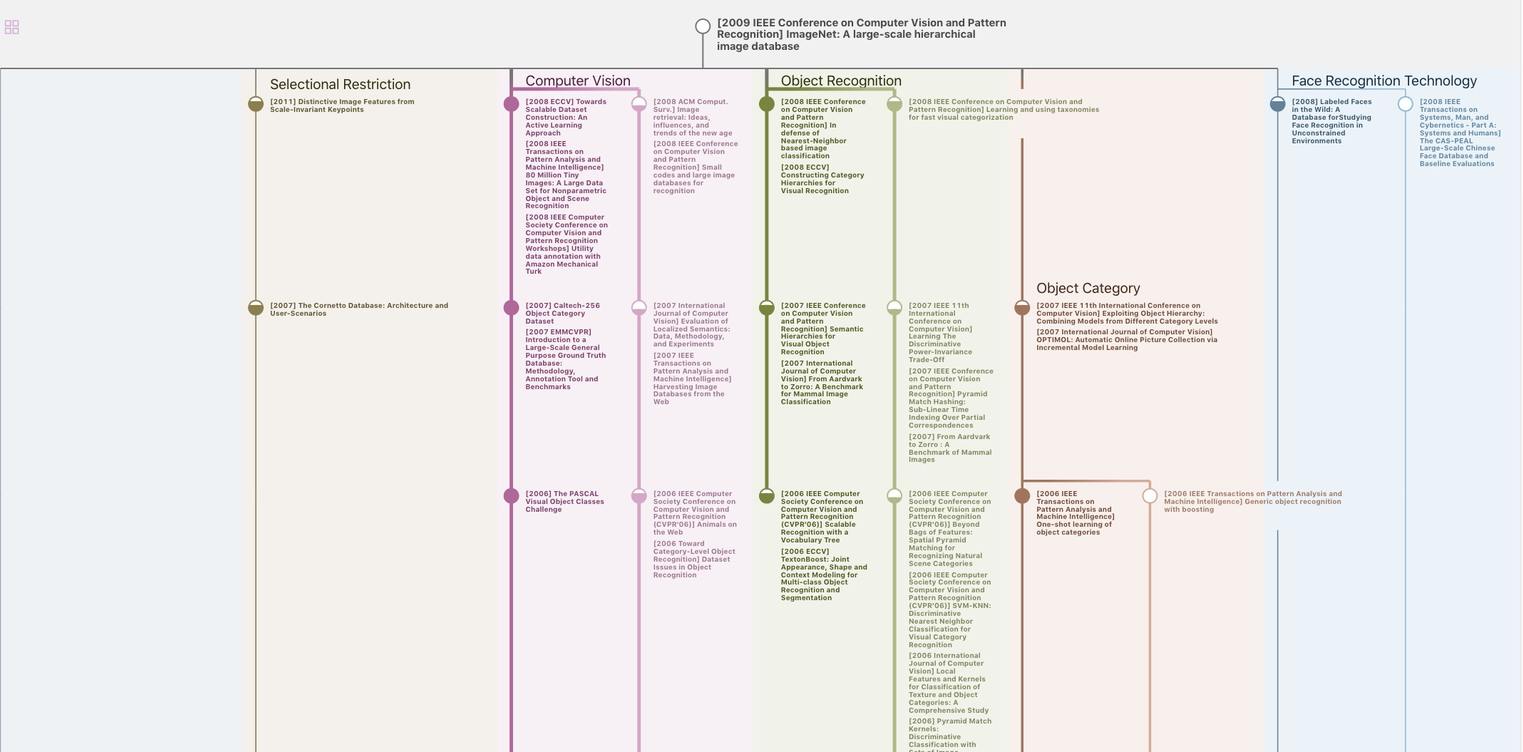
生成溯源树,研究论文发展脉络
Chat Paper
正在生成论文摘要