GDilatedDTA: Graph dilation convolution strategy for drug target binding affinity prediction
BIOMEDICAL SIGNAL PROCESSING AND CONTROL(2024)
摘要
Traditional drug development methods require a huge amount of time. The utilization of deep learning methods for predicting drug-target binding affinity (DTA) has the potential to expedite the drug screening process, thereby accelerating drug development. The key to accurately predicting DTA depends on effectively mining the potential features of drugs and targets. To address this problem, a DTA prediction model (GDilatedDTA) based on graph dilated convolution strategy, which predicts DTA by learning the potential feature representations of drug-target pairs, is proposed in this study. GDilatedDTA includes three steps. First, the feature encoding module acquires the initial features of the drug molecule and the target through integer encoding. Second, the initial encoded features are output to a subsequent deep learning model to mine their potential features. Third, the potential features are combined to predict DTA. A large number of experimental results show that the mean squared error (MSE) of GDilatedDTA on the KIBA and Davis datasets is reduced by 35.71% and 15.57%, on the average, respectively, compared with the state-of-the-art (SOTA) DTA prediction models. Under the drug cold -start setting, the GDilatedDTA's MSE score on the Davis dataset is 26.58% lower than the previous SOTA model, more robust than the baselines, and more effective for unseen drugs. In addition, the visualization of drug-target interaction can be beneficial to predict the binding region of both datasets in the structure -based DTA prediction, thereby improving the interpretability of GDilatedDTA.
更多查看译文
关键词
Deep learning,DTA,Drug repositioning,Graph neural network
AI 理解论文
溯源树
样例
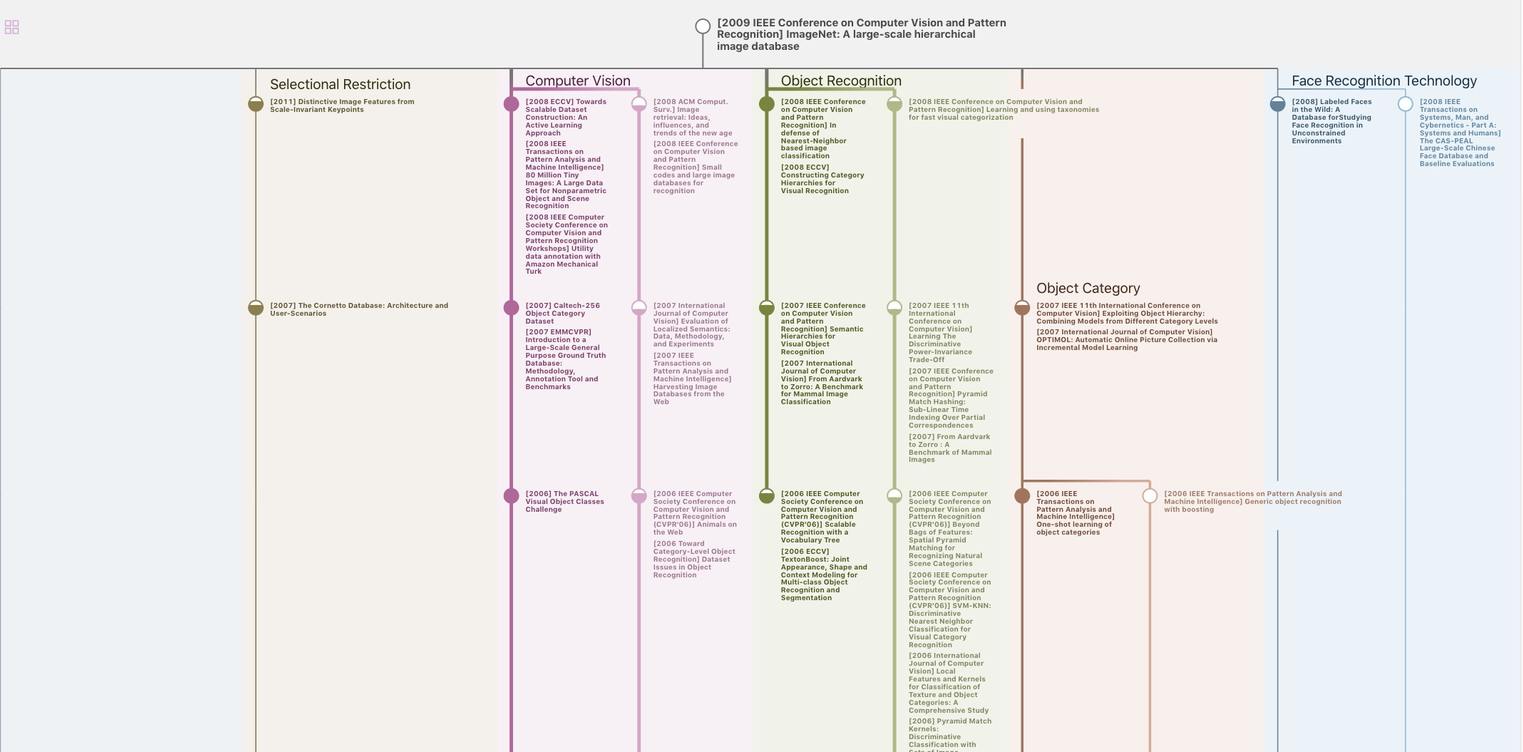
生成溯源树,研究论文发展脉络
Chat Paper
正在生成论文摘要