Optimal differentiated threshold characterization for multi-task stochastic deadline scheduling with queuing
AUTOMATICA(2024)
摘要
We study the dynamic scheduling of multiple deadline constrained tasks in a serving system with N servers and a public queue with M positions. Under stochastic task arrivals and processing costs (with possibly unknown dynamics), we seek to minimize the long-term expected system cost (the sum of task processing cost and penalty cost resulting from missing tasks' deadlines). To mitigate the curse of dimensionality in the action space, we propose a new approach that utilizes optimal policy characterizations to reduce the action space dimensionality without loss of optimality. For a general setting with preemptive/non-preemptive queuing, we establish the optimality of deadline differentiated threshold policies under arbitrary system dynamics. Integrating the established optimal policy characterizations into the proximal policy optimization (PPO) method, the proposed approach is scalable in M+N. Numerical results demonstrate that the proposed approach significantly outperforms the classical PPO method and other priority rule based PPO approaches, in real-world applications including electric vehicle charging and live streaming scheduling. (c) 2024 Elsevier Ltd. All rights reserved.
更多查看译文
关键词
Dynamic programming,Deep reinforcement learning,Queuing,Stochastic deadline scheduling,Electric vehicle charging
AI 理解论文
溯源树
样例
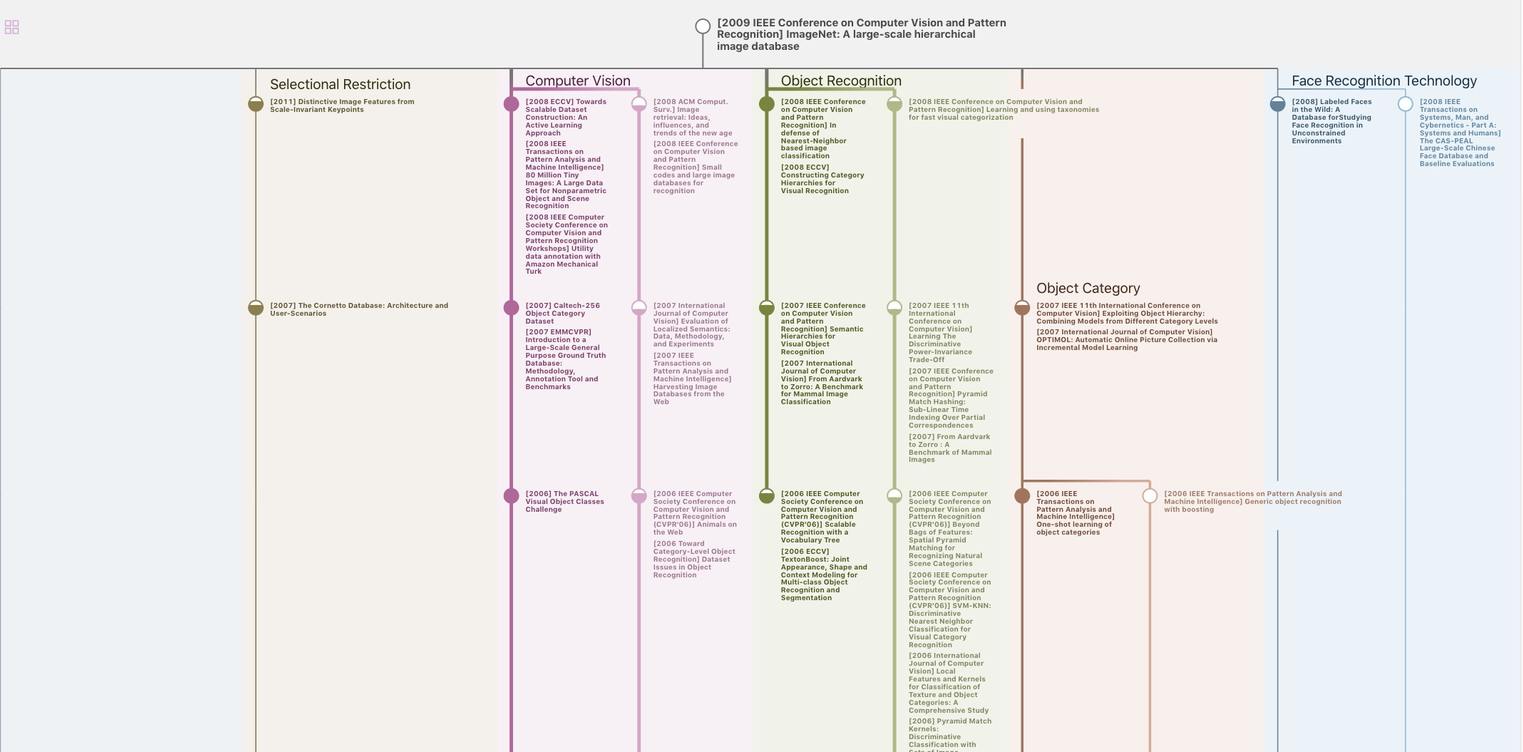
生成溯源树,研究论文发展脉络
Chat Paper
正在生成论文摘要