Analyzing the impact of regularization on REMSE
Automatica(2024)
摘要
The spectral density of a multidimensional stationary process or a homogeneous random field can be inferred through the REgularized Multidimensional Spectral Estimator (REMSE). The latter is characterized by a convex optimization problem subject to moment constraints, in which we search for a spectral density maximizing the ν-entropy (a generalized definition of entropy) while matching the covariance lags and the ν-cepstral coefficients (a generalized version of cepstral coefficients) of the underlying process. The Lagrange multiplier theory shows that it is only possible to guarantee the existence of an approximate solution provided that in the dual problem we add a regularization term. We investigate the effect of regularization on the REMSE: increasing the power of regularization corresponds to increasing the ν-entropy of the estimated spectrum and, as a consequence, it is expected that the estimated model is more robust in the risk-averse sense.
更多查看译文
关键词
Multidimensional spectral estimation,Covariance and generalized cepstral extension,Regularization,Central path,Entropy-increasing property
AI 理解论文
溯源树
样例
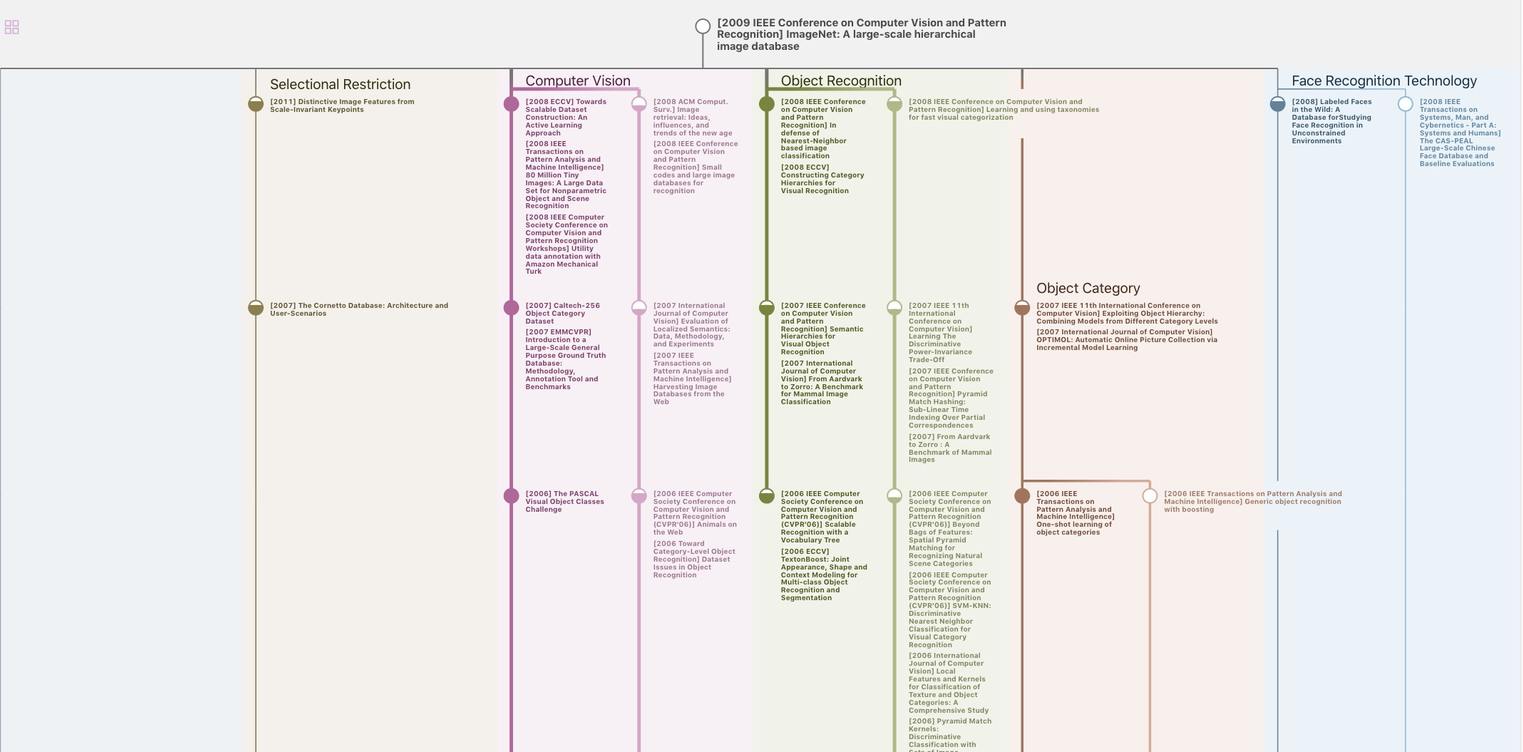
生成溯源树,研究论文发展脉络
Chat Paper
正在生成论文摘要