Multi-stream Bi-GRU network to extract a comprehensive feature set for ECG signal classification
BIOMEDICAL SIGNAL PROCESSING AND CONTROL(2024)
Abstract
Electrocardiogram (ECG) signal analysis plays a crucial role in diagnosing and monitoring various cardiac diseases. Automatic ECG beat classification is necessary to analyze long-term ECG recordings. The major limitations of the traditional automatic ECG beat classification approaches are the constraints of hand-crafted feature extraction, the requirement of an extensive training dataset, dealing with the ECG signal as an image, and poor performance in detecting supraventricular ectopic and ventricular (S and V) beats. To overcome the above -mentioned difficulties, a novel approach to ECG signal classification based on deep feature extraction with minimum complexity along with random forest is proposed in this work. Three different individual blocks are designed with convolutional neural networks (CNN), residuals, and bi-directional gated recurrent units (Bi-GRU) to extract distributed representative, hierarchical & condensed, and long-term dependency features. These extracted features are used to form deep features with the help of concatenation and fusion techniques. The resulting features are able to capture both the morphology and temporal dynamics of the ECG signal. These features are more effective in identifying different types of arrhythmias, predicting future cardiac events, and filtering out noise and artifacts. The unique nature of the features obtained by combining CNN, residual blocks, and Bi-GRU enables a more comprehensive and accurate analysis of the ECG signal, which is particularly important for diagnosing and monitoring cardiac abnormalities. Finally, the extracted deep feature set is utilized to train and test the random forest algorithm. The proposed approach was evaluated on three publicly available datasets and achieved better performance with an overall accuracy of more than 98.00%. Our approach outperforms existing literature by providing a more accurate classification of ECG signals.
MoreTranslated text
Key words
Arrhythmia,Convolutional neural network (CNN),Classification,Deep learning,Electrocardiogram (ECG)
AI Read Science
Must-Reading Tree
Example
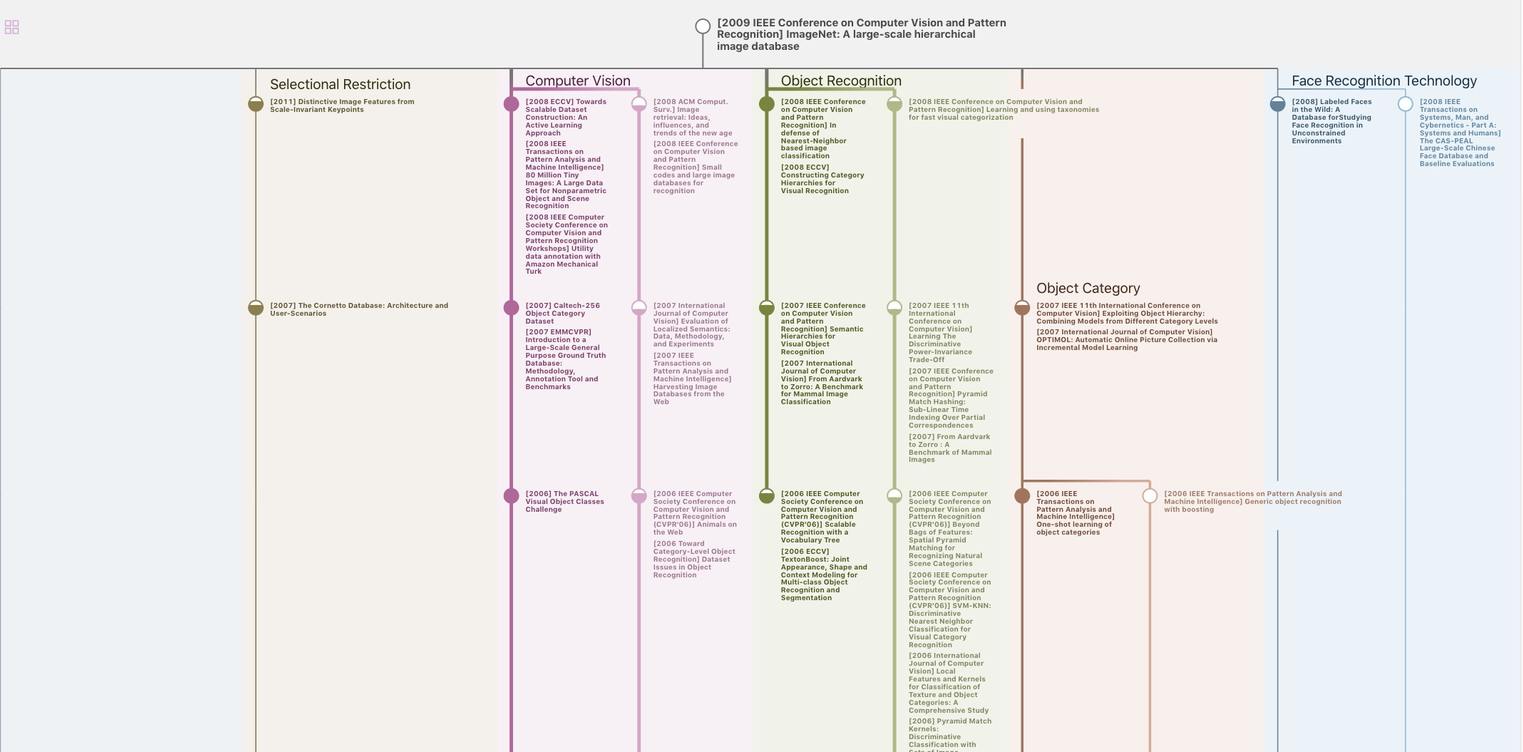
Generate MRT to find the research sequence of this paper
Chat Paper
Summary is being generated by the instructions you defined